Revolutionizing Product Retrospective Analysis: Unleashing the Power of ChatGPT in Gestión de Productos Technology
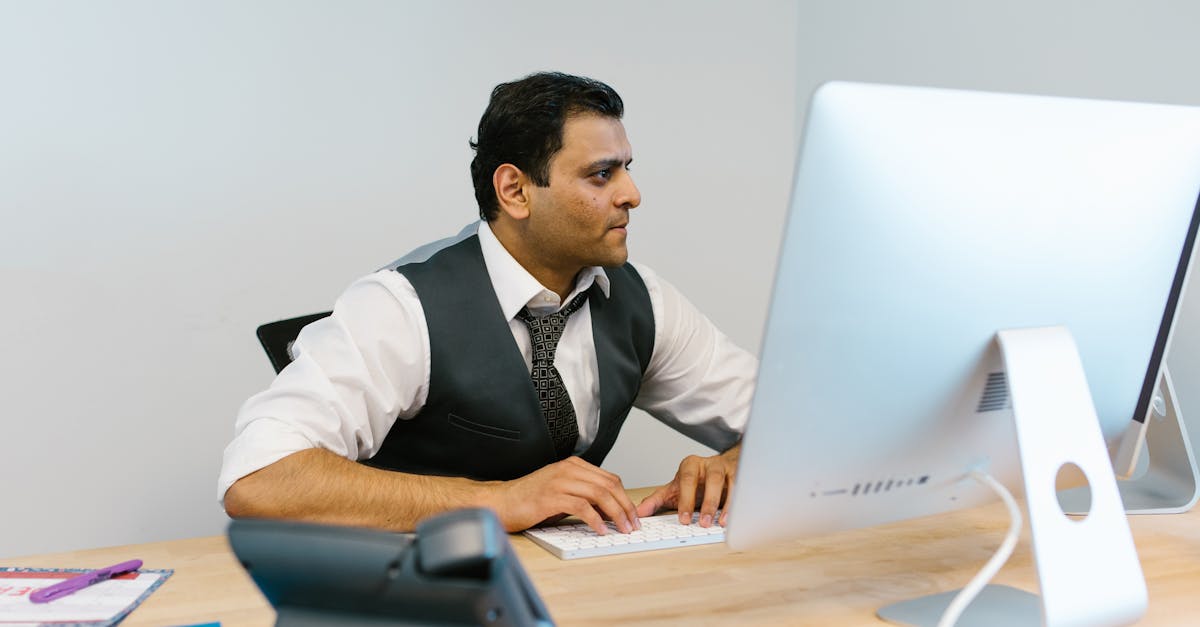
Gestión de productos, also known as product management, refers to the process of strategizing, developing, and analyzing products throughout their lifecycle. One crucial aspect of product management is conducting retrospective analysis to understand the success or failure of a product.
What is Product Retrospective Analysis?
Product retrospective analysis involves examining the factors that contributed to the outcome of a product. It aims to identify what went well, what didn't go as planned, and how to improve future product development and management processes.
The Role of ChatGPT-4 in Product Retrospective Analysis
ChatGPT-4 is an advanced AI-powered chatbot developed by OpenAI. With its natural language processing capabilities, it can assist product managers in the retrospective analysis of their products. Here's how:
1. Gathering Feedback
ChatGPT-4 can interact with users to collect feedback on a specific product. It can ask relevant questions about the product, its features, and the user's experience. The chatbot can analyze responses to identify common themes and patterns.
2. Sentiment Analysis
Using machine learning algorithms, ChatGPT-4 can perform sentiment analysis on the collected feedback. It can determine the overall sentiment of users towards the product, whether positive, negative, or neutral. This analysis provides valuable insights into customer satisfaction levels.
3. Identifying Success Factors
By analyzing positive feedback and reviews, ChatGPT-4 can identify the factors that contribute to a successful product. It can pinpoint features, functionalities, or aspects that users appreciate and find valuable.
4. Exploring Failure Factors
In cases where a product didn't perform as expected, ChatGPT-4 can help identify the potential reasons behind the failure. It can analyze negative feedback, complaints, or issues reported by users to understand what aspects need improvement.
