Revolutionizing Technology's 'Aperture': The Power of ChatGPT
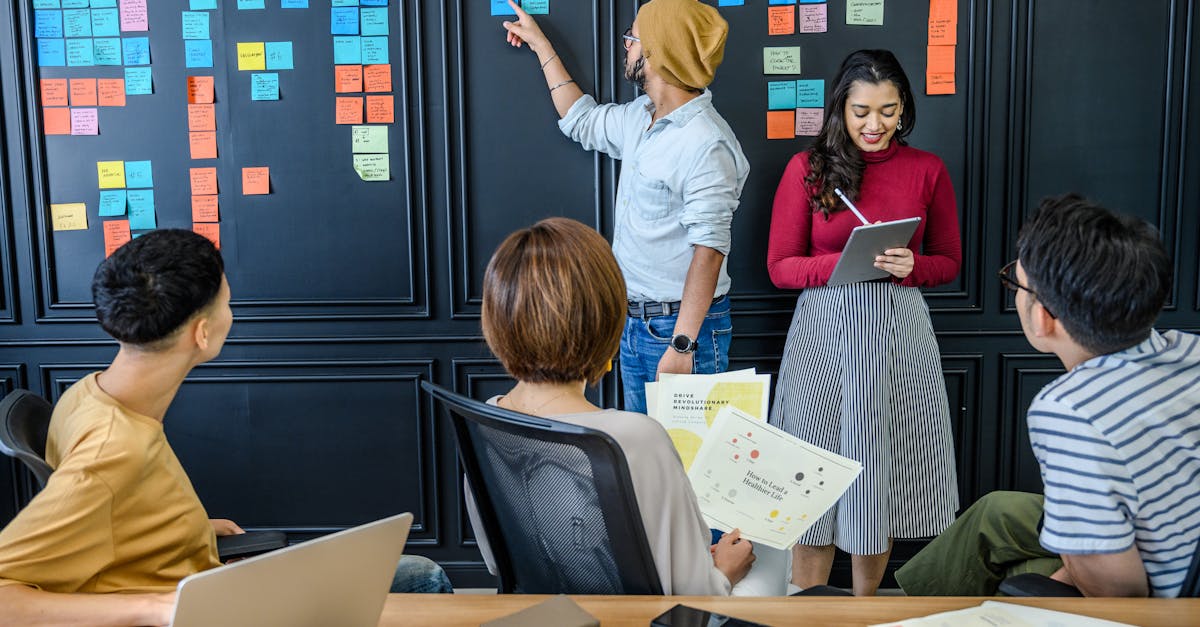
Modern technology plays a significant role in enhancing knowledge management. One particular technology that is making waves in the field is Aperture. With the fast-paced generation of data and information in organizations, the term 'Aperture' is frequently used as a metaphor representing a tool or method that allows individuals or organizations to view, understand, and manage knowledge. This article explores the concept of Aperture in Knowledge Management and how it can be applied to the latest AI technology - ChatGPT-4, to facilitate efficient cataloguing and retrieval of information.
Understanding Aperture in Knowledge Management
Aperture, from a technological sense, refers to a tool that provides an opportunity for organizations to look into the vast amount of knowledge and information they possess and manage it efficiently. It provides an overall view of the data, helping identify which areas need attention, assess information gaps, and decide where to concentrate resources for knowledge generation and application. Hence, it serves as a 'lens' through which companies can concentrate their insights generation and data interpretation.
ChatGPT-4: The Next Generation AI
ChatGPT-4 is the latest version of OpenAI's chatbot series. It has advanced Natural Language Processing (NLP) capabilities and utilises massive datasets to learn, understand, and generate human-like text. Its abilities extend to answering questions, writing articles, and even making specific decisions based on the data it obtains.
How Aperture Enhances ChatGPT-4's Knowledge Management Capabilities
Aperture's concept applied to ChatGPT-4's technology can significantly enhance its knowledge management capabilities. It will be viewed in the metaphorical sense as the methods or algorithms applied to allow the AI to manage and retrieve the vast amount of data it takes in efficiently.
Application of Aperture technology would enable ChatGPT-4 to scan its information database and understand which pieces of data or information are relevant to a particular query or task. This kind of data analysis and interpretation is crucial in tasks such as question-answering, where the AI needs to access a vast database of knowledge and pull out the correct, relevant information.
