Unleashing the Power of Gemini: Revolutionizing Probability Theory in Technology
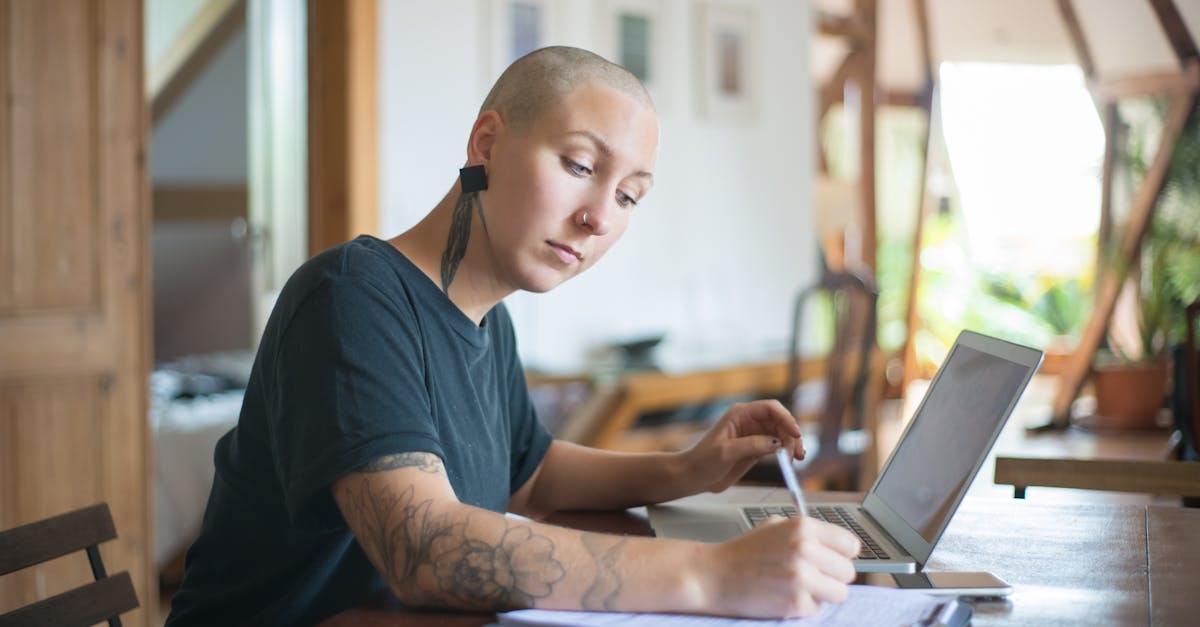
Probability theory has long been a foundational concept in various fields, particularly in technology. It helps us make informed decisions, predict outcomes, and understand uncertainties. With recent advancements in technology, the rise of artificial intelligence (AI) has opened up new possibilities for how probability theory is applied and utilized.
Introducing Gemini
One of the most remarkable AI models that has significantly influenced this field is Gemini. Developed by Google, Gemini is a language model based on the transformer architecture. It has demonstrated impressive capabilities in generating coherent, context-aware text based on given prompts.
Gemini is trained using a diverse range of data sources, which allows it to understand a wide array of topics and provide relevant responses. Its ability to generate human-like text has made it a valuable tool in several applications such as chatbots, customer support systems, and content generation.
Revolutionizing Probability Theory
So, how does Gemini revolutionize probability theory in technology? By leveraging its language generation capabilities, it can aid in various probabilistic tasks, including:
- Data Generation: Gemini can generate synthetic data based on given probability distributions. This can be immensely helpful in scenarios where real data is limited or expensive to obtain. Researchers and practitioners can use this feature to simulate data and perform analyses without relying solely on existing datasets.
- Hypothesis Testing: Gemini can be trained on historical data to capture real-world patterns and generate hypotheses for future events. This can assist in decision-making processes and help organizations assess potential risks or opportunities based on probabilistic predictions.
- Scenario Planning: By interacting with Gemini, individuals or teams can generate multiple scenarios and explore their potential outcomes. This can aid in strategic planning, risk assessment, and resource allocation, allowing organizations to make more informed decisions.
- Uncertainty Quantification: Gemini can help quantify uncertainties by generating probabilistic forecasts. It can predict the likelihood of specific events or outcomes, allowing organizations to adapt and plan accordingly.