5. Actionable Recommendations
Based on the insights gained from the retrospective analysis, ChatGPT-4 can provide actionable recommendations for product enhancements or changes. It can suggest improvements in features, user experience, marketing strategies, or any other relevant areas to boost future product success.
Benefits of using Gestión de Productos and ChatGPT-4
The combined use of Gestión de productos and ChatGPT-4 in product retrospective analysis offers several benefits to product managers and organizations:
- Efficient and automated analysis: ChatGPT-4 automates the analysis process, saving time and effort for product managers.
- Comprehensive insights: Through sentiment analysis and feedback collection, product managers gain a holistic view of their product's performance.
- Identifying strengths and weaknesses: The analysis helps identify the strengths and weaknesses of a product, enabling targeted improvements.
- Enhanced decision-making: Actionable recommendations provided by ChatGPT-4 support informed decision-making for product managers and teams.
- Iterative product development: With retrospective analysis, product managers can continually iterate and improve their products based on real user feedback and data.
Conclusion
Gestión de productos, combined with the AI capabilities of ChatGPT-4, offers a powerful solution for product managers seeking to conduct thorough retrospective analysis. By leveraging these technologies, organizations can gain valuable insights, make informed decisions, and continuously improve their products to meet customer needs and drive success.
Comments:
Thank you all for taking the time to read my article. I'm excited to discuss this topic with you!
Great article, Gary! I found the idea of using ChatGPT in product retrospective analysis very fascinating. It could indeed revolutionize the way we gather insights and reflections on product development.
Thank you, Jennifer! I believe leveraging AI-powered tools like ChatGPT can indeed bring more efficiency and depth to product retrospective analysis. It can help teams uncover valuable learnings and make informed decisions for future iterations.
Interesting concept, but won't it be biased since GPT models are trained on existing human-generated data? How would you address that concern?
That's a valid concern, David. While GPT models can carry biases from training data, efforts can be made to mitigate them. One approach is to carefully curate and diversify the training data to avoid reinforcing existing biases. Additionally, it's important to encourage open dialogue and critical thinking when analyzing the generated output to minimize the impact of any potential biases.
I see the potential, but wouldn't it be challenging for teams to adopt a new technology like ChatGPT? Change management and learning curve might impact its successful integration.
You're right, Michelle. Introducing any new technology comes with its challenges. A successful adoption would require proper change management, user training, and support. However, the potential benefits in terms of improved retrospective analysis and decision-making might outweigh the initial difficulties. It's crucial to assess the specific needs and readiness of the team before implementing such tools.
I have concerns about privacy and data security. How can we ensure that sensitive information shared during retrospective analysis doesn't get exposed or misused?
Valid point, Eric. Maintaining privacy and data security is crucial. When using ChatGPT or similar tools, it's important to evaluate and select platforms that prioritize data protection measures. Implementing encryption, access controls, and proper data handling practices can help safeguard sensitive information. It's an aspect that would need careful consideration when adopting such technologies.
I can definitely see the benefits of using ChatGPT in retrospective analysis. It could encourage more inclusive participation and reduce the bias that can arise when facilitators dominate the conversation. Exciting possibilities!
Absolutely, Samantha! By using ChatGPT, all participants can have an equal voice, and it can help create a more inclusive and collaborative environment during retrospective analysis. It promotes unbiased conversations and allows teams to tap into diverse perspectives and insights.
While the idea is intriguing, have there been any real-world implementations or case studies showcasing the effectiveness of using ChatGPT in product retrospective analysis?
Good question, Daniel. While ChatGPT is a relatively new technology, there have been initial experiments and pilot projects exploring its usage in retrospectives. Some teams have reported positive results in terms of generating valuable insights and facilitating constructive discussions. However, more extensive real-world implementations and rigorous studies are needed to fully assess its effectiveness across different contexts.
I'm curious about the potential limitations of using ChatGPT. Are there any specific scenarios where it might not be as effective or accurate?