Automated Cataloguing and Efficient Information Retrieval
With 'Aperture', the AI could categorize data in a manner that allows for quick and easy access. This 'aperture' of knowledge would be based on the AI's understanding of textual content and its ability to categorize and store it in an organized manner. This organized cataloguing of data would be automatic, requiring no human intervention, thereby saving time and resources.
This framework would serve as a basis for information retrieval, making it easier for the AI to provide accurate and relevant responses to queries. Better-organized data means more efficient retrieval, and efficient retrieval translates to better, more accurate service.
Conclusion
In conclusion, the application of Aperture to ChatGPT-4 provides a promising method for more efficient and effective Knowledge management. It strikes a balance between human-like understanding, automatic cataloguing, and efficient retrieval of information, making it an asset for any organization seeking to harness the power of AI for their knowledge management.
Comments:
Thank you all for reading my article on Revolutionizing Technology's 'Aperture': The Power of ChatGPT. I'm excited to hear your thoughts and have a fruitful discussion!
Great article, Ethan! The advancements in AI language models like ChatGPT are truly impressive. Can't wait to see how this technology evolves further.
Thank you, Lisa! I agree, the progress in AI language models is remarkable. It opens up new possibilities in various fields.
While the potential is exciting, we must also be cautious about the ethical implications of AI language models. Privacy concerns, misinformation, and biases are a few challenges that need to be addressed. Thoughts?
Absolutely, Mark. The responsibility to mitigate these risks lies with developers and researchers. There should be more transparency and accountability in the development and deployment of AI models.
Valid points, Mark and Emily. Ethical considerations are crucial, and it's essential to prioritize fairness, bias reduction, and user safety as AI technology progresses.
I've personally found ChatGPT to be incredibly useful in my work. It saves time and helps generate ideas. However, sometimes it can produce inaccurate or misleading responses. Fine-tuning it for specific domains might improve its reliability.
Thank you for sharing your experience, Sarah. You're right, fine-tuning models for specific domains can enhance their performance and accuracy.
Ethan, your article mentioned applications in customer service. How well does ChatGPT handle customer inquiries and issues compared to traditional support systems?
Good question, Chris. While ChatGPT has shown promise in handling customer inquiries, it's not without limitations. It may struggle with nuanced or complex problems and should be used alongside human-supervised systems.
Ethan, in response to your earlier comment, I think striking the right balance between automation and human supervision is critical for AI systems like ChatGPT. Human oversight can help mitigate risks and ensure responsible use.
Absolutely, Sarah. Human supervision and oversight are essential, especially when it comes to sensitive areas or critical decision-making. Finding the right balance ensures the best outcomes.
The ability of AI models like ChatGPT to understand context and generate coherent responses is impressive. It's a significant step towards more natural human-AI interactions.
Indeed, Amy. Capturing context and improving response coherence are crucial factors in making AI interactions feel more human-like.
Ethan, in response to your earlier comment, do you think AI models like ChatGPT will become capable of generating creative written content independently, beyond just assisting human writers?
While AI models have shown progress, Amy, I believe that human creativity and the unique touch we bring to creative writing will continue to be a vital aspect. AI models like ChatGPT can offer valuable assistance, but their independent creative capabilities may not match the human touch.
I'm curious about the computational resources and infrastructure required to deploy large-scale AI models like ChatGPT. Are they accessible for smaller organizations or individuals?
Great point, Daniel. The computational resources required can be substantial, but efforts are being made to democratize AI model deployment. Smaller organizations and individuals can leverage cloud computing platforms to access these models more easily.
As an AI enthusiast, I must say that ChatGPT has come a long way, especially in generating human-like text. It's fascinating to witness this rapid progress, but I wonder if there are potential risks associated with it too.
You're right, Grace. While the progress is exciting, we need to be mindful of risks like bias amplification, misuse, and unintended consequences. Responsible development and usage are crucial.
The possibilities of AI language models in creative writing and content generation are intriguing. Do you think they could replace human writers entirely in the future?
It's an interesting thought, Michael. While AI models have shown impressive capabilities, I believe human creativity and the unique perspective we bring will continue to play a vital role in writing and content creation.
ChatGPT has the potential to revolutionize education by providing personalized learning experiences. Imagine AI-powered tutors that adapt to the needs of each student. Exciting!
Absolutely, Oliver. Adaptive learning powered by AI has the potential to transform education, making it more engaging and tailored to individual students' needs.