Limitations and Challenges
While Gemini and similar language models have immense potential in revolutionizing probability theory, it is essential to acknowledge their limitations and challenges. Some of the key concerns include:
- Bias and Fairness: Language models can amplify biases present in the training data. Developers must be cautious in training Gemini on diverse datasets to minimize bias, ensuring fair and ethical usage.
- Interpretability: The inner workings of language models like Gemini are not easily interpretable. Understanding the model's decision-making process can be challenging, making it important to approach its outputs with caution.
- Data Privacy: Given that language models learn from vast amounts of data, concerns about data privacy and security arise. Safeguarding user information and complying with data protection regulations is crucial.
The Future of Probability Theory
As language models continue to evolve, incorporating them into probability theory opens up exciting possibilities. Probabilistic tasks can benefit from the sophisticated capabilities of models like Gemini, allowing practitioners to gain deeper insights, drive innovation, and make data-driven decisions.
However, it is vital to consider the ethical ramifications and potential risks associated with the use of AI-based models. Striking a balance between technological advancements and responsible implementation is key to unlocking the true potential of probability theory in the future.
Comments:
Thank you all for taking the time to read my article on Unleashing the Power of Gemini! I'm excited to discuss the potential revolution of probability theory in technology.
Great article, Jon! Gemini has indeed shown immense potential. I believe it can greatly improve various aspects of technology, especially in fields like natural language processing and virtual assistance.
Ryan, I'm particularly interested in the impact of Gemini on virtual assistants like Siri or Alexa. It can potentially enhance their conversational abilities and make them more natural and intuitive to use.
I agree, Ryan! Gemini can be a game-changer. The ability to generate human-like responses opens up exciting possibilities for chatbots, customer support systems, and even content creation. I'm curious to see how it evolves!
Absolutely, Sara! Gemini has already shown impressive improvements in generating more coherent and contextually relevant responses. It will be interesting to see how it handles complex queries and multi-turn conversations.
While Gemini shows great promise, we must also be cautious about potential ethical concerns. The output heavily relies on the training data it received, which can introduce biases and misinformation.
You're right, Emily. Bias in AI models is a crucial issue. As developers, we need to ensure diverse and representative training data to avoid perpetuating harmful stereotypes or spreading false information.
Jonas, you're spot on! Researching and addressing biases in AI systems should be an ongoing effort. Transparent evaluation frameworks can also help identify and mitigate potential issues.
Isabella, I completely agree with you. Ensuring evaluation frameworks are transparent and comprehensive will be instrumental in understanding and improving the capabilities and limitations of Gemini.
Harper, you're right. Transparent evaluation frameworks and active involvement from the AI community will help drive responsible and ethical AI development moving forward.
I completely agree, Jonas. Ethical considerations should be at the forefront of AI development. Bias, privacy, and potential misuse need to be effectively addressed to harness Gemini's power responsibly.
I'm excited about Gemini's applications in education. It could provide personalized and adaptive learning experiences. Imagine having an AI tutor that understands each student's needs and tailors lessons accordingly!
That's a great point, Amy. Educational technology has come a long way, but Gemini can take it to the next level. It has the potential to enhance both collaborative and individual learning experiences.
Amy, an AI tutor with the power of Gemini could definitely revolutionize personalized learning experiences. It may help overcome some of the challenges faced in traditional classrooms too.
Considering the widespread use of Gemini, we should ensure it complies with data protection regulations. User privacy and consent are of utmost importance, especially when handling sensitive information.
Sophia, you're absolutely right. Data protection regulations vary across regions, and it's crucial to ensure compliance while incorporating AI technologies like Gemini into various products and services.
Exactly, Joshua. Adhering to regulations and ensuring ethical practices will earn users' trust and lead to broader adoption of AI-powered technologies like Gemini.
I appreciate all your insightful comments! It's significant to be mindful of potential challenges and ethical considerations while leveraging Gemini. Responsible and careful implementation is key to its success.
Indeed, Jon. We must proceed with caution to address any challenges that arise. Transparent communication with users about the AI capabilities and limitations is vital to manage expectations.
Sara, managing user expectations is crucial, especially when it comes to AI's limitations. Transparent communication can help prevent users from assuming AI can perform tasks beyond its capabilities.
Chris, agreed! AI, whether it's Gemini or other models, should be seen as a tool that complements human abilities and enhances efficiency, not as a complete replacement.
Gemini's potential in content creation is unparalleled. It could generate blog articles, social media content, and even creative writing. However, humans should still be involved in the editing process to maintain originality and quality.
