Revolutionizing Biomedical Text Mining in Life Sciences: Harnessing the Power of ChatGPT
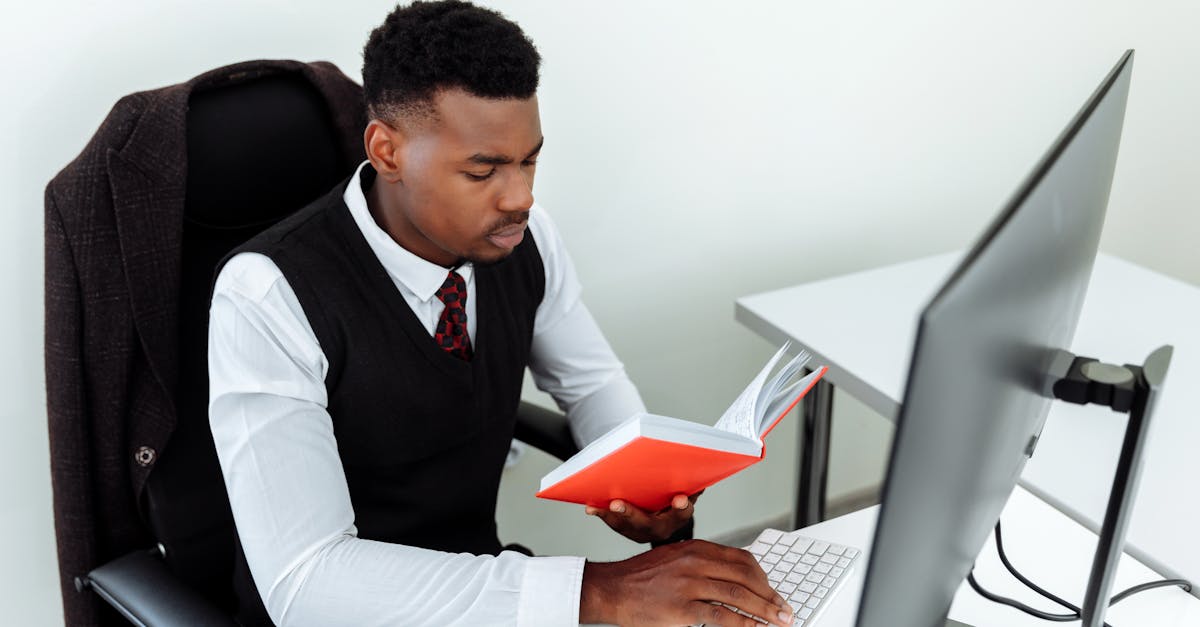
In the field of Life Sciences, advancements in technology have paved the way for various tools and techniques to support researchers in their quest for scientific knowledge. One such technology that has gained significant attention is Biomedical Text Mining. This innovative approach enables researchers to extract relevant information from vast amounts of scientific literature, facilitating the discovery of relationships between genes, diseases, and treatments.
What is Biomedical Text Mining?
Biomedical Text Mining is a technology that utilizes Natural Language Processing (NLP) and machine learning algorithms to analyze and extract valuable insights from scientific literature in the biomedical domain. With the exponential growth of published research articles, it becomes challenging for researchers to keep up with the sheer volume of information available. Biomedical Text Mining provides an automated and efficient solution to address this problem.
How Does It Work?
Biomedical Text Mining involves several steps to extract meaningful information from scientific literature:
- Text Preprocessing: Raw text documents are transformed into a suitable format for analysis. This step may include removing special characters, converting text to lowercase, and tokenizing the documents into individual words or phrases.
- Entity Recognition: Named entity recognition algorithms identify specific entities within the text, such as genes, proteins, diseases, and drug names. This step is crucial to identify the key elements for further analysis.
- Relation Extraction: Once the entities are recognized, the next step involves extracting relationships between these entities. For example, identifying the association between a particular gene and a specific disease or the relationship between a drug and its effectiveness for treating a particular condition.
- Information Integration: After the relations are extracted, the information is organized and integrated to provide a comprehensive view of the relationships between genes, diseases, and treatments. This allows researchers to gain insights and draw meaningful conclusions.
