Leveraging ChatGPT for Enhanced Financial Health Checks in Consumer Lending
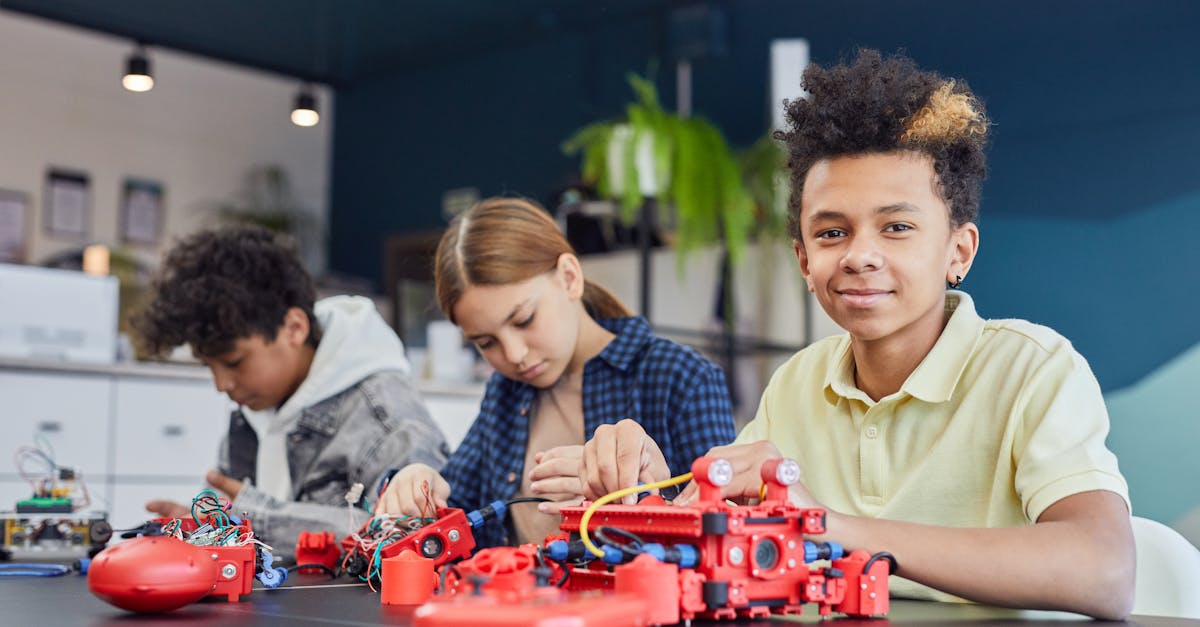
Incorporating artificial intelligence (AI) in the field of financial services is a rapidly emerging trend that is beginning to shape the future of businesses across the globe. A realm where AI can significantly offer innovative solutions is in the area of ‘Consumer Lending’ and ‘Financial Health Checks’. This article discusses how ChatGPT-4, a language prediction model developed by OpenAI, can automate financial health checks enabling customers to gain a comprehensive understanding of their financial standing.
Consumer Lending
Consumer lending is an integral aspect of banking and financial services, providing consumers with the necessary funds for various personal expenses, whether it be for purchasing a home, or funding college tuition. Despite its ubiquity, the process of securing a loan can often be cumbersome and lengthy, involving excessive paperwork and significant time spent in meetings with financial advisors. This is where AI algorithms like ChatGPT-4 can potentially streamline the process.
ChatGPT-4 and Consumer Lending
ChatGPT-4’s potential to revolutionize consumer lending lies in its ability to simplify the lending process. With its capabilities of understanding natural language, it can be used to conduct preliminary conversations with prospective borrowers, gathering information about their income, credit score, and the nature of the loan they are seeking. The algorithm can process such data with remarkable speed and accuracy, reducing the time taken to prepare loan applications and thus accelerating the lending process considerably.
Financial Health Checks
Aside from consumer lending, AI can play a substantial role in conducting financial health checks. A financial health check is a complete assessment of an individual's fiscal health, analyzing multiple aspects such as debts, investments, savings, and financial risks that a person may face. Being acquainted with one's financial standing is crucial for making informed financial decisions.
