Transforming Data Segmentation in Catalogs Technology: A Comprehensive Guide to Leveraging ChatGPT
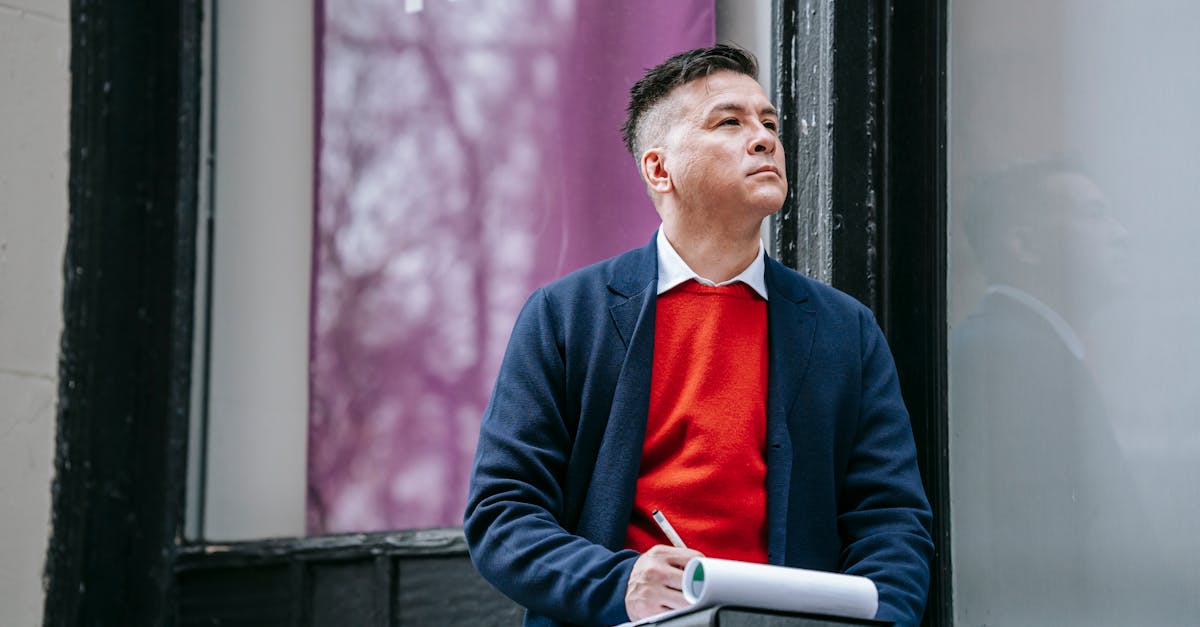
Introduction
In today's competitive business landscape, marketers strive to optimize their marketing strategies by reaching the right customers with personalized messages. Data segmentation plays a crucial role in achieving this goal, by dividing customer data into distinct groups based on specific criteria. Catalogs, as a technology and tool, enable effective data segmentation for targeted marketing strategies.
What are Catalogs?
Catalogs refer to a systematic organization and classification of customer data, allowing businesses to efficiently manage and analyze information for marketing purposes. This technology helps create a comprehensive overview of customer profiles, including their demographics, preferences, purchase history, and behavior patterns.
The Role of Data Segmentation
Data segmentation involves dividing customer data into meaningful subsets based on shared characteristics or behavior. Businesses can identify unique segments within their customer base, which enables the development of targeted marketing campaigns. By understanding the specific preferences and needs of different customer segments, marketers can tailor their messages accordingly, resulting in higher engagement rates and conversion rates.
Benefits of Catalogs for Data Segmentation
- Improved targeting: Catalogs allow businesses to identify segments of customers that are most likely to be interested in a particular product or service. This enables marketers to create personalized offers and promotions, resulting in a higher likelihood of conversion.
- Efficient resource allocation: By segmenting customer data, businesses can allocate their marketing resources more effectively. They can focus their efforts on the segments that yield the highest returns, rather than targeting a broad audience with generic messages.
- Enhanced customer experience: Personalized marketing messages based on data segmentation create a more relevant and engaging experience for customers. This can foster a sense of loyalty and improve customer satisfaction.
- Increased campaign effectiveness: Targeted marketing strategies driven by data segmentation are more likely to resonate with customers, leading to higher response rates and improved campaign effectiveness.
- Better understanding of customer behavior: Catalogs provide valuable insights into customer behavior patterns and preferences. Marketers can analyze these patterns to refine their marketing strategies and make data-driven decisions.
Usage in Targeted Marketing Strategies
Catalogs are indispensable for executing targeted marketing strategies. Here are some common applications:
- Segment-specific promotions: By analyzing customer data segmented by catalogs, marketers can design targeted promotions for specific segments. For example, if a clothing retailer identifies a segment of customers interested in sports apparel, they can create personalized offers and discounts for that specific segment.