Ethan, do you think ChatGPT can be utilized effectively in online learning platforms to support students?
I appreciate how you highlighted the importance of human-AI collaboration for leveraging the full potential of models like ChatGPT. We need to consider them as tools to augment human capabilities, not replace them.
Well said, Rachel. The synergy between humans and AI can achieve greater outcomes than either working alone. Collaboration is key to realizing the benefits of advanced AI models.
I'd love to hear more about the research behind ChatGPT's development. Are there any specific areas of focus to enhance its capabilities?
Good question, Lauren. Ongoing research aims to improve important aspects like reducing biases, addressing safety concerns, and tackling limitations in handling complex queries effectively.
ChatGPT has significant potential in the healthcare sector. AI models could assist doctors in diagnosing illnesses, predicting outcomes, and offering personalized treatment suggestions. Excited for the advancements!
Absolutely, Brian. AI's role in healthcare is promising, from improving diagnostics to optimizing treatments. It can augment medical professionals and contribute to better patient care.
Regarding natural language understanding, how well does ChatGPT handle different languages? Are there plans to expand its capabilities beyond English?
Great question, Sophia. While ChatGPT has primarily been trained on English text, there are plans to expand its language capabilities. Efforts are underway to ensure it can understand and generate text in multiple languages.
The potential of ChatGPT in enhancing accessibility for people with disabilities is worth exploring. AI models could help provide better communication and support for those with speech or cognitive impairments.
You're absolutely right, Karen. Accessibility is an important area where AI can make a positive impact. AI models like ChatGPT have the potential to assist individuals with disabilities and enable better inclusion.
Impressive article, Ethan. It's fascinating to see AI language models evolve over time. The advancements in ChatGPT bring us closer to more sophisticated human-AI interactions.
Thank you, Rebecca. Indeed, the evolution of AI language models like ChatGPT is exciting, and I'm glad you found the article informative.
While AI language models have their limitations, they're undeniably powerful tools. When combined with human creativity and judgment, they can unlock tremendous potential.
Precisely, Matthew. AI language models like ChatGPT are best used in collaboration with human expertise to maximize the benefits they offer.
The progress in AI language models like ChatGPT is astonishing. I'm eager to see how they'll shape the future of communication and problem-solving across various industries.
Indeed, Robert. AI language models have immense potential to revolutionize communication and problem-solving, empowering industries to advance and adapt in new ways.
I appreciate how the article highlighted the importance of continuous model improvement and community collaboration. It's a collective effort to address challenges and ensure responsible AI development.
Absolutely, Emily. Continuous improvement and collaboration are key in building better AI models, addressing concerns, and ensuring they serve the needs of users and society.
AI language models like ChatGPT undoubtedly have exciting potential, but there's also a responsibility to educate users on their limitations to prevent misinformation or blind reliance on their responses.
You're absolutely right, Michael. Educating users about AI model limitations is crucial to manage expectations and avoid undue reliance on their responses. Context and critical thinking remain essential.
The democratization of AI technology, like ChatGPT, has the potential to bridge the gap between experts and non-experts in various fields. It can empower individuals to access and utilize domain-specific knowledge more effectively.
Absolutely, Sophie. By making AI technology accessible, individuals across different domains can benefit from its potential to assist and augment their expertise.
I'm impressed by the versatility of ChatGPT. Its application potential seems vast, ranging from content generation to gaming experiences. This technology truly has a wide scope!
Indeed, Lucas. The versatility of ChatGPT allows for diverse applications across industries, making it an exciting technology with a wide range of possibilities.
I'm curious about the energy consumption associated with training and deploying AI models like ChatGPT. Are there efforts to make them more energy-efficient?
Great question, Elizabeth. Energy efficiency is an important area of focus for AI model development. Researchers are actively exploring techniques to reduce energy consumption during training and deployment.
I find AI language models like ChatGPT fascinating, but concerns about their potential misuse and deepfake generation are valid. Responsible development and usage norms must be established.
Absolutely, Adam. Responsible development and usage norms are crucial to prevent misuse and mitigate risks associated with AI language models. It's important to establish ethical guidelines.
Can you explain the difference between GPT, or Generative Pre-trained Transformer, and ChatGPT? Is ChatGPT a specialized version?
Certainly, Jessica. ChatGPT builds upon the GPT architecture but is fine-tuned using methods that make it more suitable for interactive conversations. It's designed to generate coherent and contextually relevant responses in a chat-like setting.