ChatGPT-4 and Financial Health Checks
With ChatGPT-4, there promises to be a simpler and more efficient way to keep track of your financial health. Through a user-friendly interface, ChatGPT-4 can carry out a comprehensive analysis of your financial situation. This includes, but is not limited to, examining your spending patterns, outlining potential areas of overspending, analyzing your current debts, suggesting ways to pay off debts, and providing recommendations for savings and investment.
Conclusion
The integration of AI in financial services is set to redefine traditional consumer lending and financial health practices, making them more efficient and consumer-centric. As we become more reliant on digital platforms to manage our financial activities, the potential for AI algorithms like ChatGPT-4 to streamline and simplify these processes becomes indispensable. The future of financial health checks and consumer lending indeed looks brighter with such developments, promising enhanced operational efficiency and an overall improved customer experience.
Comments:
Thank you all for taking the time to read and engage with my blog article on leveraging ChatGPT for enhanced financial health checks in consumer lending! I'm excited to dive into the discussion with you.
Great article, Franziska! I think leveraging AI models like ChatGPT can definitely revolutionize the consumer lending process by streamlining financial health checks and improving accuracy. It could save both lenders and borrowers valuable time. What are your thoughts on potential drawbacks or challenges in implementing such a system?
Thank you, Alice! I appreciate your positive feedback. You raise an important point about potential challenges. One major challenge could be ensuring the accuracy and reliability of the AI model's predictions, as financial decisions can have significant consequences for individuals. Adequate training and rigorous testing would be crucial to address this. Additionally, there may be concerns regarding data privacy and security. It would be essential to have strong safeguards in place. What other challenges do you think we should consider?
Another challenge we should consider is the potential for privacy breaches. ChatGPT involves processing personal financial information, which raises concerns about data security. Protecting the borrowers' sensitive information should be a top priority. Strong encryption, strict access controls, and compliance with data protection regulations would be essential. Franziska, what measures could be taken to ensure robust data security while leveraging ChatGPT for financial health checks?
Alice, you bring up a crucial point regarding data security. Robust measures need to be implemented to protect sensitive information. Encryption of data both in transit and at rest, strict access controls, and regular security audits can help safeguard borrower's personal information. Compliance with data protection regulations like GDPR or CCPA would be essential too. Additionally, educating borrowers about the security protocols in place can enhance trust and confidence. Are there any specific security measures you think we should focus on?
Franziska, in addition to encryption and access controls, we should also focus on secure software development practices. Regular vulnerability assessments and penetration testing can help identify and address potential security vulnerabilities. Secure coding standards and practices should be followed during the development of the system. Furthermore, establishing a robust incident response plan in the event of any data breaches or security incidents would be crucial. Transparency around security measures can also enhance borrowers' trust. What are your thoughts on these measures?
I completely agree, Franziska. Secure software development practices should be a priority from the early stages. Incorporating security assessments and audits throughout the development lifecycle, right from the design phase to deployment, can help uncover and address vulnerabilities early on. Additionally, conducting regular staff training and awareness programs can foster a security-conscious culture within the organization. What are your thoughts on involving third-party security experts to assess the system's security measures?
Alice, I completely agree with your point on secure software development practices. In addition to that, regularly assessing and updating the system's security measures based on emerging threats and vulnerabilities is essential. Conducting comprehensive risk assessments and penetration tests can help identify potential weaknesses. Collaborating with cybersecurity professionals and staying up-to-date with industry best practices can ensure robust data security. Franziska, how important do you think it is to have real-time monitoring and incident response capabilities in place?
I agree with Alice that the accuracy and reliability of the AI model should be a top priority. Another concern is the potential for bias in the dataset used to train the model. If the training data is not diverse and representative, it could lead to discriminatory outcomes in lending decisions. To mitigate this, the training data should be carefully curated and continuously monitored. Franziska, how do you think we can ensure fairness and avoid biased outcomes in the lending process?
Excellent point, Bob. Bias in AI models is a critical issue that needs to be addressed in order to ensure fairness. To tackle this challenge, it is important to have diverse and representative training data that includes a wide range of borrower profiles. Additionally, monitoring the model's predictions for any signs of bias or discriminatory outcomes is crucial. Regular audits and transparency in the decision-making process can help in maintaining fairness. Do you have any suggestions on specific techniques or measures we can implement?
