Revolutionizing the Reorganisation: How ChatGPT Transforms Technology
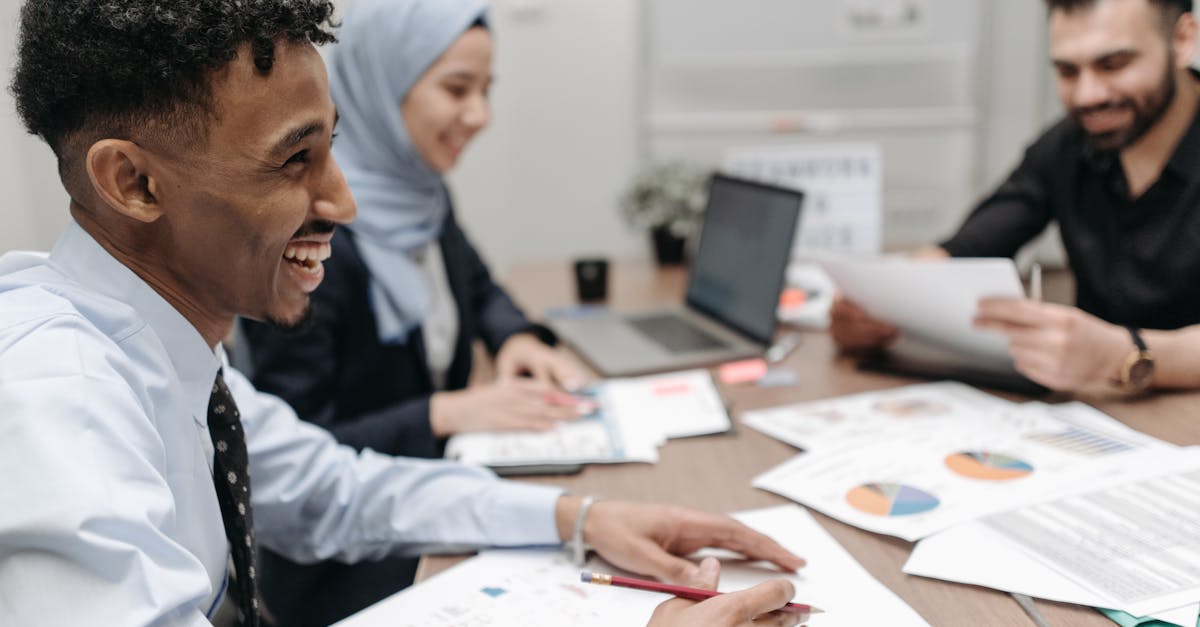
The ever-evolving landscape of technology has fostered an exponential increase in the volume, variety, and velocity of data that businesses have at their disposal. An aspect of technological advances that helps manage this outpouring of information is 'Reorganisation'. In the realm of data management, the concept of reorganisation is key to maintaining, interpreting and efficiently managing the vast collections of data.
Reorganisation in data management, essentially, is about structuring and restructuring complex data structures to make them easier to interpret and handle. Think of it as tidying up a messy room; it’s about rearranging the furniture (data) into a more logical, efficient layout that enhances usability and ease-of-access. This is usually done by the utilisation of certain algorithms and methodologies that systemise and order data in a more coherent and user-friendly manner.
Role of Reorganisation in Data Management
Reorganisation plays a critical role in data management. Its significance can be seen in various areas such as data storage, data mining, data analysis, and data retrieval among others. It bolsters operational efficiency by eliminating redundancies, managing storage, and reducing retrieval time. It also aids in data accuracy and quality management, making sure that the data obtained is reliable and clean. Furthermore, organised data strengthens security measures by ensuring a sturdy architecture resistant to breaches and leaks.
Usage of Reorganisation Technology in ChatGPT-4
OpenAI’s ChatGPT-4 is an excellent example of using reorganisation techniques to manage complex data structures. As a language prediction model, ChatGPT-4 needs to handle a mammoth amount of structured and unstructured data to deliver its functionality. The system applies reorganisation techniques like Data Matchmaking, Data Cleaning, Data Indexing and Data Linking to the incoming load of data.