- Personalized email campaigns: Catalogs allow businesses to tailor email campaigns based on customer preferences, demographics, and past behavior. Personalized emails have higher open rates and click-through rates compared to generic email blasts.
- Dynamic website content: Utilizing catalog data segmentation, businesses can customize website content to display products or offers that are relevant to specific customer segments. This enhances the user experience and increases the likelihood of conversion.
- Customized recommendations: E-commerce businesses can leverage catalogs to deliver personalized product recommendations to customers based on their past purchase history or browsing behavior.
Conclusion
Catalogs provide businesses with the necessary tools to segment customer data effectively, enabling targeted marketing strategies. By understanding and leveraging valuable insights obtained through data segmentation, businesses can craft personalized messages and offers, resulting in improved customer engagement, conversion rates, and overall marketing effectiveness.
Comments:
Thank you all for taking the time to read my article on transforming data segmentation in catalogs technology. I hope you found it insightful. I'm here to answer any questions you may have!
Great article! It provides a clear and comprehensive guide on leveraging ChatGPT for transforming data segmentation in catalogs technology. I especially appreciate the practical examples provided.
Thank you, Alexandra! I'm glad you found the practical examples helpful. Is there any specific aspect you would like me to elaborate on?
I've recently started using ChatGPT for data segmentation in my catalogs technology project. This article came at the perfect time! It clarified several doubts I had and provided useful tips.
That's great to hear, Susan! If you have any specific questions related to your project or need further guidance, feel free to ask.
I found the section on data preprocessing quite insightful. It emphasized the importance of cleaning and organizing the data before applying ChatGPT for data segmentation. Thanks for the valuable information!
You're welcome, James! Data preprocessing is indeed a crucial step to ensure optimal results. If you have any questions regarding the data preprocessing techniques mentioned in the article, feel free to ask.
The article mentions that ChatGPT can handle different types of data. Does it work equally well with structured and unstructured data in catalogs?
Good question, Nadia! While ChatGPT primarily excels with unstructured data in natural language form, it can also handle structured data effectively. Depending on the specific use case, appropriate preprocessing and data formatting might be required.
This article opened my eyes to the potential of leveraging ChatGPT for data segmentation in catalogs. I hadn't considered this approach before, but now I'm excited to explore it further!
I'm glad you found it inspiring, Hannah! If you need any further information or have specific queries while exploring this approach, feel free to ask.
What are the main limitations or challenges one might encounter while applying ChatGPT for data segmentation in catalogs? Are there any particular ways to mitigate these challenges?
Great question, Daniel! Some limitations of ChatGPT include generating incorrect or nonsensical outputs, sensitivity to input phrasing, and a tendency to be verbose. To mitigate these, one can fine-tune the model, add context prompts, use human reviewers, and have a feedback loop for continuous improvement.
Is ChatGPT suitable for real-time data segmentation in catalogs, or is it mainly for batch processing?
Good question, Sophia! While ChatGPT can be used for real-time data segmentation, it's important to consider the computational resources required based on the frequency and volume of real-time requests. For lower latency requirements, batching can be a more efficient approach.
The article mentions using domain-specific prompts for better results. Could you provide any examples of effective prompts for data segmentation in catalogs?
Certainly, Emily! Effective prompts can be tailored to your specific catalog and data segmentation needs, such as 'Segment products based on customer preferences' or 'Classify catalog items into relevant categories.' It's important to experiment and refine prompts based on the desired segmentation goals.
I noticed in the article that ChatGPT can be fine-tuned on custom datasets. Are there any recommendations or best practices for fine-tuning the model for data segmentation in catalogs?
Good question, Jacob! When fine-tuning ChatGPT, it's important to balance between overfitting and generalization. Having an appropriate mix of domain-specific and more general training data can help achieve better results. Additionally, using a validation set, experimenting with hyperparameters, and iteration cycles are recommended practices.
The article mentions using the 'temperature' parameter to control the randomness of generated outputs. Are there any specific temperature ranges commonly used for data segmentation in catalogs?
Great question, Oliver! The 'temperature' parameter can be adjusted to increase or decrease randomness. A higher temperature (e.g., 0.7-1.0) leads to more diverse but potentially less focused outputs. A lower temperature (e.g., 0.2-0.5) produces more deterministic and focused outputs. The choice depends on the desired segmentation context and specific use case.
Are there any ethical considerations to keep in mind while leveraging ChatGPT for data segmentation in catalogs?
Ethical considerations are crucial when using AI technologies. It's important to ensure the data used for training doesn't introduce biases. Monitoring and reviewing the generated outputs, maintaining a feedback loop with human reviewers, and addressing any biases or ethical concerns that arise are essential steps to safeguard against ethical issues.
How does ChatGPT handle multilingual catalogs? Can it effectively segment catalogs in different languages?
