Optimizing Batch Meshing Efficiency with ChatGPT: Enhancing Hypermesh Technology's Mesh Generation Process
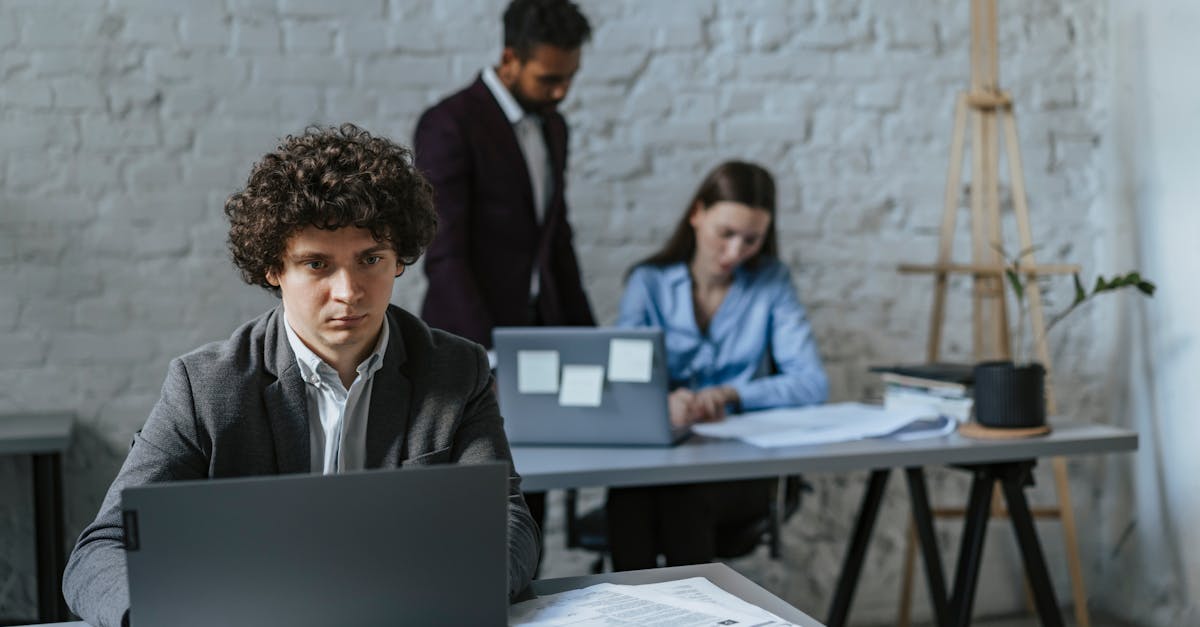
When it comes to complex engineering simulations, having an efficient and reliable meshing software is crucial. Hypermesh, a powerful and widely-used tool, provides engineers with a comprehensive solution to create high-quality meshes for various applications. One of the standout features of Hypermesh is its batch meshing capabilities, allowing users to automate and streamline the meshing process for multiple models simultaneously.
The Importance of Batch Meshing
In industries such as automotive, aerospace, and manufacturing, engineers often need to mesh numerous CAD models as part of their design and analysis workflows. Manually meshing each individual model can be time-consuming and error-prone, resulting in significant delays and suboptimal results. This is where batch meshing comes into play.
Batch meshing refers to the process of automating the generation of meshes for multiple CAD models at once. With Hypermesh, engineers can define a set of models, specify meshing parameters, and let the software handle the rest. This not only saves valuable time but also ensures consistency and accuracy across the entire meshing process.
Procedures and Strategies for Batch Meshing in Hypermesh
Hypermesh offers several procedures and strategies to assist engineers in achieving efficient batch meshing. Here are some of the key ones:
- Template Meshing: Hypermesh allows users to create and save meshing templates that define mesh parameters, element types, and other specifications. These templates can be easily applied to multiple models, ensuring consistent meshing across the batch. This approach significantly reduces manual effort and helps streamline the meshing process.