Data Matchmaking
ChatGPT-4 utilises data matchmaking to coordinate and connect relevant pieces of information. By comparing certain preset features and characteristics, matchmaking efforts ensure that seemingly unrelated pieces of data can be linked together in a meaningful way, making data interpretation and analysis more effective.
Data Cleaning
Then, the data cleaning process tames the chaos that comes with massive datasets. It involves removing duplicates, filling in missing values, correcting errors, and standardising different formats to ensure consistency. By 'cleaning' the data, ChatGPT-4 ensures the precision and accuracy of its language predictions.
Data Indexing
Data Indexing, meanwhile, streamlines data retrieval. Imagine a massive library without indexing; finding a single book would be a nightmare. Data indexing works similarly, turning data into a well-organised library where each 'book' or informative piece of data can be easily and quickly located.
Data Linking
Data Linking strategically creates connections between relevant datasets. Linked data enriches the context by providing comprehensive and related information. This technique greatly enhances the conversational capabilities and contextual understanding of ChatGPT-4.
Furthermore, ChatGPT-4 deploys machine learning algorithms for reorganisation to learn and improve from its interactions, making it more efficient with time. This way, reorganisation is not just data management, but active learning as well.
Conclusion
Reorganisation is a powerful tool in managing the surfeit of data that the digital era produces. By structuring and organising complex data structures, it facilitates user interaction, improves data integrity, and advances system performance. More innovatively, it breathes life into systems like ChatGPT-4, providing them the ability to handle, interpret, and learn from data in the most efficient way. As technology continues to evolve, reorganisation techniques will only grow more sophisticated and integral to managing data in the digital age.
Comments:
Thank you for reading my article on revolutionizing the reorganization with ChatGPT! I'd love to hear your thoughts and opinions.
Great article, Lisa! ChatGPT has definitely revolutionized the way we approach technology. It's exciting to see AI evolving at such a rapid pace.
I agree, Mark. The advancements in AI like ChatGPT are astounding. However, we should also consider the ethical implications of such powerful technology.
I couldn't agree more, Emily. As much as AI is beneficial, we need to ensure it doesn't harm us in any way. Ethical guidelines must be in place.
Great points, Mark and Emily. AI's potential is immense, but without proper regulations, it could lead to significant challenges. We should tread carefully.
Thank you, Mark, Emily, Sarah, and Michael, for your insightful comments. Ethics and regulations are indeed crucial when it comes to AI. Let's discuss further.
I enjoyed reading your article, Lisa. ChatGPT has undoubtedly transformed technology. It's fascinating to witness AI's impact across various sectors.
I completely agree, Alexandra. The versatility of ChatGPT is impressive. It has the potential to revolutionize customer service and support systems too.
Absolutely, Daniel. With ChatGPT, businesses can offer personalized and efficient support to their customers, enhancing the overall experience.
I can see ChatGPT's value in providing real-time assistance and interactive conversations. It could significantly improve customer satisfaction and engagement.
Thank you, Alexandra, Daniel, Olivia, and Hannah. I appreciate your positive feedback. Indeed, ChatGPT holds immense potential for customer service applications.
Lisa, your article was enlightening. ChatGPT's ability to generate human-like text is truly remarkable. It can revolutionize content creation and copywriting too!
Absolutely, Peter! ChatGPT can streamline and enhance content production, making it easier for writers and marketers to create engaging material.
You're spot on, Peter and Sophia. The applications of ChatGPT in content creation are vast. It's exciting to witness its impact on various domains.
Lisa, your article highlighted the incredible potential of ChatGPT in transforming technology. It's an exciting time for AI advancements.
I couldn't agree more, Jason. The possibilities AI offers are limitless, and ChatGPT is a significant step towards more interactive and intelligent systems.
Thank you, Jason and Natalie, for your positive feedback. AI's potential is indeed limitless, and ChatGPT is making waves in the industry.
Lisa, your article shed light on the transformative power of ChatGPT. However, we must also be cognizant of potential misuse and biases in AI systems.
Well said, Benjamin. AI systems like ChatGPT should undergo thorough testing and evaluation to eliminate biases that may perpetuate social inequalities.
