Harnessing ChatGPT for Molecular Modelling in Laboratory Automation: A Revolutionary Approach
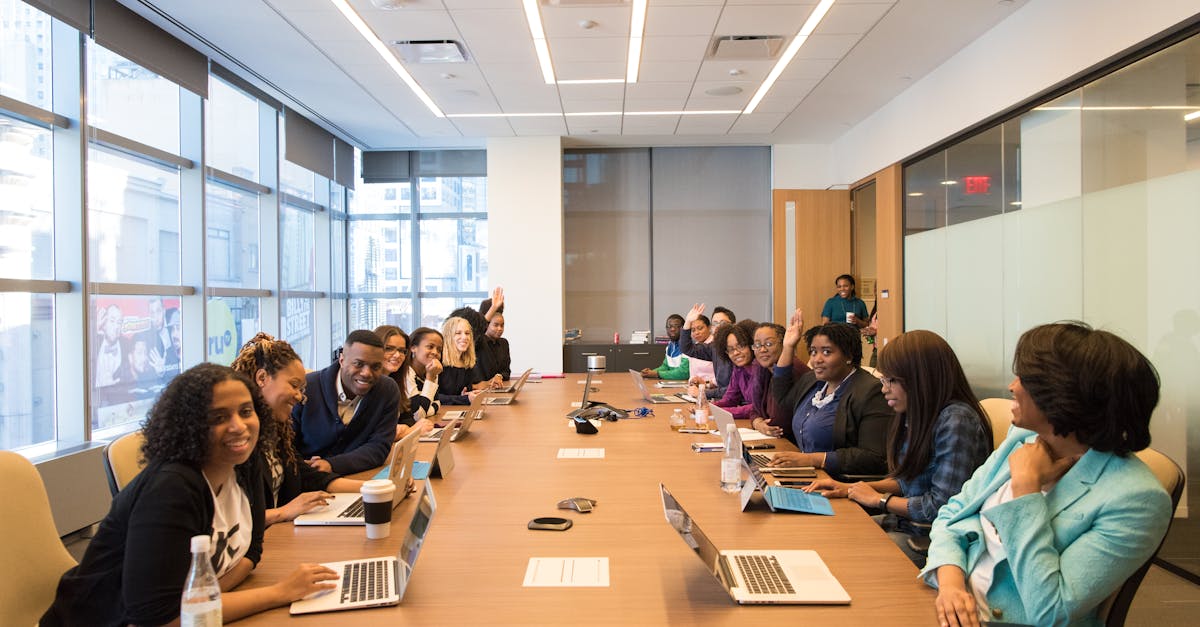
In the field of Molecular Modeling, laboratory automation plays a crucial role in improving efficiency and accuracy in performing experiments. One of the latest advancements in this field is the integration of ChatGPT-4, a powerful language model, to assist scientists in performing and analyzing molecular modeling experiments.
Understanding Laboratory Automation
Laboratory automation refers to the use of technology and equipment to automate various laboratory tasks and processes. These can include sample handling, data analysis, and experiment execution. By automating routine tasks, scientists can save time and focus on more complex aspects of their research.
Molecular Modeling
Molecular modeling is a widely used technique in the field of chemistry and biochemistry. It involves the use of computational methods and models to simulate and analyze the behavior of molecules. This can provide valuable insights into their properties, interactions, and potential applications. Molecular modeling techniques are employed in areas such as drug discovery, material science, and protein folding studies.
The Role of ChatGPT-4
ChatGPT-4, powered by advanced Natural Language Processing (NLP) algorithms, can contribute significantly to laboratory automation in molecular modeling. This cutting-edge technology allows scientists to interact with the language model, receiving assistance in designing experiments, analyzing data, and interpreting results.
Experiment Design
ChatGPT-4 can help researchers in designing molecular modeling experiments. By inputting specific parameters and objectives, scientists can receive suggestions on the most suitable simulation techniques, software tools, and potential limitations to consider. The language model can provide valuable insights based on its extensive knowledge base and ability to understand complex scientific concepts.
Data Analysis and Interpretation
Once the experiments are performed and data is generated, ChatGPT-4 can assist in analyzing and interpreting the results. Researchers can input the relevant data, and the language model can extract meaningful information, detect patterns, and suggest possible explanations or further experiments. This streamlines the data analysis process and provides valuable insights, potentially saving scientists precious time.
