Improving Medical Diagnostics with ChatGPT: Harnessing the Power of Semantic Web Technology
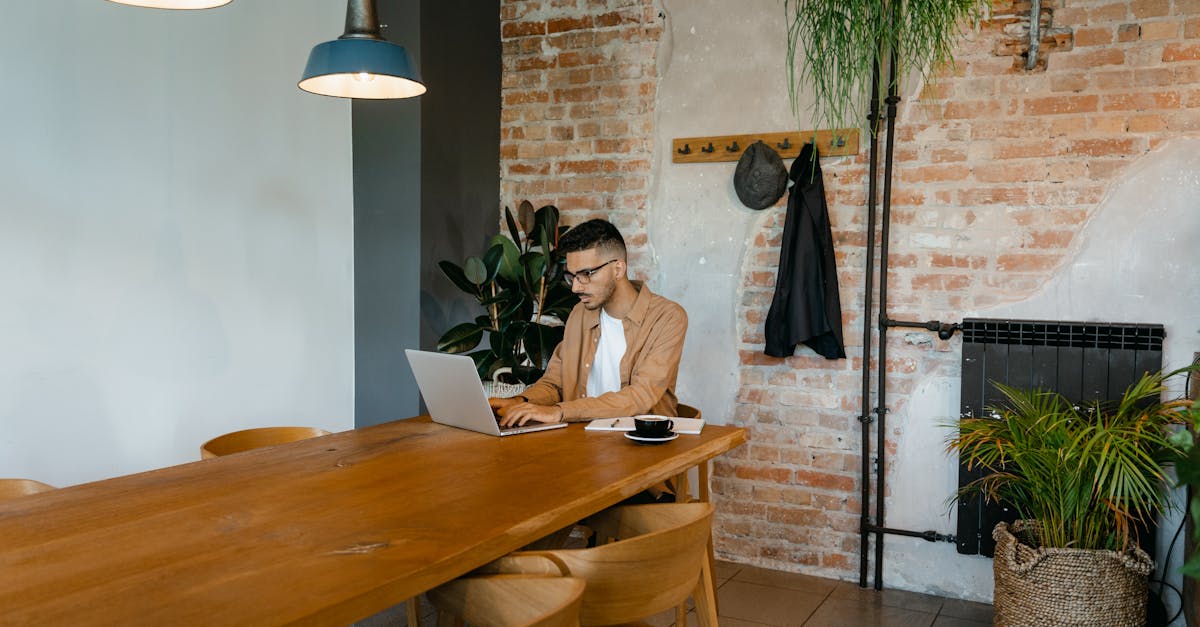
The field of medical diagnostics has always relied on gathering and interpreting data from patients' medical history and symptoms to infer and predict their health conditions. With advancements in technology, the use of semantic web has revolutionized the diagnostic process, enabling healthcare professionals to make more accurate and targeted assessments.
What is Semantic Web?
Semantic web refers to a set of technologies and practices that aim to make the meaning of information on the web understandable to machines, facilitating automated processing and analysis. It allows information to be connected and interpreted in a way that enables inferencing and reasoning.
How Semantic Web is Applied in Medical Diagnostics?
In medical diagnostics, semantic web technologies are utilized to integrate and analyze diverse healthcare data sources, such as electronic health records (EHRs), medical literature, and clinical guidelines. By structuring and linking these sources using ontologies and semantic annotations, healthcare professionals can gain deeper insights into patients' conditions.
Inferencing and Predicting Health Conditions
One of the significant applications of semantic web in medical diagnostics is the ability to infer and predict health conditions based on patients' medical history and symptoms. By leveraging semantic data representations, healthcare professionals can identify patterns and correlations that may not be immediately apparent.
For example, with the help of semantic web technologies, a physician can query a knowledge base with a patient's symptoms and medical history, and infer the likelihood of specific diseases or conditions. The system can analyze the semantic connections between the symptoms, medical history, and known disease patterns, enabling more accurate predictions.
Enhanced Decision Support Systems
Semantic web also plays a crucial role in enhancing decision support systems used by healthcare professionals. These systems rely on capturing and interpreting complex medical data to provide recommendations and guidance for diagnosis and treatment.