Considering the rapid advancements in AI language models, how do you envision their impact on employment and job markets in the long run?
A valid concern, Alex. While AI models bring changes to job markets, they also create new opportunities. Repetitive or mundane tasks may be automated, allowing humans to focus on more complex and creative endeavors.
I enjoyed your article, Ethan. The potential applications of ChatGPT in enhancing virtual assistant technologies seem promising. Do you think it can revolutionize the way we interact with our devices?
Thank you, Samantha. Absolutely, ChatGPT and similar AI models have immense potential to revolutionize virtual assistance by enabling more natural and intuitive interactions with devices.
Impressive article, Ethan. AI models like ChatGPT have significant implications in improving accessibility and inclusion by providing better digital support to individuals with disabilities.
Thank you, Ryan. AI models can indeed contribute to enhancing accessibility, offering improved digital support to individuals with disabilities and fostering greater inclusion.
The article highlights the importance of user feedback to improve AI models. How can developers effectively incorporate user feedback into the model's development process?
Great question, Jennifer. Developer feedback loops and user studies play a significant role in improving AI models. Gathering user feedback, identifying limitations, and fine-tuning the models accordingly helps refine their performance and address biases.
AI technology like ChatGPT has the potential to transform the way we approach research and information retrieval. It can help streamline knowledge acquisition and discovery.
Absolutely, Andrew. AI models like ChatGPT can assist researchers and individuals in exploring and accessing relevant information, accelerating the knowledge acquisition process.
The article mentions that ChatGPT has limitations regarding biased or politically charged responses. How can these issues be effectively addressed to ensure fairness?
Valid concern, Mary. Addressing biases is an ongoing effort. Researchers are working on techniques like data filtering, prompt engineering, and external review processes to reduce biased or politically charged responses, ensuring a more fair and balanced system.
What measures are in place to prevent malicious actors from exploiting AI language models like ChatGPT to spread misinformation or engage in harmful activities?
A pressing concern, Jessica. Detection mechanisms, user feedback loops, and ongoing research help combat misuse. Collaborative efforts between developers, researchers, and the wider community are crucial in mitigating potential risks and ensuring responsible usage of AI language models.
AI advancements like ChatGPT open up exciting possibilities for creative collaboration and co-creation between humans and machines. It's a fusion of the best of both worlds!
Well said, Gregory. The collaboration between humans and AI models like ChatGPT can unlock new dimensions of creativity and co-creation, enabling us to achieve more together.
Impressive article, Ethan. Can you share any noteworthy real-world use cases where ChatGPT or similar models have shown substantial impact?
Thank you, Stephanie. ChatGPT and similar models have demonstrated promising results in areas like content generation, language translation, and text completion. They have the potential to enhance customer support interactions and aid in writing assistance.
Considering the dynamic nature of conversations, how does ChatGPT handle sarcasm, irony, or humor? Can it capture nuances effectively?
Good question, Peter. While ChatGPT has shown improvement in understanding nuances, it can still struggle with complex facets like sarcasm or irony. Handling humor effectively remains a challenge and an active area of research.
Brilliant article, Ethan. AI language models like ChatGPT become powerful collaboration tools when combined with human ingenuity. Together, we can push boundaries!
Thank you, Carlos. Collaboration between AI models and human ingenuity indeed opens up new possibilities and helps us excel in various domains. Pushing boundaries together is the way forward!
AI models like ChatGPT have immense potential in the field of creative arts. They can assist with generating ideas, providing inspiration, and pushing artistic boundaries. Exciting times for artists!
Absolutely, Lauren! AI models like ChatGPT offer creative support to artists, enabling them to explore new horizons, experiment, and find inspiration. The fusion of art and AI is indeed remarkable.
I appreciate how the article highlighted the importance of responsible AI development. AI language models should be designed and deployed with ethical considerations at the forefront.
Thank you, Rachel. Responsible AI development is paramount. By prioritizing ethics and ensuring transparency, developers and researchers can build AI systems that benefit society while minimizing potential risks.
It's exciting to see how AI language models like ChatGPT can aid in language learning. They can provide personalized practice, instant feedback, and assist in language understanding. A boon for language enthusiasts!
Absolutely, Lucy. AI language models can greatly support language learning, offering personalized practice and real-time feedback, helping learners improve their language understanding and proficiency.