Limitations and Precautions
While ChatGPT-4 offers tremendous potential, it is essential to acknowledge its limitations. The language model's suggestions and interpretations are based on the data it has been trained on, and may not always be accurate or suitable for specific experimental setups. Scientists must exercise caution and use their expertise to validate and cross-check the information provided by the model.
Conclusion
Laboratory automation, coupled with advancements in language modeling technology like ChatGPT-4, has transformed the field of molecular modeling. With its ability to assist in experimental design, data analysis, and interpretation, this integration enhances researchers' efficiency and accelerates scientific discoveries. However, it is crucial to balance the use of automation with human expertise to ensure accurate and reliable results in this critical scientific area.
Comments:
Thank you all for reading my article on 'Harnessing ChatGPT for Molecular Modelling in Laboratory Automation: A Revolutionary Approach'. I'm excited to hear your thoughts and answer any questions you may have!
Laslo, I found your article very intriguing. The potential of ChatGPT in molecular modelling is indeed revolutionary. Do you think this approach can significantly accelerate drug discovery processes?
Amy, I'm glad you found it intriguing! Yes, I believe harnessing ChatGPT in molecular modelling has the potential to greatly speed up drug discovery processes. The ability to generate novel molecules and predict their properties could streamline the search for new drug candidates.
Laslo, your article was well-written and informative. I think the use of ChatGPT in laboratory automation can be a game-changer. However, I'm curious about the limitations and potential risks associated with this approach. Could you shed some light on that?
Thank you, William! It's important to consider the limitations and risks. While ChatGPT can assist in generating new molecules, it's essential to validate their physical and chemical properties in real-world experiments. There's also a risk of bias introduced by the training data, which requires careful evaluation. Although promising, caution and experimental validation remain crucial.
Laslo, your article opened my mind to the incredible possibilities of ChatGPT in molecular modelling. It seems like it could revolutionize the field. Do you envision ChatGPT working alongside human scientists, or could it eventually replace them in certain tasks?
Linda, I'm glad you found the possibilities intriguing! While ChatGPT can automate certain tasks and generate hypotheses, I believe it will work best in collaboration with human scientists. Human expertise and judgment play crucial roles in experimental design, data interpretation, and ensuring ethical considerations. ChatGPT can enhance efficiency and offer insights, but I don't foresee it replacing human scientists entirely.
This article fascinated me, Laslo. The integration of ChatGPT in laboratory automation could bring transformative advancements. My concern, though, is the lack of regulation and potential misuse of this technology. Do you think there should be any specific regulations or ethical guidelines?
Samuel, I share your concern. ChatGPT's integration should go hand in hand with thoughtful regulations and ethical guidelines. It's essential to address data privacy, potential biases, and ensure responsible use. Engaging the scientific community, policymakers, and experts in AI ethics will help establish the necessary frameworks to avoid misuse and promote responsible innovation.
Laslo, I really enjoyed reading your article. The application of ChatGPT in molecular modelling holds immense promise. Are there any practical implementations of this approach currently in progress, or is it still primarily theoretical?
Rachel, thank you for your feedback! The application of ChatGPT in molecular modelling is progressing beyond the theoretical realm. Several research groups and companies are actively exploring its practical implementations. While challenges remain, experimental successes and ongoing studies indicate promising real-world applications in drug discovery and materials science.
Laslo, your article provides a fresh perspective on the potential role of AI in laboratory automation. However, I wonder about the computational requirements of ChatGPT. Are there any concerns about scalability and resource limitations?
Robert, scalability and resource limitations are important considerations. ChatGPT does require significant computational resources, especially for training large models. However, as AI technology progresses, there are ongoing efforts to optimize and make it more accessible. Advances in distributed computing and cloud-based solutions can help address these concerns, enabling broader adoption of the technology.
Laslo, I found your article thought-provoking. The potential implications of ChatGPT in laboratory automation are mind-boggling. Are there any potential challenges in integrating this approach into existing laboratory workflows?
Emily, I'm glad you were intrigued! Integrating ChatGPT into existing laboratory workflows can indeed present challenges. Incorporating new technologies often requires adapting workflows, ensuring compatibility with existing tools and processes, and addressing potential resistance to change. Collaborative efforts and open communication between AI developers and laboratory personnel can help overcome these challenges for a successful integration.
Laslo, your article highlights the immense potential of ChatGPT in molecular modelling. However, could you discuss any current limitations in terms of accuracy and reliability?