Absolutely, Franziska. One approach could be to introduce fairness metrics during the model training phase, such as measuring disparate impact across different demographic groups. By monitoring and minimizing such disparities, we can ensure unbiased lending decisions. Additionally, conducting regular bias audits on the model's predictions and incorporating feedback from an unbiased human review can help fine-tune the system. It's essential to have a combination of rigorous testing and human oversight. What are your thoughts on applying explainable AI methods to address the issue of bias?
Hey everyone! I found this article really insightful. Leveraging ChatGPT for financial health checks sounds promising. But I'm curious about the potential limitations and risks of using such an AI model. Can it handle complex financial scenarios and adjust to changing market conditions? I would love to hear your perspectives!
Hi Carolyn, thank you for joining the discussion! It's a great question. While ChatGPT can be a helpful tool for financial health checks, it's important to note that its performance might have limitations in handling complex financial scenarios. Market conditions, regulations, and individual financial situations can vary significantly, so the model's adaptability to these changes would need to be carefully addressed. Continuous model updates and the integration of real-time data could help alleviate these risks. What other limitations or risks do you think we should consider?
Carolyn, I agree with your concerns. One potential limitation could be the reliance on historical data for making predictions. As we've seen in unprecedented events like the COVID-19 pandemic, traditional models may struggle to adapt to sudden changes in economic conditions. While ChatGPT can learn from the past data, it may need periodic recalibration to ensure it can handle unforeseen scenarios. Ensuring the model's ability to adapt and incorporating expert opinions could mitigate this risk. Franziska, how would you suggest combining the expertise of human reviewers with the AI model's capabilities?
David, you make a valid point. Combining the expertise of human reviewers and the capabilities of the AI model is crucial for effective decision-making. One approach could be to introduce a layered review process where the predictions made by the model are carefully examined by human reviewers with expertise in consumer lending. Their insights and domain knowledge can help validate and refine the model's outcomes. Regular feedback loops between the reviewers and the model can enhance its performance. What other strategies can we employ to combine human expertise with AI capabilities?
I'm concerned about potential algorithmic biases that could arise from training data that may not fully represent the diverse population that takes out loans. If the model is primarily trained on data from a certain demographic, it may not accurately assess the creditworthiness of borrowers from underrepresented communities. Franziska, what steps do you think could be taken to mitigate these biases and ensure fairness in lending even for marginalized communities?
Emily, you raise an important concern. To mitigate algorithmic biases, it would be vital to have a diverse and representative training dataset that includes borrowers from marginalized communities. Careful data collection from a wide range of sources, including different socioeconomic backgrounds, can help address this issue. Regular bias audits and continuous monitoring of the model's performance across different demographic groups can ensure fairness. Additionally, obtaining feedback from borrowers and integrating their experiences into the model's evolution could help mitigate biases. What other steps do you think can be taken to mitigate algorithmic biases?
To mitigate biases, monitoring and auditing the model's performance should go beyond the initial training phase. Ongoing maintenance and updates are essential to address any emerging biases or shifts in the data distribution that affect different communities. Collaborating with domain experts, sociologists, and ethicists can help uncover hidden biases and ensure fair lending practices. Franziska, have you come across any approaches that can help identify and mitigate biases during the ongoing operation of such AI models?
Emily, ongoing monitoring and auditing are indeed crucial to address biases. One approach that can help identify and mitigate biases during system operation is using interpretability techniques such as SHAP (Shapley Additive Explanations). These techniques provide insights into feature importances, allowing for bias analysis and adjustment. Collaborating with external auditors or research organizations for continuous evaluation and monitoring of the AI model's fairness can also contribute to improving its performance. What other measures can we take during the ongoing operation of AI models to mitigate biases?
Franziska, I think interdisciplinary collaborations between experts in finance, machine learning, and consumer protection law would be valuable. By involving legal experts, we can ensure that the decisions made by the AI model comply with applicable regulations and ethical standards. Additionally, setting up a framework for periodic audits and regulatory oversight could provide an extra layer of accountability. What mechanisms do you think can help ensure compliance and ethical decision-making while leveraging ChatGPT in consumer lending?