Through the use of semantic web technologies, decision support systems can access and analyze vast amounts of medical data, including research papers, clinical guidelines, and patient records. By understanding the context and relationships encoded in the semantic data, these systems can provide more tailored and personalized recommendations for healthcare professionals.
Future Implications
The adoption of semantic web technologies in medical diagnostics has the potential to greatly improve healthcare outcomes. By leveraging the power of inferencing and predicting health conditions, healthcare professionals can make more informed decisions, leading to more accurate diagnoses and personalized treatments.
With ongoing advancements in artificial intelligence and machine learning, the role of semantic web in medical diagnostics is likely to expand further. The integration of medical imaging, genomics, and real-time sensor data into the semantic web framework holds promise for even more precise and timely diagnoses.
Conclusion
The use of semantic web technologies in medical diagnostics brings a new level of connectivity and knowledge integration. By leveraging semantic data from patients' medical history and symptoms, healthcare professionals can enhance their ability to infer and predict health conditions accurately. This not only improves the accuracy of diagnoses but also enables more personalized treatment plans, ultimately leading to better patient outcomes.
Comments:
Thank you for reading my article on improving medical diagnostics with ChatGPT! I'm excited to join this discussion and hear your thoughts.
The potential of integrating semantic web technology with medical diagnostics is immense. It could greatly enhance the accuracy and speed of diagnoses.
I agree, Daniel! The ability to leverage ChatGPT's semantic understanding to interpret medical texts and effectively assist doctors is truly groundbreaking.
However, we should also consider the ethical and legal implications around relying heavily on AI-based diagnostics. Overreliance might lead to negligence or misdiagnosis.
Good point, Amy. While ChatGPT can provide valuable support, it should always be seen as a tool to augment medical professionals' expertise rather than replace them entirely.
I'm curious about the scalability of this approach. How can semantic web technology handle the vast amount of medical data and keep up with new research?
Great question, David! The semantic web technology used in ChatGPT can continuously update its knowledge base by accessing reputable medical databases and staying up-to-date with new research findings.
Another aspect to consider is data privacy and security. How can we ensure that patient data used by ChatGPT remains protected?
Absolutely, Emily. Patient data privacy and security are paramount. Implementing robust encryption, access controls, and strict compliance policies should be a priority when developing and deploying such systems.
On the bright side, ChatGPT can bridge the gap between doctors and patients, making medical information more accessible and understandable for individuals.
Indeed, Marcus. By enabling natural language interactions, ChatGPT can empower patients to ask questions, understand medical jargon, and make informed decisions about their health.
I wonder how well ChatGPT can handle complex and rare medical conditions. Will it be as reliable as human experts in those cases?
That's a valid concern, Sophia. While ChatGPT can analyze vast amounts of medical literature and assist with a wide range of conditions, it's essential to leverage human expertise for complex and rare cases to ensure accuracy.
Do you think the implementation of ChatGPT in medical diagnostics will require specific domain knowledge from the AI model training perspective?
Absolutely, Liam. Fine-tuning ChatGPT with domain-specific medical knowledge is crucial to ensure it understands the intricacies of medical language and maintains high accuracy in diagnosing and suggesting treatments.
What measures should be taken to prevent biases in ChatGPT's predictions and recommendations in medical diagnoses?
A great question, Daniel. It's vital to carefully curate training data and involve diverse medical experts in the model's development to mitigate biases that could lead to unfair treatment or disparities in diagnoses.
I can see ChatGPT being extremely useful in primary care settings, where doctors often encounter a wide variety of conditions. It could assist in initial assessments and help identify potential red flags.
Absolutely, Sarah. ChatGPT's ability to parse and understand patient symptoms can aid primary care physicians in providing more accurate early-stage evaluations and improve patient outcomes.
However, we should be cautious about the potential overreliance on ChatGPT. It's crucial to maintain a balance and encourage doctors to exercise their clinical judgment rather than solely relying on the AI model's suggestions.
Well said, Amy. ChatGPT's purpose is to assist doctors, not replace their expertise. A collaborative approach where doctors and AI work together is the way forward for better healthcare.
How user-friendly would a ChatGPT interface need to be to ensure effective utilization by doctors and patients alike?
Great question, Emily. The interface should be intuitive, user-friendly, and designed in collaboration with medical professionals and patients to meet their specific needs and make interactions efficient and effective.