Mark, accuracy and reliability are indeed crucial. While ChatGPT has shown promising results, it's important to acknowledge its current limitations. Generating accurate molecular structures with desired properties can still be challenging, and the predictions should be experimentally validated. However, ongoing research and model improvements continue to enhance the accuracy and reliability of ChatGPT in molecular modelling tasks.
Laslo, your article paints an exciting future for molecular modelling. Could you highlight any potential applications of ChatGPT beyond drug discovery?
Sophia, beyond drug discovery, ChatGPT holds potential in various applications. It can aid in materials discovery, catalyst design, and optimization of chemical processes. The generative capabilities of ChatGPT can also be applied to protein design and protein-ligand interaction studies. Its versatility offers opportunities across multiple domains within molecular sciences.
Laslo, your article presents an exciting vision for the future of laboratory automation. Do you think ChatGPT could eventually lead to fully autonomous molecular laboratories?
Oliver, it's an intriguing possibility, but fully autonomous molecular laboratories may still be a distant goal. While ChatGPT can automate certain aspects of the laboratory workflow, developing a comprehensive and adaptable autonomous system requires addressing numerous challenges. Safety, reliability, situational adaptability, and human oversight are critical factors that need careful consideration for such a vision to become a reality.
Laslo, your article on ChatGPT in molecular modelling highlights the incredible potential of AI. However, I'm concerned about the accessibility and affordability of such technologies for smaller laboratories or research institutes. What are your thoughts on this?
Isabella, accessibility and affordability are valid concerns. Making advanced AI technologies like ChatGPT more accessible to smaller laboratories and research institutes is crucial for inclusivity. Open-source initiatives, cloud-based platforms, and collaborative efforts can help lower the barriers. It's important to foster an environment where the benefits of AI can be harnessed across different scales and resource levels.
Laslo, your article is fascinating. I can envision ChatGPT as a valuable tool for molecular modelling. However, do you think there's a learning curve for scientists to adapt to this technology, especially those with limited programming skills?
David, adapting to new technologies can certainly involve a learning curve. To ensure wide adoption, it's crucial to develop user-friendly interfaces and tools that abstract away the complexities of programming. Providing intuitive and accessible user experiences can help scientists with limited programming skills leverage the power of ChatGPT effectively, enabling wider participation and democratization of AI in scientific research.
Laslo, your article showcases the transformative potential of ChatGPT in molecular modelling. I wonder about the robustness of the model to handle complex chemical reactions and interactions. Could you shed some light on that?
Mia, handling complex chemical reactions and interactions remains an ongoing challenge. While ChatGPT can generate novel molecules and propose reaction pathways, capturing the full complexity of chemical systems and accurately predicting outcomes in all cases is difficult. Incorporating domain-specific knowledge, adapting training strategies, and refining the models are crucial steps toward improving the robustness in handling complex chemical reactions and interactions.
Laslo, I thoroughly enjoyed reading your article. The integration of ChatGPT in laboratory automation seems promising. In terms of implementation, do you see any specific hurdles to overcome?
Daniel, implementation does come with its share of hurdles. One significant challenge is the integration of ChatGPT with existing laboratory infrastructure, data management systems, and standard protocols. Ensuring interoperability, data security, and compatibility while minimizing disruptions to established workflows are crucial considerations during implementation. Collaboration between AI developers and laboratory personnel can help identify and overcome these hurdles.
Laslo, your article provided a fascinating perspective on the potential of ChatGPT in molecular modelling. I'm curious to know if there are any ongoing collaborations between AI researchers and molecular scientists to accelerate the practical deployment of this technology.
Emma, there are indeed ongoing collaborations between AI researchers and molecular scientists. Many research groups and organizations are working together to bridge the gap between AI advancements and practical deployment in molecular sciences. These collaborations allow for interdisciplinary knowledge-sharing, collective problem-solving, and holistic approaches to accelerate the integration of technologies like ChatGPT into laboratory automation.
Laslo, your article highlights the potential of ChatGPT to revolutionize laboratory automation. However, I'm interested in understanding the role of data quality and quantity in training these models. Could you elaborate on that?
Noah, data quality and quantity are critical factors in training models like ChatGPT. High-quality training data can reduce biases and improve model performance. Sourcing diverse and representative data helps ensure generalizability. However, data quantity alone isn't always sufficient. Strategies like active learning, data augmentation, and continuous model improvement ensure that the models learn effectively from the available data, maximize performance, and adapt to new challenges.