David, interdisciplinary collaborations are indeed crucial for ensuring compliance and ethical decision-making. Involving legal experts and consumer protection advocates throughout the process can help identify and address any legal or ethical concerns. Regular audits, both internally and by regulatory bodies, can contribute to establishing a compliant framework. Additionally, incorporating explainable AI methods can provide transparency in the decision-making process. What techniques do you think can increase transparency and accountability?
I really enjoyed your article, Franziska! Leveraging AI models like ChatGPT could indeed revolutionize financial health checks. However, I wonder about the potential legal and ethical implications. How do we ensure transparency and accountability when AI algorithms are making lending decisions? What happens if borrowers want to question or appeal against a decision made by the AI model?
I agree with Michael. Transparency and accountability are vital when it comes to AI-driven lending decisions. Borrowers should have the right to understand how decisions are made and have access to explanations if needed. Creating a well-defined and transparent appeals process, where borrowers can question or challenge decisions made by the AI model, would be essential. Franziska, how can we strike the right balance between transparency and protecting proprietary information or model intricacies?
Bob, you've touched upon an important consideration. Balancing transparency and proprietary information can be challenging. While protecting the model's intricacies and proprietary details, it would still be possible to provide explanations of the decision-making process using techniques such as layer-wise relevance propagation or counterfactual explanations. These techniques can help borrowers understand the factors that influenced the decisions made by the AI model without revealing specific details about the model's internals. What are your thoughts on striking this balance?
Franziska, in terms of increasing transparency and accountability in lending decisions, publishing anonymized summaries of the lending decisions made by the AI model, including the factors considered, could be one way to provide insights into the decision-making process. Both borrowers and regulators could have access to these summaries for evaluation purposes, while still maintaining the confidentiality of individual borrower information. What are your thoughts on this approach?
Franziska, finding the right balance between transparency and proprietary information is indeed challenging. I like the idea of providing anonymized summaries of lending decisions to borrowers and regulators. However, it's crucial to ensure that these summaries are easily understandable, avoiding technical jargon or overly complex explanations. Striking the right balance between comprehensibility and level of detail can contribute to fostering transparency and borrower trust. What are your thoughts on involving independent third-party audits to validate the transparency and fairness of the lending decisions?
Bob, involving independent third-party audits is an excellent idea. External audits can provide an unbiased assessment of the system's transparency and fairness. Independent auditors can validate the compliance of the AI model with regulation, ethics, and best practices, thus instilling confidence in both borrowers and regulators. It would also demonstrate a commitment to accountability and openness. Regular audits can help identify any shortcomings or areas for improvement. What are your thoughts on establishing an independent regulatory body to oversee the implementation of AI models in consumer lending?
Hello everyone! As an AI enthusiast, I find this article fascinating. However, I'm curious to know whether ChatGPT could potentially add a human touch to customer interactions. While AI models can be efficient, some borrowers might still prefer speaking with a person directly, especially during complex financial discussions. Franziska, do you think there would be a need for a hybrid approach that combines AI-driven algorithms with human customer support in consumer lending?
Grace, I agree that a hybrid approach could be beneficial. While AI-driven algorithms can significantly streamline the lending process, human customer support can provide personalized assistance and address complex financial discussions where empathy and an understanding of individual circumstances are crucial. A combination of human interaction and AI assistance could offer a more well-rounded customer experience. Franziska, how do you envision the interaction between AI algorithms and human customer support in consumer lending?
David, you raised a valid concern about the limitations of historical data. Continuous recalibration of the model is necessary to ensure it can handle unforeseen scenarios. I believe collaborating with expert economists and financial analysts could help in regularly updating the model's assumptions and inputs based on changing market conditions. Additionally, incorporating real-time economic indicators into the model's features could help it adapt to an evolving economic landscape. What are your thoughts on involving external domain experts to ensure the model's adaptability?