The article rightly emphasizes the need for AI models like ChatGPT to be transparent and interpretability. Users should be able to understand how the responses are generated.
You're absolutely right, Sophie. Ensuring transparency and interpretability of AI models like ChatGPT builds trust and helps users understand how the system generates responses.
AI language models that can conversate naturally like ChatGPT have massive implications for digital storytelling and interactive narratives. It opens up new dimensions for immersive experiences!
Indeed, Emily. AI language models like ChatGPT can transform the realm of digital storytelling, offering more immersive and interactive narratives, enhancing user experiences, and pushing the boundaries of creativity.
Ethan, addressing the limitations head-on will help refine the technology and make it more reliable and useful.
Emily, refining ChatGPT's contextual understanding will undoubtedly be crucial for its further development.
I'm curious about the computational resources and infrastructure required to deploy large-scale AI models like ChatGPT. Are they accessible for smaller organizations or individuals?
Great point, Robert. While the computational resources required can be substantial, cloud computing platforms offer accessibility to smaller organizations and individuals, enabling them to leverage large-scale AI models like ChatGPT.
AI models like ChatGPT have incredible potential in preserving and sharing cultural heritage. They can assist in language preservation and reviving historical texts. A boon for cultural preservationists!
Absolutely, Emma. AI models like ChatGPT can contribute significantly to cultural preservation efforts by assisting in language preservation, translation, and reviving historical texts, ensuring our collective heritage is not lost.
The progress in AI language models is truly remarkable, Ethan. They have the potential to reshape various industries and the way we interact with technology. The future holds exciting possibilities!
Thank you, David. The progress made in AI language models is indeed remarkable, and it's exciting to witness their potential to reshape industries and enhance our interactions with technology.
I'm curious about the data privacy considerations when using AI models like ChatGPT. How can we ensure user privacy and prevent sensitive information leakage?
Valid concern, Sophia. Safeguarding user privacy is crucial. AI models and systems need to be designed with privacy in mind, ensuring data protection and adopting practices that prevent sensitive information leakage.
The analysis and insights provided by AI models like ChatGPT can help businesses make more informed decisions and improve their operations. Excited to witness the AI-driven transformation!
Absolutely, John. AI models like ChatGPT can provide valuable analysis and insights, empowering businesses to make data-driven decisions, optimize processes, and drive transformative change.
Given the rapid advancements in AI, what do you think is the most exciting direction for AI language models like ChatGPT in the near future?
A great question, Sophie. I believe making AI language models more interactive, context-aware, and capable of nuanced understanding will be a significant focus in the near future. Enabling more dynamic and natural conversations is an exciting direction.
AI language models like ChatGPT can be valuable tools in facilitating cross-cultural communication and bridging language barriers. The potential for global impact is enormous!
Absolutely, Daniel. AI language models can help bridge language barriers, facilitating cross-cultural communication, and enabling greater global collaboration. Their potential impact on connecting people is immense.
Thank you all for taking the time to read my article on 'Revolutionizing Technology's 'Aperture': The Power of ChatGPT'. I'm excited to hear your thoughts and engage in a fruitful discussion.
Great article, Ethan! The potential of ChatGPT to revolutionize technology is truly fascinating. It's impressive how it can learn and adapt to provide more accurate and contextual responses.
I completely agree, Claire. In terms of natural language understanding, ChatGPT seems to have made significant strides. The examples shared in the article showcase its ability to comprehend complex queries.
ChatGPT's potential for applications in customer service is immense. With improvements, it could greatly enhance user experiences and streamline support processes.
Agreed, Olivia! The technology's ability to generate human-like responses can contribute to a more personalized customer service approach.
Olivia, that's a great point! A more personalized approach in customer service can result in better overall satisfaction and loyalty.
While ChatGPT is undoubtedly impressive, there are concerns about its ability to generate false or misleading information. It's crucial to address this issue before deploying it on a larger scale.
That's a valid concern, Lucas. Overcoming biases and ensuring the AI system provides accurate and reliable information should be a top priority.
I find the idea of a chatbot that can assist users in various domains extremely intriguing. The article mentions ChatGPT being used for coding assistance, which sounds quite promising.
Absolutely, Isabella! Having an AI-powered chatbot that can provide coding support would definitely be invaluable for developers, especially beginners.
Michael, as someone who's new to coding, I can definitely see the appeal of having a helpful coding assistant like ChatGPT.