I appreciate your input, Benjamin and Sophie. Addressing biases and ensuring fairness in AI systems is a critical aspect that needs constant attention.
Lisa, your article on ChatGPT's impact was insightful. However, what steps can individuals take to adapt to these evolving technologies?
That's an intriguing question, Christopher. Learning and upskilling ourselves to better understand and leverage AI can help us adapt and succeed in this changing landscape.
Christopher and Ethan, you raise an important point. Continuous learning and embracing technological advancements can empower individuals to navigate the evolving landscape with ease.
Lisa, your article showcased the transformative potential of ChatGPT. AI advancements like this open up exciting opportunities for innovation across industries.
Absolutely, Patrick. ChatGPT has the potential to fuel innovation and create new possibilities in every sector, leading to unprecedented growth.
Thank you, Patrick and Emma, for your valuable feedback. Indeed, ChatGPT paves the way for innovation and growth in various sectors.
Lisa, your article provided a comprehensive overview of how ChatGPT revolutionizes technology. It's fascinating to witness the AI advancements in action.
I couldn't agree more, David. The exponential progress of AI, as exemplified by ChatGPT, is reshaping the technological landscape.
David and Melissa, thank you for your kind words. AI, especially ChatGPT, is indeed remolding technology as we know it.
Lisa, your article offered valuable insights into the impact of ChatGPT. How do you foresee its integration into our daily lives in the future?
That's a thought-provoking question, Andrea. With AI becoming increasingly widespread, ChatGPT could become an integral part of our daily interactions, assisting us in various tasks.
Andrea and James, your question raises an exciting prospect. As AI technologies advance further, ChatGPT has the potential to become a ubiquitous presence in our daily lives, providing assistance and enriching experiences.
Lisa, your article shed light on ChatGPT's transformative capabilities. How do you envision its impact on education and learning?
That's an excellent question, Sophia. AI technologies like ChatGPT can revolutionize education, offering personalized learning experiences and assisting students and teachers alike.
Sophia and Oliver, a great point indeed. ChatGPT's integration into education holds promise for personalized learning and enhanced educational outcomes, facilitating student growth.
I thoroughly enjoyed your article, Lisa. ChatGPT's impact on communication and language understanding is significant. How do you see it evolving further?
That's an interesting question, Sarah. As AI evolves, I believe ChatGPT will become even more versatile, grasping complex contexts and offering nuanced responses.
Thank you, Sarah and Nathan. I envision ChatGPT evolving into a highly contextual and adaptable AI, providing seamless communications across various domains.
Lisa, your article highlighted the transformative potential of ChatGPT in various sectors. As AI advances, how can we address privacy concerns?
An essential consideration, Emma. Stakeholders must prioritize privacy, data security, and open dialogues to build trust and protect users' personal information.
Emma and Matthew, you raise a vital point. To address privacy concerns, regulations, transparency, and responsible practices should be at the forefront of AI development and deployment.
Great article, Lisa! AI like ChatGPT holds immense potential in transforming the way we interact with technology. Exciting times ahead!
Lisa, your article was a fascinating read! ChatGPT is truly revolutionizing the technology landscape. It's incredible how far AI has come.
Lisa, your article provided an excellent overview of ChatGPT's impact. It's amazing to witness the advancements in AI and their real-world applications.
Lisa, I found your article thought-provoking. AI advancements like ChatGPT offer endless possibilities in streamlining various processes and systems.
Great insights, Lisa! ChatGPT's potential to transform technology is incredible. I look forward to seeing how it evolves in the future.
Lisa, your article shed light on the incredible potential of ChatGPT. It's exciting to imagine the possibilities it brings to technology and beyond.
Lisa, your article captured the essence of ChatGPT's transformative power. It's exciting to think about the future possibilities this technology unlocks.
Great job, Lisa! The advancements in AI like ChatGPT are remarkable. They have the potential to revolutionize multiple industries.
Lisa, your article was a compelling exploration of ChatGPT. It's fascinating to witness the impact of AI technology on our lives.
Fantastic article, Lisa! AI innovations like ChatGPT are reshaping technology, opening up possibilities across various fields.
Lisa, your article provided valuable insights into the impact of ChatGPT. It's exhilarating to see the growth of AI and its remarkable potential.