Carolyn, I completely agree. Involving external domain experts would be crucial to ensure the model's adaptability to changing market conditions. Expert economists and financial analysts can provide unique perspectives and insights that the AI model might miss. Collaboration with industry professionals who closely monitor economic trends can help in continuously revising the model's assumptions and inputs. By integrating real-time economic indicators, we can make the model more responsive to dynamic market conditions. What other techniques or data sources do you think would enhance the model's adaptability?
Thank you all for your insightful comments and questions so far! I'm glad we're addressing various aspects of leveraging ChatGPT for enhanced financial health checks in consumer lending. Let's keep the discussion going!
This article got me thinking about potential fraud risks. AI models like ChatGPT need to be resistant to adversarial attacks and attempts to manipulate the system for fraudulent purposes. Ensuring the model's robustness against such attacks would be crucial to maintain the integrity of the lending process. Franziska, do you think the model's performance could be impacted by adversarial attacks, and how could we address this concern?
Oliver, you bring up an important point about fraud risks. Adversarial attacks could impact the model's performance and integrity. Techniques like adversarial training during model development can help improve the model's robustness against such attacks. Additionally, continuously monitoring the system's output for any signs of suspicious behavior or attempted manipulations is crucial. Implementing anomaly detection algorithms and deploying fraud detection mechanisms can help identify and address potential risks. What other strategies or measures do you think would mitigate fraud risks when using ChatGPT for financial health checks?
Hi everyone! I must say, this article provided an interesting perspective on using ChatGPT for financial health checks. However, I'm curious about the feedback loop between the AI model and borrowers. How can the model learn and adapt based on the feedback it receives from borrowers? Franziska, what measures do you think could be implemented to improve the model's performance over time?
Jennifer, the feedback loop between the AI model and borrowers is vital for improving performance. One way to incorporate borrower feedback is to have a post-decision feedback mechanism where borrowers can provide their opinions and perspectives on the lending decision. Supervised fine-tuning of the model based on this feedback can help align the model's predictions with borrowers' needs and expectations. Continuous data collection and learning from borrower interactions would allow the model to adapt and improve over time. What other strategies do you think could enhance the feedback loop?
Franziska, I think integrating user surveys or questionnaires to gather feedback from borrowers could be valuable. These surveys can touch upon their satisfaction levels, perceived fairness of the lending decisions, and areas for improvement. Combining qualitative feedback with quantitative metrics can provide a holistic view of borrower experiences. Additionally, regular sentiment analysis of customer interactions with the model can help identify areas where the model may need adjustments. How would you suggest including borrower feedback during the training process for continual improvement?
Jennifer, including borrower feedback during the training process is important for continual improvement. One approach could be to create a feedback dataset that includes anonymized borrower feedback as part of the training data. By incorporating actual borrower responses in the training, the model can learn from real-world borrower experiences and adapt accordingly. Regular model updates based on this feedback dataset can help improve its performance and ensure that it aligns with borrowers' expectations. What other methods or techniques can be employed to leverage borrower feedback during the training process?
Franziska, for incorporating borrower feedback during the training process, one approach could be to diversify the dataset by including synthetic borrower feedback generated through simulations or controlled experiments. By introducing controlled variations and scenarios, we can explore how the model responds to varying feedback inputs. This iterative approach can fine-tune the model's responses and enhance its ability to generalize to new borrower interactions. Additionally, gathering feedback from real borrowers periodically can help validate the model's performance against evolving borrower expectations. What are your thoughts on incorporating synthetic feedback?
Jennifer, incorporating synthetic borrower feedback through simulations or controlled experiments is a fascinating idea! By generating diverse scenarios and feedback, we can test the model's responses in hypothetical situations that may not be encountered frequently in real-world data. This approach can help ensure the model's robustness and adaptability. However, it's important to strike a balance to avoid overfitting to synthetic scenarios. Regular validation against real borrower feedback and iterative improvements based on their responses remain crucial. What other techniques or methodologies can we employ to validate the model's behavior and responses during the training process?
Hello everyone! As a financial advisor, I find this topic intriguing. Leveraging AI to enhance financial health checks could indeed improve decision-making efficiency. However, I'm curious about the potential impact on employment in the financial industry. How do you think the adoption of ChatGPT and similar AI models could reshape the job landscape in consumer lending? Franziska, I'd love to hear your insights on this aspect.