Great question, Michael. Like any AI-powered tool, ChatGPT has limitations. It might struggle with understanding complex or ambiguous queries, and occasionally produce incorrect or nonsensical responses. Moreover, it's important to consider the need for human facilitation and the value of face-to-face interactions in certain retrospective scenarios. ChatGPT can be a valuable addition, but it's not a complete replacement for traditional methods.
I can see the potential for ChatGPT in retrospective analysis, but would it be suitable for all types of product teams? For example, how about hardware or physical product development teams?
That's a good question, Alexandra. While ChatGPT is primarily text-based, it can still be useful for hardware or physical product development teams. Feedback and insights from team members can be captured through text, and the analysis can focus on aspects like collaboration, decision-making processes, and timeline management. However, it might be necessary to adapt the framework to suit the specific needs and context of non-software product teams.
Regarding bias in GPT models, I think it's important to iterate on the training process and constantly monitor and address biases as they're discovered. Transparency and accountability are key.
Well said, Grace! Iterative training and continuous monitoring are crucial to identify and address biases in GPT models. Transparency about the methods and data used in training is important. Further research and collaboration within the AI community can help in creating more accountable and unbiased AI systems.
I completely agree, Grace and Gary. Ensuring transparency and accountability will be essential to build trust in the use of ChatGPT for retrospective analysis. This will be important not only concerning bias but also in terms of explaining how the AI arrives at its responses.
Another aspect to consider regarding data security is the anonymization of user input. Having the ability to provide feedback and reflections anonymously might encourage more honest and open responses from team members.
Great point, Sophia. Anonymity can play a significant role in building trust and encouraging honest feedback during retrospective analysis. By providing an option for anonymous input, teams can create a safe environment where team members feel comfortable expressing their thoughts without fear of judgement or reprisal.
Are there any known challenges or limitations when it comes to training GPT models specifically for retrospective analysis? How can one ensure the model understands the context accurately?
Good question, Ethan. Training GPT models for retrospective analysis can be challenging due to the need to capture and understand the specific terminology, domain knowledge, and contextual nuances of product development. It requires a well-curated dataset that includes relevant retrospective examples and discussions to help the model grasp the context accurately. It's an iterative process that involves fine-tuning the model based on feedback and continuous improvement.
I'm wondering if using ChatGPT could hinder team dynamics by depersonalizing the retrospective process. Would it create a sense of detachment among team members?
That's an interesting thought, Samuel. While ChatGPT can automate certain aspects of retrospective analysis, it's important to strike a balance to prevent depersonalization. Integrating human facilitation and incorporating face-to-face discussions where appropriate can help maintain team dynamics and foster meaningful interactions. The aim should be to leverage ChatGPT as a helpful tool without losing the human element.
I think one potential benefit is having a centralized platform for retrospective analysis where all conversations and insights are recorded. It could be valuable for capturing historical knowledge and enabling future reference.
Absolutely, Emily! Having a centralized platform powered by ChatGPT can facilitate knowledge sharing and retention. The recorded conversations can serve as a valuable reference for future retrospectives, ensuring that insights and learnings are not lost over time. It can contribute to building a knowledge base and fostering continuous improvement within product teams.
What about teams with members who prefer more traditional methods? How can we encourage their participation and ensure that everyone benefits from using ChatGPT?
Good question, Oliver. It's important to consider the preferences and comfort levels of all team members. Introducing ChatGPT should be seen as a complement to traditional methods, not a replacement. Ensuring proper training, support, and emphasizing the value it brings can help encourage participation and adoption among those who prefer more traditional approaches. Making the transition gradual and showcasing the benefits through tangible outcomes can make it easier for everyone to see the value.
How efficient would ChatGPT be in handling different languages during retrospective analysis? Is it optimized for multilingual teams?
Good point, Richard. ChatGPT's efficiency in handling different languages may depend on the language models and training data available. While the initial models were English-focused, efforts are being made to expand and optimize models for multilingual teams. With proper training and data, ChatGPT has the potential to assist in retrospective analysis across different languages, enabling global collaboration and knowledge sharing.
Transparency in AI decision-making is crucial, especially when using tools like ChatGPT. Users should be able to understand why and how specific responses are generated to trust and validate the output.