- Text Mining Evaluation: Finally, the extracted information is evaluated for accuracy and quality to ensure the reliability of the results.
Applications in Life Sciences
Biomedical Text Mining has several applications in the field of Life Sciences:
- Drug Discovery: By analyzing scientific literature, researchers can identify potential drug targets based on the relationships between genes, diseases, and treatments. This can significantly expedite the drug discovery process.
- Disease Understanding: Biomedical Text Mining helps researchers uncover hidden patterns and relationships between diseases, genes, and other biological entities. This knowledge can contribute to a better understanding of disease mechanisms and facilitate the development of improved diagnostic and therapeutic approaches.
- Literature Review: Conducting a comprehensive literature review is a crucial step in any research project. Biomedical Text Mining supports researchers in efficiently reviewing relevant scientific articles, thereby saving time and effort.
- Bioinformatics: Biomedical Text Mining complements bioinformatics approaches by providing additional insights from unstructured text data. This can enhance the accuracy and efficiency of various bioinformatics analyses.
Benefits of Biomedical Text Mining
The adoption of Biomedical Text Mining in Life Sciences offers numerous benefits:
- Efficiency: By automating the process of information retrieval and analysis, Biomedical Text Mining significantly reduces the time and effort required for researchers to gather the necessary information from scientific literature.
- High Scalability: With the exponential growth of scientific literature, Biomedical Text Mining provides a scalable solution to handle vast amounts of data and extract valuable insights efficiently.
- Discovery of Hidden Relationships: Biomedical Text Mining enables the identification of relationships between genes, diseases, and treatments that may not be easily evident from manual analysis. This can lead to novel discoveries and insights.
- Integration of Diverse Data Sources: Biomedical Text Mining can integrate information from various sources, including scientific articles, databases, and clinical records, allowing researchers to consolidate and analyze data from multiple perspectives.
- Accessibility: The extracted information can be made accessible and searchable, contributing to the creation of comprehensive knowledge bases that can be utilized by researchers across the globe.
Conclusion
Biomedical Text Mining holds great promise for the field of Life Sciences. By leveraging this technology, researchers can extract relevant information from scientific literature, enabling the discovery of relationships between genes, diseases, and treatments. With its applications in drug discovery, disease understanding, literature review, and bioinformatics, Biomedical Text Mining has the potential to revolutionize how research is conducted in the Life Sciences domain. As the volume of scientific literature continues to grow, Biomedical Text Mining will become an indispensable tool for researchers seeking to unlock the vast knowledge hidden within scientific texts.
Comments:
Thank you all for engaging with my article! I'm excited to discuss the potential of ChatGPT in revolutionizing biomedical text mining in life sciences.
This is fascinating, Taren! Do you think ChatGPT can handle the complexity and specificity of biomedical texts?
Great article, Taren! I believe ChatGPT has shown promising results in other domains, so it could definitely be a game-changer in life sciences as well.
I agree, Michael. But do you think ChatGPT can effectively deal with the ever-evolving scientific terminology in the field?
Absolutely, Emily. Leveraging pre-trained models like ChatGPT can be a major asset in handling the vast amounts of scientific literature and data in biomedical research.
Indeed, Michael. It could greatly assist researchers in extracting relevant information from large databases more efficiently.
Michael, do you think ChatGPT could facilitate knowledge sharing among researchers and reduce barriers to collaboration?
Absolutely, Julia. ChatGPT's ability to extract and summarize relevant information could help researchers with different expertise communicate and share knowledge efficiently.
That sounds really promising, Michael. It could foster interdisciplinary collaborations and ultimately accelerate scientific discoveries.
Indeed, Michael. ChatGPT's potential in assisting researchers makes it an exciting tool to explore further.
Michael, how can research institutions and organizations effectively integrate ChatGPT into their workflows?
A good question, Joseph. Organizations should invest in training researchers to leverage ChatGPT effectively and provide necessary infrastructure to integrate it into their existing workflows.
That makes sense, Michael. Proper training and support will aid in maximizing the potential benefits of ChatGPT.
I'm curious about the limitations of ChatGPT in understanding complex scientific concepts. What are your thoughts, Taren?
Good question, Rebecca. While ChatGPT is improving in its understanding capabilities, it may struggle with domain-specific knowledge and nuances. It could benefit from domain-specific fine-tuning.