Emma, your concern about the impact on employment is valid. The adoption of AI models like ChatGPT could lead to a transformation in the job landscape in consumer lending. While some routine tasks related to financial health checks may become automated, there would still be a need for skilled professionals to oversee the AI systems, maintain data privacy and security, and ensure ethical decision-making. Repurposing and upskilling the workforce to focus on tasks that require human judgment, empathy, and expertise could be a way forward. What are your thoughts on retraining and reskilling existing industry professionals to adapt to the changing job landscape?
Hi everyone! I'm intrigued by the possibilities of using ChatGPT for financial health checks. However, I wonder how the model can ensure personalized recommendations and unbiased lending decisions at the same time. Personal financial circumstances can be complex, and tailoring the lending process to individual needs is vital. Franziska, how can we strike the right balance between personalization and avoiding biased recommendations?
Olivia, you bring up an important consideration. Striking the right balance between personalization and avoiding biased recommendations is crucial. One approach could be to train the AI model using data with diverse and representative borrower profiles, ensuring that it captures a wide range of financial circumstances. Additionally, integrating transparency techniques that allow borrowers to understand the factors contributing to the model's recommendations can help ensure fair and unbiased lending decisions. Continuous model evaluation and feedback loops are essential to refine its personalization capabilities and minimize any biases. What other methods do you think can help achieve this balance?
Franziska, I agree with your suggestions on ensuring diverse and representative training data to achieve personalization without biases. Another method could be to incorporate a mechanism for borrowers to provide additional information that might not be captured by the initial dataset. Allowing borrowers to express their specific needs or circumstances through an optional questionnaire or additional input fields can help the model tailor its recommendations more accurately. This way, personalization can be enhanced while minimizing the risk of biased decisions. What are your thoughts on this approach?
Olivia, I appreciate your input! Incorporating a mechanism for borrowers to provide additional information is an excellent idea. By allowing borrowers to share specific needs or circumstances beyond the initial dataset, the model can take into account individual preferences and circumstances, leading to more accurate recommendations. However, proper caution should be exercised to ensure the voluntary provision of information without making it a prerequisite, as mandating extra data might create accessibility challenges. Striking the right balance is crucial to make the process inclusive and personalized. What other measures can we incorporate to enhance personalization while avoiding biases?
Hi everyone! This article sparked an interesting discussion. While leveraging AI models for enhanced financial health checks can bring numerous benefits, we should be cautious about the potential lack of human oversight. Financial decisions carry significant impacts, and solely relying on AI algorithms can lead to potential risks and errors. Franziska, what role do you think the human factor should play in the lending process when AI models are involved?
Sophia, you raise a critical point. The human factor should play an integral role in the lending process, even when AI models are involved. Human oversight is necessary to validate the model's predictions, identify potential biases or errors, and ensure fairness and ethical decision-making. Collaborating with human reviewers who possess expertise in consumer lending can provide the necessary checks and balances. A hybrid approach that combines AI algorithms with human judgment can lead to more reliable and responsible lending practices. What other roles or responsibilities do you see for humans in AI-driven lending processes?
Franziska, I completely agree. Incorporating human judgment and expertise is vital for mitigating risks, ensuring fair lending, and maintaining ethical standards. Apart from human reviewers, it would also be valuable to involve customer-facing personnel who can interact directly with borrowers, providing explanations, clarifications, or assistance when needed. These professionals can act as a bridge between borrowers and AI algorithms, instilling trust and enhancing the overall customer experience. Additionally, their feedback and insights can help uncover areas for improvement in the system. What are your thoughts on this approach?
Sophia, involving customer-facing personnel who can provide support, explanations, and assistance is an excellent suggestion. They can help address complex borrower concerns, provide a human touch to interactions, and instill confidence in the lending process. These professionals can act as trusted advisors, guiding borrowers through the process and ensuring that their individual needs are met. Their direct feedback and observations can be valuable in identifying potential issues or improvements in the AI system as well. What other roles or responsibilities do you think customer-facing personnel could have in an AI-driven lending process?