Absolutely, Kimberly. Transparency builds trust, and it's vital in AI-powered systems. Making the decision-making process of ChatGPT more transparent and explainable can help users understand the rationale behind the responses it generates. By providing visibility into the underlying mechanisms and enabling users to validate the output, we can ensure accountability and promote responsible adoption of such technologies.
Would using ChatGPT eliminate the need for a facilitator or moderator during retrospective analysis?
Not necessarily, Nathan. While ChatGPT can automate certain tasks and facilitate discussions, having a skilled facilitator or moderator can bring immense value to the retrospective analysis process. They can ensure smooth facilitation, encourage equal participation, and drive meaningful conversations. ChatGPT can support the facilitator's role but cannot completely replace their expertise in guiding the session effectively.
What kind of training or preparation would product teams need before integrating ChatGPT into their retrospective analysis process?
Good question, Robert. Before integrating ChatGPT, teams would benefit from training and familiarizing themselves with the tool's capabilities and limitations. Understanding how to make the most of the generated responses, interpreting them critically, and effectively integrating them into the retrospective process would be important. Additionally, providing guidelines and training on giving constructive feedback to the AI model can also enhance the overall experience.
Since ChatGPT relies on text-based interactions, could it potentially hinder the non-verbal cues and expressions that contribute to effective communication and understanding within a retrospective?
Valid concern, Victoria. Non-verbal cues play an important role in communication, and ChatGPT's text-based nature doesn't capture them. In certain scenarios, it might be necessary to supplement ChatGPT interactions with in-person or video-based discussions to maintain the richness of communication. Evaluating when and how to incorporate non-verbal cues can enhance understanding and ensure effective retrospective analysis.
What are the considerations when determining the right balance between automated analysis using ChatGPT and human-driven analysis in retrospective sessions?
Excellent question, Jonathan. The balance between automated and human-driven analysis would depend on the specific needs, available resources, and the nature of the retrospective session. Automated analysis using ChatGPT can provide valuable insights efficiently, but human-driven analysis allows for contextual understanding, expert interpretation, and adaptability. Striking the right balance might involve using ChatGPT for generating initial insights and then leveraging human expertise for deeper analysis and synthesis.
Are there any specific challenges or limitations when it comes to scaling up ChatGPT for larger teams or organizations?
Good point, Anne. Scaling up ChatGPT for larger teams or organizations may present challenges related to managing multiple conversations, ensuring response quality across different user inputs, and handling increased computational demands. Performance, reliability, and user experience considerations become magnified at scale. Solutions would involve efficient conversation tracking, model optimization, and infrastructure enhancements to maintain satisfactory performance and support widespread adoption.
I wonder how ChatGPT can handle situations where there are disagreements or conflicts during retrospective analysis. Are there any mechanisms to facilitate resolution in such cases?
That's an important aspect to consider, Olivia. In cases of disagreements or conflicts, ChatGPT can assist by providing alternative perspectives and potential solutions. It can help facilitate resolution by encouraging open communication, guiding parties to consider different viewpoints, and emphasizing constructive dialogue. However, it's essential to recognize that certain conflicts may require human intervention or additional techniques for conflict resolution within the retrospective process.
How would you compare the effectiveness of using ChatGPT in retrospective analysis to more traditional methods like face-to-face discussions or surveys?
Great question, Thomas. Comparing the effectiveness of ChatGPT to traditional methods depends on various factors like the nature of the retrospective, team dynamics, and the desired outcomes. Face-to-face discussions offer real-time interaction and non-verbal cues, fostering deeper understanding and empathy. Surveys provide structured data but lack the richness of conversations. ChatGPT strikes a balance by automating aspects, enabling inclusive participation, and providing teams with valuable insights. It can complement and enhance traditional methods but not entirely replace them.
What potential risks should organizations consider when adopting ChatGPT for retrospective analysis?
Good question, Jake. Organizations should consider risks such as potential biases in the AI-generated responses, data security and confidentiality, dependence on the technology, and overreliance without critical analysis. Lack of proper training and support for team members, especially in the early stages, can also hinder successful adoption. Being cognizant of these risks and proactively addressing them through responsible implementation and continuous improvement can contribute to a successful adoption of ChatGPT.