Predicting Adverse Reactions: Harnessing the Power of ChatGPT in Pharmaceuticals
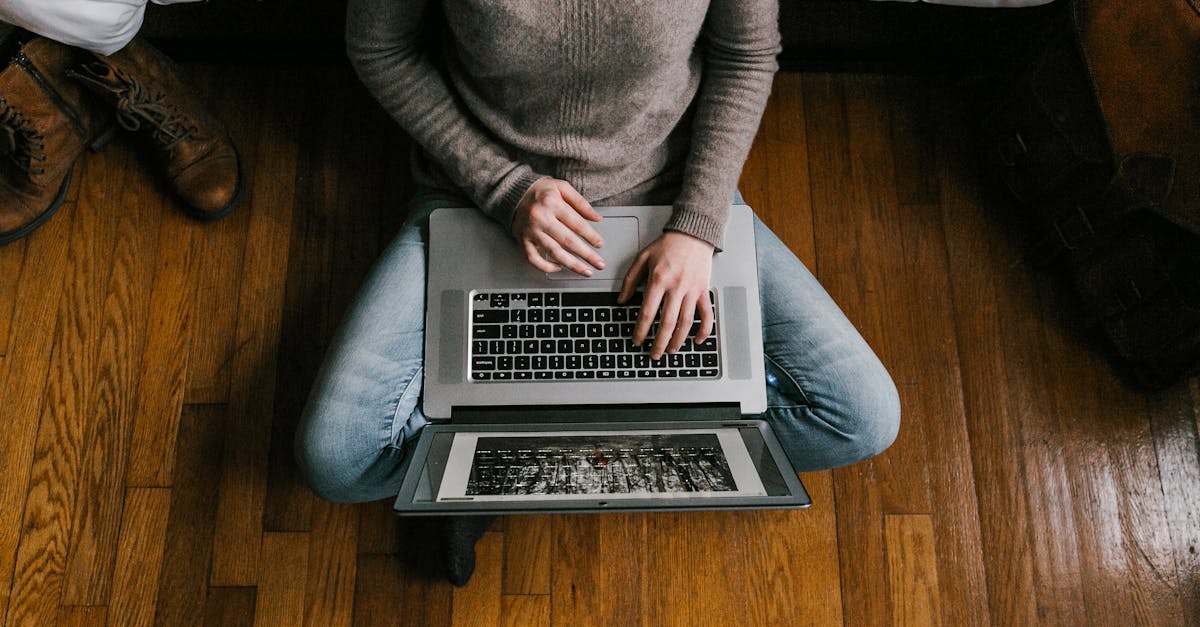
Pharmaceuticals play a crucial role in the healthcare industry, providing treatments and lifesaving medications to millions of patients worldwide. However, like any medical intervention, medications can have unintended effects, known as adverse drug reactions (ADRs). These reactions can range from mild discomfort to life-threatening conditions, making it essential for pharmaceutical companies and healthcare professionals to identify and predict potential ADRs.
Advancements in technology, specifically machine learning, have opened up new possibilities for predicting and preventing ADRs. Machine learning models can analyze vast amounts of medical data, including patient demographics, medical history, and drug usage, to identify patterns and associations between certain medications and adverse reactions.
One of the primary uses of machine learning in pharmaceuticals is the development of predictive models that can assess the likelihood of a patient experiencing an adverse reaction to a particular drug. These models employ various algorithms, such as decision trees, random forests, and neural networks, to analyze and classify medical data.
The process of building these predictive models involves training the algorithm on a dataset containing historical medical records, adverse reactions, and drug information. The model then learns to recognize patterns and associations between specific drugs and adverse reactions by analyzing the data. Once the model is trained, it can be used to predict the likelihood of an adverse reaction for a new patient based on their unique characteristics and medication history.