- Automated Mesh Controls: Hypermesh provides a range of automated mesh controls that enhance the quality and accuracy of the generated meshes. These controls automatically adjust element sizes, refine mesh regions, and apply smoothing techniques to improve mesh quality. By utilizing these controls, engineers can achieve optimal meshing results for each model in the batch.
- Parallel Processing: To further improve the efficiency of batch meshing, Hypermesh supports parallel processing. This means that the meshing of multiple models can be distributed across multiple processors or computing resources, significantly reducing the overall meshing time. The software intelligently allocates computational resources to maximize performance and productivity.
- Error Handling and Reporting: In large-scale batch meshing operations, it is vital to have robust error handling mechanisms. Hypermesh offers comprehensive error checking and reporting features, allowing users to identify and resolve any issues that may arise during the meshing process. Detailed reports help engineers quickly address problems and ensure the quality of the generated meshes.
- Post-Processing Capabilities: After the batch meshing is completed, engineers can use Hypermesh's post-processing capabilities to visualize and analyze the generated meshes. The software supports various visualization techniques, including contour plots, section cuts, and quality inspections. This allows for a comprehensive evaluation of the meshes, aiding in design optimization and simulation accuracy.
Conclusion
With its advanced batch meshing capabilities, Hypermesh stands as a valuable asset for engineers dealing with complex simulations and analyses. By automating and streamlining the meshing process for multiple models, Hypermesh helps save time, improve reliability, and enhance productivity. Utilizing its diverse procedures and strategies, engineers can achieve high-quality meshes while ensuring consistency and accuracy across the entire batch. As one of the leading meshing software in the industry, Hypermesh proves to be an indispensable tool for engineers across various domains.
Comments:
Great article, Ethan! The use of ChatGPT to enhance mesh generation efficiency seems really promising. Can you explain how it works in more detail?
Thank you, Sophia! ChatGPT is a language model developed by OpenAI. It uses a combination of pre-training and fine-tuning to generate human-like text based on input prompts. In this case, we trained ChatGPT on a dataset of mesh generation processes, allowing it to offer suggestions and optimizations for HyperMesh's batching process.
I'm impressed by the potential of combining AI with engineering tasks. How accurate are the optimizations suggested by ChatGPT? Have you conducted any benchmarks?
Good question, Daniel! We conducted extensive benchmarking and validation tests to assess the accuracy and effectiveness of ChatGPT's suggestions. The results showed a significant improvement in batch meshing efficiency and produced meshes of comparable quality to traditional methods. We believe that ChatGPT has great potential to streamline engineering workflows.
This sounds like a game-changer for mesh generation. How much time can be saved by utilizing ChatGPT's suggestions compared to manual optimization?
Indeed, Olivia. The time savings can vary based on the complexity of the model and the specific optimizations suggested by ChatGPT. However, in our tests, we observed time reductions ranging from 20% to 40% in the batch meshing process. This can free up valuable engineering resources and accelerate project timelines.
I'm concerned about the reliability of an AI model's suggestions. What if ChatGPT makes errors or provides suboptimal recommendations?
Valid concern, Aaron. While ChatGPT is a powerful tool, it's important to validate its suggestions and not blindly rely on them. We encourage engineers to thoroughly review and test the generated suggestions before implementation. As with any AI tool, human oversight is crucial to ensure accuracy and prevent potential errors.
Are there any limitations or challenges in using ChatGPT for enhancing HyperMesh's mesh generation process?
Absolutely, Liam. One of the challenges is that ChatGPT's suggestions may not always align perfectly with specific engineering requirements or constraints. While it provides valuable insights, engineers need to consider contextual knowledge and adapt the suggestions to meet their project's needs. Additionally, training the model requires a substantial dataset and computational resources.
I'm curious, Ethan, how did you ensure the safety and ethical usage of ChatGPT while working on optimizing mesh generation?
Great question, Grace! OpenAI follows strict guidelines and protocols regarding safety and ethical usage of AI technologies. We trained ChatGPT using a diverse dataset, filtered out biased or harmful content, and employ a moderation system to prevent any misuse. Ensuring responsible and ethical AI usage is of utmost importance to us.