Good question, Grace! ChatGPT can process and generate text in multiple languages, but it's essential to fine-tune or train the model on diverse multilingual data for better performance with specific languages. It's also helpful to provide appropriate language context prompts to guide the segmentation process.
The article mentions using ChatGPT for clustering catalog items. How does ChatGPT compare to traditional clustering algorithms in terms of performance and accuracy?
Good question, Andrew! ChatGPT can provide an alternative approach to clustering catalog items compared to traditional algorithms. Its performance and accuracy may vary depending on the dataset and specific clustering requirements. It's recommended to evaluate different methodologies and compare results before making a final decision.
Is ChatGPT suitable for small-scale catalogs with limited data?
Absolutely, Isabella! ChatGPT can be suitable for small-scale catalogs with limited data. However, it's important to fine-tune the model on a relevant subset of your data to improve its understanding and segmentation capabilities. Iteratively expanding the fine-tuning with additional data can also be beneficial.
How can we measure the success of ChatGPT's segmentation performance in catalogs?
Measuring the success of ChatGPT's segmentation performance can vary depending on the specific goals. Some common metrics include precision, recall, F1 score, and clustering evaluation metrics like silhouette coefficient or normalized mutual information. It's important to define relevant evaluation criteria based on the desired outcomes for your catalog segmentation.
Can ChatGPT handle noisy or incomplete data while segmenting catalogs? Or does it require clean and complete data?
Good question, Emma! While ChatGPT can handle some amount of noisy or incomplete data, it generally works better with clean and complete data. Preprocessing and data cleaning techniques, such as removing irrelevant noise and filling missing values, can improve its performance with noisy or incomplete catalog data.
What are the typical use cases where ChatGPT can significantly improve data segmentation in catalogs?
Good question, William! ChatGPT can significantly improve data segmentation in catalogs for use cases like categorizing products, generating relevant attributes for listings, clustering similar items, and automating the segmentation process based on customer feedback or preferences. Its language understanding and generation capabilities make it a versatile tool for catalog segmentation.
Is it possible to integrate ChatGPT with existing catalog management systems? How can the generated segmentation results be incorporated back?
Integration with existing catalog management systems is definitely possible, Sophie! The generated segmentation results can be incorporated back by using APIs or developing custom connectors to feed the results into the catalog management system. It's important to design the integration flow ensuring compatibility and reliability between ChatGPT and the system.
Are there any potential privacy concerns when using ChatGPT for data segmentation in catalogs?
Privacy concerns should be taken into account, Ella. When using ChatGPT, it's crucial to handle sensitive customer information carefully, ensure secure data handling practices, and consider privacy regulations. Implementing appropriate data anonymization techniques and reviewing the generated outputs can help mitigate potential privacy risks and maintain compliance.
Can ChatGPT handle large-scale catalogs with millions of items?
Certainly, Lucas! ChatGPT can handle large-scale catalogs with millions of items. However, it's important to consider computational resources and optimize the segmentation process for efficiency at that scale. Techniques like batching, distributed processing, or utilizing hardware accelerators can be useful.
Are there any limitations or challenges in terms of language understanding and accuracy when using ChatGPT for catalog data segmentation in languages other than English?
Language understanding and accuracy can be more challenging with languages other than English, Olivia. While ChatGPT supports multiple languages, the quality of segmentation results may depend on the availability of training data and fine-tuning in the specific language. It's important to assess the results in different languages and iterate to improve accuracy.
In your experience, what are some of the most exciting applications of ChatGPT in catalog technology?
Great question, Emma! Some of the most exciting applications of ChatGPT in catalog technology include automating product attribute generation, enhancing search and recommendation systems, automating product categorization, and enabling personalized shopping experiences. The combination of language understanding and generation capabilities provides a powerful tool for various catalog-related tasks.
Can ChatGPT be used to analyze customer sentiment or preferences from catalog data as part of segmentation?
Absolutely, Oliver! ChatGPT can be utilized to analyze customer sentiment or preferences from catalog data by generating relevant insights based on the provided prompts. By fine-tuning the model with sentiment-specific data or using context prompts related to customer preferences, it can effectively capture and segment sentiment or preference-related information from the catalog.
What are the recommended hardware requirements to leverage ChatGPT for data segmentation in catalogs efficiently?
The hardware requirements for efficiently leveraging ChatGPT depend on factors like the dataset size, desired response times, and computational resources available. GPU acceleration is usually recommended for improved performance, and larger memory capacity helps handle larger-scale datasets. It's a good practice to consider reputable cloud platforms or dedicated hardware for optimal results based on your specific requirements.
Thank you, everyone, for your valuable questions and comments! I hope this discussion has been informative and insightful for all of you. If you have any more queries or need further assistance, I'm here to help!