Isabella, as an experienced coder, I can say that an AI assistant like ChatGPT would be a game-changer for both beginners and professionals.
One potential drawback of ChatGPT is its inability to ask clarifying questions when faced with ambiguous queries. This limits its effectiveness in certain scenarios.
I agree, Liam. Contextual understanding is crucial, and the ability to ask clarifying questions would enhance the usefulness of ChatGPT even further.
Emily, you're absolutely right. Ensuring ChatGPT can seek clarifications would be a significant improvement.
Liam, you raise a valid concern. The inability of ChatGPT to seek clarifications can limit its usefulness in certain situations.
Michael, I think it's intriguing how AI advancements like ChatGPT can bridge the knowledge gap and make coding more accessible.
Michael, exactly. The inability to seek clarifications may limit ChatGPT's effectiveness compared to human agents in complex scenarios.
Exactly, Emily. These precautions are necessary to prevent AI systems from amplifying biases or spreading misinformation inadvertently.
The ethical considerations surrounding AI systems like ChatGPT cannot be ignored. We must ensure responsible development and usage to prevent potential harm.
Indeed, Joshua. We must prioritize ethics to ensure AI technologies are developed and deployed responsibly.
Absolutely, Lucas. Proactive measures to mitigate biases and misinformation should be incorporated into the development process.
Lucas, you bring up an important point. Responsible AI development means considering the societal and ethical implications of these powerful technologies.
The implications for education are also worth exploring. ChatGPT could be a valuable tool for personalized learning and student support.
Thank you all for sharing your perspectives! It's fascinating to see the various ways ChatGPT's potential is being evaluated and considered.
Ethan, I appreciate your article's in-depth analysis. It provides valuable insights into the capabilities and challenges of ChatGPT.
Ethan, have you come across any limitations of ChatGPT that were not mentioned in your article? I'd love to hear your thoughts.
Max, there are indeed some limitations. ChatGPT can sometimes produce plausible-sounding but incorrect or nonsensical answers. It also tends to be sensitive to input phrasing, where slight rephrasing can yield different responses.
Ethan, have there been any documented cases of ChatGPT providing misinformation or inaccurate advice?
Ethan, it's good to be aware of those limitations. Fine-tuning ChatGPT to handle such cases will be essential for its practical applications.
Max, another limitation is ChatGPT's tendency to be excessively verbose, often producing unnecessarily long and convoluted answers.
I appreciate your insights, Ethan. These limitations provide areas for future research and development to make ChatGPT more robust.
Ethan, I think the potential for ChatGPT lies in its ability to bridge the human-AI interaction gap further, allowing for more seamless collaboration.
Max, that's an interesting question. Ethan, I'm also curious about your insights on ChatGPT's limitations.
Claire, while ChatGPT has been impressive, there have been instances where it generates inaccurate responses. It's essential to validate its outputs and ensure reliable information.
Ethan, addressing ChatGPT's limitations and ensuring factual accuracy is key for widespread trust and adoption of the technology.
Indeed, responsible development and continuous monitoring to detect biases and misinformation are crucial aspects to consider.
Absolutely, a personalized approach can humanize the customer support experience in a world becoming increasingly reliant on technology.
Ethics should definitely be at the forefront of developing AI systems. The potential benefits cannot outweigh the potential harm.
Joshua, I agree. Ethical considerations should guide the development and usage of AI systems like ChatGPT to prevent any unintended harm.
Oliver, absolutely! Personalized learning and support could greatly benefit from AI systems like ChatGPT, tailoring educational experiences to individual needs.
I enjoyed reading the article, Ethan. The potential of ChatGPT is indeed exciting, though we need to be cautious about its limitations.
Sarah, indeed, being aware of the limitations is crucial. Careful deployment and user education are necessary to avoid potential drawbacks.
Thanks, Claire. You're right, trust is essential, and addressing limitations will be crucial for the wide adoption of AI technologies like ChatGPT.
Ethan, how do you envision the future of ChatGPT? Are there any specific improvements or directions you hope to see?
Claire, in the future, I hope to see ChatGPT becoming more adaptable to user preferences and providing explanations behind its responses to enhance transparency.
Sarah, being cautious about the limitations is indeed important. Continuous improvement is necessary to harness the full potential of ChatGPT.
Thank you all for the insightful comments and questions. Your perspectives have added depth to the discussion, and I appreciate your engagement.