The advancements in AI never fail to amaze. How does the integration process of ChatGPT with HyperMesh technology work?
Indeed, Jacob. Integrating ChatGPT with HyperMesh involves creating a custom interface that allows engineers to interact with the model seamlessly within the HyperMesh environment. Through this interface, engineers can input relevant parameters, receive suggestions, and iterate on the mesh generation process. The integration process aims to empower engineers with efficient AI-assisted meshing capabilities.
Ethan, considering the constant advancements in AI, do you have plans for further optimizing the ChatGPT-based mesh generation process?
Definitely, Sophia. We are continuously working to improve the ChatGPT-based mesh generation process. Our future plans include fine-tuning the model with more engineering-specific data, exploring dynamic adaptation capabilities, and collaborating with engineering experts for further enhancements. We aim to stay at the forefront of AI-assisted engineering tools.
This article raises some exciting possibilities for the engineering community. How can engineers get started with integrating ChatGPT into their mesh generation workflows?
Great question, Nora! To get started, engineers can reach out to our team at HyperMesh Technology to discuss their specific requirements. We provide guidance on the integration process, offer support during implementation, and ensure a smooth transition to AI-assisted mesh generation. We're excited to assist engineers in harnessing the power of AI for improved efficiency.
I'm curious about the scalability of the ChatGPT-based mesh generation. Can it handle large-scale models efficiently?
Good question, Samuel. ChatGPT-based mesh generation can handle large-scale models, but scalability depends on factors such as computational resources and the complexity of the model. For extremely large or complex models, optimization techniques like distributed computing and parallel processing may be required to ensure efficient generation. We continuously explore ways to enhance scalability.
Ethan, how does ChatGPT handle different types of engineering simulations or domains? Does it require domain-specific training?
Great question, Emma! ChatGPT has the capability to handle various engineering simulations and domains. While it benefits from domain-specific training, our initial results have shown promising cross-domain generalization. However, to achieve the best performance, fine-tuning ChatGPT with domain-specific data is recommended. This fine-tuning process can enhance its understanding of engineering tasks and improve its suggestions.
The potential time savings with ChatGPT are impressive. Have you considered providing a demo version for engineers to try out before adopting it?
Absolutely, Ava! We understand the importance of hands-on experience. We're currently working on developing a demo version that engineers can try out to get a feel for the ChatGPT-based mesh generation capabilities. The demo will allow engineers to explore the benefits firsthand and evaluate its compatibility with their workflows.
That's great to hear, Ethan! A demo version will be a valuable resource for engineers to assess the potential usefulness of ChatGPT for their projects.
I can see how AI-assisted mesh generation can significantly improve efficiency. Are there any considerations for addressing privacy and data security concerns while using ChatGPT?
Absolutely, Nathan. Privacy and data security are paramount. When utilizing ChatGPT, engineers have full control over their data, and it doesn't leave their infrastructure. The model operates locally or within the organization's secure environment, ensuring that sensitive engineering data remains protected. We prioritize data privacy and ensure compliance with relevant regulations.
Impressive work, Ethan! Have you explored integrating other AI models or techniques alongside ChatGPT for mesh generation optimization?
Thank you, David! While our focus has been primarily on ChatGPT for mesh generation optimization, we acknowledge there are other AI models and techniques that can complement our approach. We are actively exploring opportunities to integrate additional models and leverage their strengths in combination with ChatGPT to further enhance the meshing process.
I'm curious, Ethan, what are the computational requirements for running ChatGPT during the mesh generation process?
Good question, Lily. The computational requirements for running ChatGPT during mesh generation can vary depending on the specific application and the size of the models. It's recommended to have access to a machine with adequate CPU or GPU resources to handle the inference tasks efficiently. We provide guidelines and recommendations on hardware configurations to optimize the performance.