That makes sense, Taren. It seems like collaboration with domain experts would be key to refine ChatGPT's understanding and accuracy.
While ChatGPT shows potential, I wonder about the risk of biases in its training data affecting the accuracy of results.
That's an important concern, John. It's crucial to ensure diverse and representative training data to minimize biases.
Taren, this article is thought-provoking! Can you give an example of how ChatGPT could be effectively applied in life sciences?
Certainly, Sophia! ChatGPT could assist researchers in automatically extracting relevant information from scientific papers, aiding in literature reviews and accelerating knowledge discovery.
That sounds promising, Taren. It could be a powerful tool in coping with the ever-increasing volume of scientific literature.
Taren, what potential challenges do you foresee when implementing ChatGPT in assisting researchers?
Great question, David! One challenge could be the need for continuous updates as new scientific advancements and domain-specific terms emerge.
I see. Keeping ChatGPT up-to-date with the latest developments would indeed be crucial to ensure accurate results.
As an AI enthusiast, I'm excited about ChatGPT's potential. Taren, do you have any insights into how ChatGPT could impact collaboration in research?
Certainly, Alicia! ChatGPT could facilitate cross-disciplinary collaborations by extracting and summarizing information from various scientific domains, bridging knowledge gaps.
That's fascinating, Taren. It could foster interdisciplinary interactions and spark new avenues of research.
Alicia, what potential challenges should organizations be aware of when implementing ChatGPT for research collaboration?
Emily, organizations should consider challenges like data security, establishing clear guidelines for collaboration, and ensuring effective communication channels between researchers using ChatGPT.
Those are important aspects to consider, Alicia. Overcoming such challenges will be crucial in successfully leveraging ChatGPT for collaborative research.
Taren, I'm curious about the potential impact of ChatGPT on reducing research time and increasing productivity. Any thoughts?
Good point, Oliver. ChatGPT has the potential to automate certain tasks, expediting the literature review process and enabling researchers to focus on higher-level analysis.
However, Taren, what precautions do you suggest to ensure that automation does not compromise research rigor and quality?
An excellent question, Lisa. While automation can enhance efficiency, researchers should carefully validate ChatGPT's outputs and maintain a critical approach in their work.
Thank you for emphasizing the importance of critical thinking, Taren. It's crucial to strike the right balance between automation and manual verification.
Taren, what are the potential privacy and ethical concerns that could arise with the widespread adoption of ChatGPT in life sciences?
Great question, Robert. Privacy concerns could arise if sensitive or personal data is exposed during the training process. Safeguarding data privacy must be a top priority.
Indeed, Taren. Ethical guidelines should be established to ensure responsible AI implementation and protect patient data.
Taren, what steps can be taken to address potential biases in ChatGPT's outputs, especially those related to underrepresented patient populations?
An important concern, Daniel. By diversifying the training data and involving diverse domain experts, we can mitigate biases and strive for more inclusive and equal representation.
Taren, do you think ChatGPT could eventually replace human experts in certain medical or research areas?
Thanks for your response, Taren. Diversifying the training data and involving experts from diverse backgrounds could indeed lead to more equitable outcomes.
That could be a game-changer! Researchers could save valuable time and allocate their efforts more efficiently.
While ChatGPT can assist and augment human expertise, I don't believe it could completely replace human experts. It can help streamline processes, but human interpretation and decision-making remain invaluable.
Thank you, Taren. I agree that human expertise is irreplaceable, and ChatGPT can be a valuable tool in assisting and advancing research.
Collaboration with domain experts is crucial indeed. By including researchers and practitioners, we can train and fine-tune ChatGPT to tackle specific challenges in life sciences.
Thank you all for the insightful comments and questions! Your engagement is greatly appreciated.
Taren, how can we ensure that the outputs generated by ChatGPT are reliable and trustworthy?
Validating the generated outputs through rigorous testing against known results, involving peer review, and ensuring transparency in ChatGPT's decision-making process can help establish reliability and trustworthiness.
I appreciate all your participation in this discussion! Your questions and insights have further enriched the potential applications of ChatGPT in biomedical text mining. Thank you!