Lisa, your article was a great read! AI like ChatGPT has the potential to transform industries, making technology more accessible and impactful.
Great insights, Lisa! ChatGPT's advancements usher in a new era of technology. Exciting times lie ahead.
Lisa, your article painted a vibrant picture of ChatGPT's potential. The possibilities are endless, and it will be fascinating to see how it unfolds.
Fantastic article, Lisa! AI innovations like ChatGPT will redefine the way we perceive and interact with technology.
Lisa, your article provided valuable insights into ChatGPT's transformative capabilities. The advancements in AI continue to amaze and inspire.
Great job, Lisa! ChatGPT's ability to revolutionize technology is astounding. It's incredible to witness AI impact our lives.
Lisa, your article was a captivating exploration of ChatGPT's potential. AI advancements continue to push the boundaries of what's possible.
Fantastic insights, Lisa! ChatGPT is truly revolutionizing technology, with far-reaching implications across sectors.
Lisa, your article eloquently presented the transformative capacity of ChatGPT. It's fascinating how AI is becoming an integral part of our lives.
Great article, Lisa! ChatGPT's potential to reshape technology is exceptional. The future looks promising.
Lisa, your article brought to light the immense potential of ChatGPT. It's exciting to consider its implications across various domains.
Lisa, your article was an engaging discussion on ChatGPT's transformative capabilities. I'm thrilled to witness the progress of AI.
Fantastic insights, Lisa! ChatGPT's evolution in technology has the potential to redefine the way we approach daily tasks.
Lisa, your article offered an excellent exploration of ChatGPT's transformative power. AI offers boundless possibilities.
Great job, Lisa! ChatGPT's advancements open doors to unimaginable possibilities. The technological landscape is evolving rapidly.
Lisa, your article highlighted the immense potential of ChatGPT. It's encouraging to see how AI is shaping our future.
Fantastic article, Lisa! ChatGPT's transformative capabilities are truly remarkable. It's exciting to be witness to this technological revolution.
Lisa, your article provided valuable insights into the revolutionary nature of ChatGPT. The rapid advancement of AI is awe-inspiring.
Great insights, Lisa! ChatGPT's potential to transform technology is immense. I can't wait to see how it shapes the future.
Lisa, your article skillfully portrayed ChatGPT's transformative impact. AI advancements continue to be fascinating and game-changing.
Fantastic article, Lisa! ChatGPT's potential to reshape technology is astounding. The possibilities are endless.
Lisa, your article was a thought-provoking exploration of ChatGPT's transformative capabilities. AI's progress is incredible.
Great job, Lisa! ChatGPT's ability to revolutionize technology is fascinating. AI's impact is undeniable.
Lisa, your article eloquently discussed ChatGPT's transformative potential. It's exciting to witness AI's rapid evolution.
Fantastic insights, Lisa! ChatGPT's transformative capabilities have endless implications for technology and beyond.
Great article, Lisa! ChatGPT's impact on technology is immense. AI continues to reshape our world.
Lisa, your article showcased the transformative potential of ChatGPT. It's incredible to see the progress AI has made.
Fantastic job, Lisa! ChatGPT's transformative capabilities are awe-inspiring. Technology is evolving rapidly.
Lisa, your article offered valuable insights into ChatGPT's transformative potential. AI continues to amaze and inspire.
Great insights, Lisa! ChatGPT's potential to revolutionize technology is remarkable. The future looks bright.
Lisa, your article provided a comprehensive overview of ChatGPT's impact. AI's potential is boundless.
Fantastic article, Lisa! ChatGPT's transformative capabilities are remarkable. It's an exciting time for AI.
Lisa, your article highlighted the transformative potential of ChatGPT. AI advancements continue to astound.
Great job, Lisa! ChatGPT's ability to revolutionize technology is incredible. It's an exciting time for AI.
Lisa, your article provided interesting insights into ChatGPT's transformative power. AI's growth is truly remarkable.
Fantastic article, Lisa! ChatGPT's transformative capabilities are revolutionizing technology. AI's progress is astonishing.
Lisa, your article portrayed ChatGPT's transformative potential effectively. AI's impact knows no bounds.