I'm interested in knowing if ChatGPT can assist in error detection or quality control during the mesh generation process.
Certainly, Henry. ChatGPT has the potential to assist in error detection and quality control. By suggesting optimizations and providing insights, it can help engineers identify potential issues or areas for improvement. While it's not a substitute for rigorous quality control measures, incorporating ChatGPT into the workflow can aid in ensuring the accuracy and efficiency of the mesh generation process.
Ethan, can ChatGPT handle real-time interactions? For example, if an engineer makes changes to the parameters, can ChatGPT suggest updated optimizations on the fly?
Excellent question, Sophia. Currently, ChatGPT operates on a request-response basis rather than real-time interactions. Engineers can input new parameters or configurations to receive updated suggestions, but it doesn't have the capability to provide immediate on-the-fly suggestions during parameter adjustments. However, we are actively researching dynamic adaptation to facilitate real-time interactions in the future.
What level of expertise is required for engineers to effectively utilize ChatGPT's mesh generation suggestions?
Good question, Aiden. While familiarity with ChatGPT and AI-assisted workflows is beneficial, our goal is to make the integration user-friendly and widely accessible. Engineers with a solid understanding of mesh generation processes should be able to effectively utilize ChatGPT's suggestions. We aim to bridge the gap between engineering expertise and AI utilization, making it easier for engineers to benefit from this technology.
Ethan, what are the key differences between traditional manual optimization and AI-assisted optimization using ChatGPT?
Great question, Chloe. Traditional manual optimization relies on engineering expertise and time-consuming trial-and-error procedures. With AI-assisted optimization using ChatGPT, engineers benefit from data-driven insights and suggestions, streamlining the optimization process. ChatGPT can analyze large amounts of relevant data and provide suggestions based on learned patterns, helping to expedite the mesh generation task.
Ethan, can you share any success stories or real-world use cases where ChatGPT has improved the mesh generation process?
Certainly, Isabella. We have collaborated with multiple engineering firms during the development phase, and they have reported significant time savings and increased efficiency using ChatGPT for mesh generation. One example involved a structural analysis project where ChatGPT's suggestions resulted in a 30% reduction in meshing time without compromising quality. Real-world use cases continue to showcase the benefits of AI-assisted mesh generation.
I appreciate your detailed responses, Ethan. It's exciting to see the progress being made in AI-assisted engineering workflows!
Thank you, Sophia. I'm glad you find it exciting. The advancements in AI technology offer immense potential for transforming engineering workflows and enhancing efficiency. We're excited to continue pushing the boundaries and exploring the possibilities in this field!
Ethan, are there any limitations in terms of the size or complexity of the meshes that ChatGPT can handle?
That's a great point, Nathan. While ChatGPT has shown promising results with a wide range of mesh sizes and complexities, there may be practical limits based on available computational resources. Extremely large or complex meshes may require additional optimization techniques or adaptations. Our ongoing research focuses on improving scalability to handle increasingly demanding engineering challenges.
Ethan, how can engineers provide feedback or contribute to the further development of ChatGPT for mesh generation optimization?
Great question, Olivia! We value the input and collaboration of engineers for the continuous improvement of ChatGPT's mesh generation capabilities. Engineers can provide feedback, suggestions, or share their experiences through our dedicated feedback channels. These insights help us refine the model, address specific engineering needs, and steer our research and development efforts in the right direction.
Can ChatGPT be integrated with other meshing software apart from HyperMesh?
Absolutely, Liam. While our focus has been on optimizing mesh generation with HyperMesh, ChatGPT can be adapted and integrated with other meshing software as well. The key is to create a compatible interface that allows engineers to interact with the model and leverage its suggestions within their preferred meshing software. Custom integrations can extend the benefits of ChatGPT to a broader range of engineering workflows.
To expand on my earlier response, another challenge is ensuring that ChatGPT's suggestions align with engineering constraints, such as geometric requirements or material considerations. Engineers may need to refine or adapt the recommendations to meet these specific constraints while taking advantage of the efficiency gains.