Laslo, your article showcases the immense potential of ChatGPT in molecular modelling. Are there any specific real-world examples or success stories you can share?
Olivia, real-world examples and success stories are emerging in the field of molecular modelling. Researchers have used ChatGPT to propose novel molecules that were subsequently synthesized and shown to possess desired properties. The collaborative efforts of AI and human scientists have led to accelerated materials discovery and drug repurposing. While these successes demonstrate the potential, it's an exciting and active area of ongoing research.
Laslo, I'm amazed by the possibilities ChatGPT presents for molecular modelling. However, what are the computational time requirements for generating novel molecules using this approach?
Jack, the computational time requirements depend on various factors like the complexity of the desired molecules, model size, and available computing resources. Generating novel molecules can be time-consuming, especially for larger and more complex designs. However, advancements in hardware, distributed computing, and algorithmic optimizations can significantly reduce the time required. Efficient implementation and parallelization techniques further aid in mitigating computational time challenges.
Laslo, your article on the use of ChatGPT in molecular modelling is fascinating. Can you discuss any potential biases that might arise during the training and deployment of this technology?
Sophie, biases can indeed arise during the training and deployment of ChatGPT. Biases may be introduced by the training data, which can impact the generated molecules and predictions. Awareness and careful evaluation of the training data, diversity of sources, and continuous monitoring of the models help mitigate the risk of bias. Researchers and developers are actively working on addressing biases to ensure fair and unbiased applications of ChatGPT.
Laslo, your article highlights the potential of ChatGPT in molecular modelling. I'm curious to know if this technology can aid in the study of rare diseases and personalized medicine.
Andrew, ChatGPT can indeed aid in the study of rare diseases and personalized medicine. Its generative capabilities and ability to predict molecular properties can help in identifying potential drug candidates for rare diseases with limited treatment options. Furthermore, ChatGPT can contribute to the design of personalized therapies by assisting in tailoring molecules or identifying molecular interactions specific to individual patients.
Laslo, your article provides an exciting glimpse into the future of molecular modelling. However, what are the computational and resource implications of training and fine-tuning ChatGPT?
Abigail, training and fine-tuning ChatGPT can indeed require significant computational resources. Training large-scale models often demands specialized hardware and access to high-performance computing. However, pre-trained models and transfer learning can help mitigate resource requirements and leverage existing knowledge. Fine-tuning can be done on smaller datasets, reducing the need for massive computational infrastructure while still benefiting from the generative capabilities of ChatGPT.
Laslo, your article highlights the potential of ChatGPT in laboratory automation. Could you discuss any challenges in deploying this technology in domains with strict regulatory standards, such as pharmaceuticals?
Zoe, deploying ChatGPT in domains with strict regulatory standards does present challenges. In highly regulated industries like pharmaceuticals, compliance with safety, quality, and ethical standards is crucial. The explainability and interpretability of AI models, validation requirements, and adherence to established regulatory guidelines are important considerations. Collaborations between AI experts and domain specialists can help navigate these challenges and ensure compliance while harnessing the benefits of ChatGPT.
Laslo, your article provides an insightful perspective on molecular modelling with ChatGPT. Are there any specific areas where researchers are focusing to enhance the performance and capabilities of this technology?
Sophia, researchers are actively working on enhancing the performance and capabilities of ChatGPT in molecular modelling. Improving the accuracy and reliability of generated molecules, expanding the range of molecular properties predicted, and better understanding the underlying mechanisms and limitations are areas of focus. Novel training strategies, integration of domain-specific knowledge, and feedback from experimental validation contribute to the continuous refinement and advancement of ChatGPT in molecular modelling.
Laslo, your article opens up exciting possibilities. Do you think ChatGPT could be used to tackle other scientific challenges beyond molecular modelling?
Grace, absolutely! ChatGPT has the potential to address various scientific challenges beyond molecular modelling. Its generative capabilities and ability to handle complex data make it applicable to tasks like protein folding predictions, materials discovery, and scientific creativity assistance. The flexibility and adaptability of ChatGPT can enable its integration into multiple scientific domains, revolutionizing the way we approach and solve complex research problems.
Thank you all for participating in this discussion on my article. Your questions and insights have been valuable. I hope this article has sparked further interest and exploration into the potential of ChatGPT in molecular modelling. Let's continue to push the boundaries of scientific research and technological innovation!