Great insights, Lisa! ChatGPT's potential to transform technology is unrivaled. The future looks promising.
Lisa, your article offered valuable insights into ChatGPT's transformative power. The possibilities seem endless.
Fantastic job, Lisa! ChatGPT's transformative capabilities are awe-inspiring. AI's growth is extraordinary.
Lisa, your article skillfully approached ChatGPT's transformative potential. AI's progress is remarkable.
Great article, Lisa! ChatGPT's ability to revolutionize technology is awe-inspiring. AI continues to reshape our world.
Lisa, your article provided an excellent exploration of ChatGPT's transformative capabilities. The progress of AI is exceptional.
Fantastic insights, Lisa! ChatGPT holds immense potential in reshaping technology. AI's rapid growth is fascinating.
Great job, Lisa! ChatGPT's transformative capabilities are remarkable. The future of AI looks bright.
Lisa, your article eloquently presented ChatGPT's transformative potential. The progress of AI is awe-inspiring.
Fantastic article, Lisa! ChatGPT's transformative capabilities are awe-inspiring. AI's impact is remarkable.
Lisa, your article provided valuable insights into ChatGPT's transformative power. The possibilities seem endless.
Thank you all for taking the time to read my article on ChatGPT and join in the discussion. I'm excited to hear your thoughts and opinions!
Great article, Lisa! ChatGPT definitely seems like a game-changer in the field. The potential applications for natural language processing are endless.
I completely agree, Alex! ChatGPT has the ability to revolutionize how we interact with technology, making it more intuitive and user-friendly.
While ChatGPT certainly has its advantages, I am concerned about the potential risks associated with AI-powered systems like this. Can we ensure data privacy and avoid bias?
Valid points, David. Data privacy and bias are indeed important considerations. The developers are actively working to mitigate these issues and ensure transparency. However, it's a continuous process that requires collaboration and vigilance from all stakeholders.
I think one of the most exciting aspects of ChatGPT is its potential impact on customer service. It could greatly improve the efficiency and effectiveness of chatbots, enhancing the customer experience.
Absolutely, Sophia! ChatGPT has the ability to understand and respond to natural language queries, leading to more personalized and satisfying customer support interactions.
I can see ChatGPT being extremely useful in the education sector. It could provide personalized tutoring, help with language learning, and even assist students in understanding complex concepts.
You're absolutely right, Sarah! ChatGPT has immense potential in education, offering adaptive learning experiences and personalized assistance to students, thereby improving educational outcomes.
I have to say, though, sometimes ChatGPT still produces inaccurate or nonsensical answers. It's impressive, but there's definitely room for improvement. Are there any plans for further enhancements?
Indeed, Daniel, continuous improvement is a key focus. OpenAI is actively seeking feedback from users to identify areas of improvement and address the limitations of ChatGPT. Your input would be invaluable!
Glad to hear that improvements are actively being made, Lisa. I look forward to seeing ChatGPT become even more reliable and accurate over time.
Thank you, Daniel! OpenAI's commitment to continuous improvement ensures that ChatGPT will evolve and address the concerns raised by users like yourself.
I believe competition is important in driving innovation. Are there any other similar AI models in development that could pose a challenge to ChatGPT?
Absolutely, Alex! There are several other AI models, both developed by OpenAI and external researchers, that are challenging ChatGPT's capabilities. This healthy competition encourages further advancements in the field.
Competition drives progress, as you mentioned, Lisa. I believe this constant drive to outperform each other will lead to more robust and capable AI systems.
Absolutely, Alex! The AI community's collaborative efforts and healthy competition contribute to the advancement of AI systems, benefiting society as a whole.
Lisa, do you foresee any challenges in training ChatGPT in multiple languages? Language nuances and cultural differences can be complex.
You raise an important point, Alex. Training ChatGPT in multiple languages does present challenges. However, OpenAI is actively working on expanding language support to make it more inclusive and reliable.
Expanding ChatGPT's language support will definitely make it more accessible to a wider range of users, Lisa. I'm glad OpenAI is actively working on that.