Machine learning models can significantly improve the accuracy and efficiency of ADR prediction compared to traditional methods. They can utilize a much larger dataset, encompassing diverse patient populations and drug histories, leading to more comprehensive and reliable predictions. Additionally, these models can adapt and update themselves as new data becomes available, improving their accuracy over time.
Pharmaceutical companies can benefit from ADR prediction models by using them during the drug development process. By identifying potential adverse reactions early on, pharmaceutical companies can make informed decisions about drug safety and efficacy. This can help prevent costly drug recalls and ensure patient safety.
In healthcare settings, machine learning models for ADR prediction can assist healthcare professionals in personalized patient care. These models can provide additional insights into a patient's risk profile, allowing healthcare professionals to tailor their treatment plans and minimize the potential for adverse reactions.
Despite the many advantages of machine learning in adverse reaction prediction, there are challenges that need to be addressed. The quality and completeness of the medical data being used, data privacy concerns, and the interpretability of the machine learning models are all important considerations in deploying these models for practical use.
In conclusion, machine learning models have emerged as a powerful tool in predicting adverse drug reactions in the pharmaceutical industry. These models can analyze large amounts of medical data to identify patterns and associations, enabling accurate prediction of potential adverse reactions. By leveraging these models, pharmaceutical companies and healthcare professionals can make informed decisions, improve patient safety, and enhance personalized care.
Comments:
Thank you all for taking the time to read my article! I'm excited to discuss the use of ChatGPT in the pharmaceutical industry for predicting adverse reactions. Let's get started!
As a pharmacist, I find this application of ChatGPT fascinating. It has the potential to greatly enhance our ability to identify and prevent adverse reactions. However, I wonder how accurate the predictions are based on GPT's language model.
Jessica, I'm sure you have valid concerns, but it's important to note that ChatGPT can learn from vast amounts of medical literature and analyze patterns that humans might miss. It may not be perfect, but it can certainly serve as an additional tool for pharmacists and researchers.
I share the same concern, Jessica. While ChatGPT has shown impressive language processing capabilities, it's crucial to assess its reliability in predicting adverse reactions. Controlled studies comparing its predictions with real-world data would be insightful.
I believe ChatGPT can be a valuable tool, but we must exercise caution when relying solely on AI predictions. Human expertise and validation should always be an integral part of the decision-making process.
Absolutely, Natalie. AI is a powerful ally if used in conjunction with human expertise. We should aim for a collaborative approach, leveraging the strengths of both humans and AI algorithms for accurate predictions.
I think ChatGPT's ability to process natural language is impressive, but it's important to consider biases in the training data. We need to ensure that the models are trained on diverse datasets to avoid potential biases in prediction.
You're right, Megan. Bias in the data used for training can lead to biased predictions, especially in healthcare. Developers must prioritize diversity and inclusivity while curating the training datasets for AI models.
I appreciate your input, Emily and Andrew. Collaborating with AI algorithms can certainly enhance our work. It's crucial to validate ChatGPT's predictions with clinical studies and real-world data to establish its reliability.
The potential of ChatGPT in pharmaceutical applications is promising, but we should also address concerns about data privacy and security. How can we ensure that patient data is adequately protected?
Valid point, Michael. Data privacy and security are paramount when working with AI models in healthcare. Stricter regulations and safeguards should be in place to protect patient information from unauthorized access or misuse.
I agree, Megan. Patient privacy is a critical concern when dealing with AI systems that process sensitive medical data. Adhering to HIPAA regulations and implementing robust security measures will be crucial.
One potential challenge I see is the interpretability of ChatGPT's predictions. How do we understand the reasoning behind its adverse reaction predictions, especially when it comes to complex drug interactions?
Apologies, I accidentally posted the same comment twice!
Great point, Sophia. Interpreting AI models' decisions is crucial, especially in complex drug interactions. Researchers need to develop methods that provide transparency and explainability to help build trust among healthcare professionals.