I can imagine how ChatGPT, with its vast medical knowledge, could potentially assist with accurate differential diagnoses, saving doctors valuable time and minimizing unnecessary tests.
You're absolutely right, David. The ability to generate differential diagnoses based on symptoms and medical history can lead to more efficient and targeted healthcare, benefiting both doctors and patients.
What are the key challenges in implementing ChatGPT in real-world medical settings, and how can we overcome them?
Great question, Marcus. Some key challenges include integrating ChatGPT into existing electronic health record systems, addressing potential liability issues, and ensuring ongoing model updates based on new research. Collaboration between AI developers and healthcare institutions is crucial to overcome these challenges.
ChatGPT's potential for telemedicine could be enormous, especially for remote areas with limited access to specialized healthcare. It could provide immediate assistance and preliminary evaluations.
I couldn't agree more, Sophia. Telemedicine combined with AI-powered medical diagnostics has the potential to bridge gaps in healthcare accessibility, providing prompt assistance where it's needed the most.
Would it be possible to extend ChatGPT's capabilities beyond diagnostics, such as treatment recommendations or staying updated with the latest medical research?
Absolutely, Daniel. ChatGPT's capabilities can be enhanced to provide personalized treatment recommendations, suggest relevant research papers, or even assist in clinical trial searches. The possibilities are vast!
While AI-based diagnostics can be immensely useful in improving healthcare, it's equally important to strike a balance with human interaction to ensure empathetic care and personalized attention.
Well said, Sarah. AI should complement human qualities like empathy, compassion, and the ability to address emotional nuances to create a holistic healthcare experience.
I'm curious about the potential limitations of ChatGPT. What are the situations where it might struggle or provide inaccurate advice?
A valid concern, Amy. ChatGPT might struggle in situations where there is limited information available, ambiguous symptoms, or complex rare conditions. Augmenting it with human expertise can help mitigate these limitations.
Do you envision any regulatory challenges in deploying AI-based diagnostic systems like ChatGPT in healthcare institutions?
Great question, Emily. Regulatory challenges such as obtaining necessary approvals, compliance with data protection laws, and ensuring transparency in AI decision-making may arise. Collaboration between regulators, developers, and healthcare institutions can help navigate these challenges.
The involvement of medical AI in diagnostics certainly looks promising, but we must ensure that AI systems like ChatGPT remain transparent, interpretable, and accountable to build trust among patients and medical professionals.
Well said, David. Transparency and interpretability are essential to gain the trust of both patients and doctors, fostering acceptance and effective utilization of AI systems in medical diagnostics.
Can ChatGPT be tailored to assist specific specialties within the medical field, such as radiology or dermatology?
Absolutely, Marcus. ChatGPT's capabilities can be tailored and specialized to assist various medical specialties, including radiology, dermatology, and more. It can provide valuable insights and aid in accurate diagnoses for specific disciplines.
Considering the rapid progress of AI, do you see a future where AI plays a significant role in medical education and training?
Definitely, Sophia. AI can play a substantial role in medical education by offering intelligent tutoring systems, simulators, and customized learning approaches, ultimately enhancing the training and knowledge retention of future medical professionals.
In many countries, including mine, there's a shortage of doctors and specialists. AI systems like ChatGPT can help alleviate the burden and make healthcare more accessible and efficient for all.
You're absolutely right, Daniel. AI can fill the gaps created by doctor shortages, extend the reach of healthcare to rural or remote areas, and ensure better access to medical expertise for everyone.
I believe that deploying AI systems in healthcare requires a multidisciplinary approach, involving professionals from medicine, computer science, ethics, and law, to ensure responsible and effective use.
Couldn't agree more, Emily. Collaboration between various disciplines is crucial to address the technological, ethical, legal, and societal aspects associated with deploying AI systems in healthcare.
As AI evolves and becomes more capable, it's important to keep the decision-making process transparent. Patients and doctors should understand how AI-derived diagnostics are reached to trust the system.
Absolutely, Amy. Transparency in the decision-making process is key to ensure accountability and build trust between AI systems and the healthcare professionals and patients who rely on them.
The potential of AI-driven medical diagnostics is truly exciting. I'm eager to see how ChatGPT and similar technologies continue to advance and revolutionize healthcare.