Absolutely, Alex. OpenAI's dedication to expanding language support will ensure a more inclusive and global reach for ChatGPT, enabling more users to benefit from its capabilities.
ChatGPT could be a game-changer for individuals with disabilities. It could assist those with speech impairments in communicating more effectively.
That's a great point, Samuel! ChatGPT's potential to aid people with disabilities is incredible. It could provide them with a more independent and inclusive means of communication.
I hadn't thought about the impact on individuals with speech impairments, Samuel. ChatGPT's potential to assist them is truly remarkable and can make a real difference in their lives.
Absolutely, Emily. The potential for ChatGPT to bridge communication gaps for individuals with speech impairments is truly groundbreaking.
I wonder how ChatGPT would fare with more complex tasks, such as programming or scientific research.
That's an interesting thought, Oliver! While ChatGPT is designed with versatility in mind, it may require further fine-tuning and specialization to tackle those complex domains effectively.
Oliver, while ChatGPT might not be an expert in programming or scientific research yet, it can certainly provide useful insights and assist in understanding concepts in those fields.
That's a fair point, Sophia. Starting with providing assistance and insights could be a stepping stone towards more specialized AI models for programming or scientific research.
Oliver, what if AI systems like ChatGPT become advanced enough to automatically generate code snippets or conduct basic scientific research? The possibilities are exciting!
Indeed, Sophia, the prospect of AI systems assisting in code generation or contributing to scientific research is incredibly exciting. It could accelerate progress in those areas.
As an educator, I'm cautiously optimistic about ChatGPT's role in education. While it could be a valuable tool, we should ensure it complements human teaching instead of replacing it entirely.
I agree, Jennifer. The human touch is irreplaceable in education. ChatGPT should be seen as a powerful supplement that can enhance the learning experience, not replace teachers.
Are there any particular ethical considerations that need to be taken into account while developing and deploying AI models like ChatGPT? How do we prevent misuse?
Ethical considerations are paramount, David. OpenAI is committed to responsible AI development and deployment. They're actively working on addressing biases, transparency, and ensuring guidelines for responsible use.
Lisa, what steps can be taken to educate users about the limitations of ChatGPT and prevent them from relying on potentially inaccurate or biased information?
That's a crucial point, David. OpenAI recognizes the importance of user education and is actively exploring methods to highlight ChatGPT's limitations, promoting responsible and informed usage.
That's reassuring to hear, Lisa. User education will be key in ensuring ChatGPT is used as a helpful tool without relying solely on its responses.
With such advances in AI, it's crucial to establish clear regulations and guidelines to ensure the responsible and ethical use of these models.
I couldn't agree more, Daniel. As AI becomes more prevalent, establishing ethical frameworks and regulations will safeguard against potential misuse and ensure the technology benefits society.
User education is crucial, Lisa. Providing clear disclaimers about the limitations of ChatGPT and promoting critical thinking can help users make informed decisions about its responses.
Absolutely, Daniel. OpenAI is actively working on developing mechanisms to provide users with transparent information about ChatGPT's capabilities and limitations, encouraging responsible usage.
I think it's important to ensure that ChatGPT is accessible to all students, regardless of their socioeconomic background, to avoid exacerbating educational inequalities.
Absolutely, Jennifer. Promoting equitable access to AI technologies like ChatGPT is crucial. Efforts are being made to address accessibility barriers and include diverse perspectives during development.
Competition pushes technology forward, and I believe ChatGPT's capabilities will continue to grow as researchers develop more advanced AI models.
You're absolutely right, Sarah. The ongoing development of AI models and the competition among researchers are driving the field towards greater advancements and more capable systems.
The challenges in training ChatGPT in multiple languages are indeed complex, Lisa. It requires a deep understanding of different languages and cultural contexts to ensure accurate and unbiased responses.
You're absolutely right, Emily. OpenAI is actively working on refining language models to account for nuances and cultural sensitivities, ensuring ChatGPT performs well across different languages.
It's fascinating to witness the advancements in AI technology, and I can't wait to see how ChatGPT and its successors shape the future.
Indeed, Sarah! The future of AI holds immense potential, and as ChatGPT continues to improve and evolve, it will play a significant role in shaping the way we interact with technology.