Absolutely, Michael. Building trust in AI models requires transparency. If we can understand the rationale behind ChatGPT's predictions, clinicians will be more willing to incorporate the technology into their practices.
Exactly, Natalie. Ensuring transparency will be key to wider adoption and acceptance of AI tools in healthcare. We need to overcome the 'black box' perception and promote accountability in the decision-making process.
While I see the potential benefits of leveraging ChatGPT, we must also consider ethical implications. How can we prevent the misuse of AI in pharmaceuticals, such as biased recommendations or unfair prioritization of certain drugs?
Ethical considerations are crucial, Grace. To prevent misuse, clear regulations and guidelines should be established to ensure fairness and accountability in AI-driven pharmaceutical applications. Continuous monitoring is also necessary.
Apologies, I accidentally replied to the wrong comment.
No worries, Michael! I completely agree with you. Ethical frameworks should be in place to govern the development, deployment, and use of AI techniques in the pharmaceutical industry to minimize risks and ensure fairness.
I'm intrigued by the potential cost-effectiveness of utilizing ChatGPT for adverse reaction predictions. By reducing unexpected adverse reactions, we could potentially save significant healthcare costs. What are your thoughts?
Daniel, that's a great point. Preventing adverse reactions can indeed save costs associated with hospitalizations, additional treatments, and legal implications. If validated, ChatGPT's predictions can aid in reducing healthcare expenditures.
Considering the rapid advancements in AI, what future developments can we expect in this field? Will ChatGPT continue to improve and potentially become an indispensable tool in pharmaceutical research?
Oops! I accidentally posted the same comment again. My apologies!
Considering the rapid advancements in AI, what future developments can we expect in this field? Will ChatGPT continue to improve and potentially become an indispensable tool in pharmaceutical research?
Excellent question, Sophia! The potential for future improvements in ChatGPT is indeed exciting. As AI technology advances, the accuracy and reliability of predictions are likely to improve, making it an invaluable asset in various pharmaceutical research domains.
I agree with Mark. The field of AI in healthcare is evolving rapidly. As models like ChatGPT become more sophisticated and adept at processing medical data, we can expect even more accurate predictions of adverse reactions and better patient care.
All the discussions here have been enlightening! One question I have is whether there are any regulatory barriers that need to be addressed before implementing ChatGPT for adverse reaction prediction in clinical settings?
Oops, sorry for the duplicate post!
No worries, David! To your question, regulatory barriers indeed need attention. The pharmaceutical industry must work closely with regulatory bodies to develop guidelines for implementing AI models like ChatGPT in clinical settings.
Regulatory compliance will be crucial, David. We must ensure that the regulatory landscape is adapted to address AI's unique challenges in predicting adverse reactions. Collaboration between industry stakeholders and regulators is vital.
The power of AI in healthcare is awe-inspiring. However, we should not overlook the importance of educating healthcare professionals on AI's capabilities and limitations. Only then can we maximize the potential of ChatGPT.
Oops! Apologies for the repeated comment.
No problem, Liam! You make an excellent point. To ensure effective adoption, healthcare professionals should receive proper training to understand AI technologies like ChatGPT and how to incorporate them into their workflows safely.
Overall, I'm optimistic about ChatGPT's potential in predicting adverse reactions. It can help mitigate risks and improve patient safety. However, rigorous evaluation and continuous validation are necessary to establish its reliability.
Oops! My comment got duplicated. Sorry about that!
I agree with you, Sarah. Thorough evaluation with real-world data will be crucial to measure ChatGPT's effectiveness and ensure it provides reliable predictions to enhance patient safety.
Thank you all for your valuable insights and engaging in this discussion. Your thoughts and concerns are important as we navigate the future of AI in pharmaceuticals. Let's continue exploring the potential of ChatGPT while addressing the challenges along the way.
Thank you, Mark, for initiating this informative discussion. It was great learning from everyone. I look forward to witnessing the advancements in AI systems like ChatGPT and their impact on pharmaceutical research.