Mia, you're absolutely right. AI can assist in content creation, but human creativity, perspective, and critical thinking are still essential in maintaining the quality and originality of the content.
I love the idea of an AI companion powered by Gemini. It could provide emotional support, engage in meaningful conversations, and even assist with mental health-related tasks. It could be a real game-changer for those in need.
Emma, that's a fascinating aspect! AI companions utilizing Gemini could have a profound impact on mental health support, making it more accessible and removing some of the barriers people face in seeking help.
While Gemini has incredible potential, we should also be mindful of its limitations. It's essential to be aware of when we're interacting with AI and not humans to avoid any inadvertent misinformation.
Great insights, everyone! Liam, you make an excellent point. Clearly distinguishing AI-generated responses from human input is necessary to prevent any potential issues or confusion.
Jon, you've done a great job highlighting the importance of responsible implementation. AI tools like Gemini should be integrated into systems with checks and balances to ensure they assist rather than deceive.
Absolutely, Emily. Continuous monitoring, feedback loops, and guidelines for proper usage can help prevent unintended negative consequences while leveraging AI technologies.
Jon, your article was thought-provoking! The possibilities Gemini brings to the table are indeed fascinating. It will be interesting to see how it evolves and shapes the future of technology.
Jon, thanks for shedding light on Gemini's power. I'm particularly interested in its potential applications in data analysis and decision-making. It could assist with complex statistical models and predictions.
Oliver, I agree! Gemini's potential in data analysis and predictive modeling is exciting. It can help automate tasks and provide valuable insights, optimizing decision-making processes.
Oliver, feedback loops and guidelines are essential, especially in complex and sensitive areas like AI-powered healthcare. Constant evaluation and improvement should be part of the process to ensure reliability and safety.
The potential impact of Gemini in healthcare is immense. It could help doctors with decision support, medical research, and even facilitate patient communication. However, strict regulations and privacy protocols must be in place.
AI tools like Gemini can greatly augment creativity. It can provide inspiration, ideas, and generate solutions to complex problems. I'm excited to see its potential in various creative domains.
Thank you all for the engaging conversation! I'm glad you found the article thought-provoking. The future of AI, especially with advancements like Gemini, is indeed intriguing and full of possibilities.
Jon, your article makes it clear how Gemini can be a catalyst for innovation across various domains. Thank you for shedding light on its potential and prompting this insightful discussion.
Jon, you've highlighted the significance of responsible implementation with Gemini and other AI technologies. It's essential to mitigate potential risks and align with ethical guidelines at every step.
Absolutely, Jon's article paints an exciting picture of how Gemini can revolutionize probability theory and shape the future of technology. It's crucial to have constructive discussions around its potential challenges and implications.
Indeed, Sophia. Constructive discussions like this one contribute to the responsible development and utilization of AI technologies. I appreciate your active participation!
Thank you all for reading my article! I'm excited to join this discussion about Gemini and its potential impact on probability theory in technology.
Great article, Jon! Gemini indeed has the potential to revolutionize probability theory in technology. The ability to generate human-like responses opens up new possibilities for modeling and prediction. It can greatly enhance decision-making processes.
I agree, Maria. Gemini provides a more intuitive way to explore complex data and understand its underlying patterns. This could be a game-changer for various industries, such as finance and healthcare, where accurate probability assessment is crucial.
While Gemini is impressive, we should also consider the potential risks. It heavily relies on the training data, and if biased or flawed data is used, it might produce incorrect probabilities. Ensuring ethical and fair use is essential.
Valid point, Emily. Bias in training data can certainly be an issue. It's crucial to pay attention to the quality and diversity of the data used for training these models to minimize any potential biases.
I'm excited about Gemini's potential application in natural language understanding tasks. It could help improve text classification algorithms by considering a broader context and generating more accurate probabilities.
Absolutely, Nathan! Gemini can enable better sentiment analysis, intent recognition, and even language translation. The scope of its impact goes beyond just probability theory, making it a valuable tool for NLP researchers.
One concern I have is the potential for Gemini to generate misleading probabilities. It might provide compelling but false probabilities, leading to wrong decisions. How do we address that?
Emma, that's a valid concern. Explainability and interpretability of the generated probabilities are important aspects. Developers need to invest in techniques to understand and explain how Gemini arrives at its probability estimates.
Gemini has a lot of potential, but I wonder about its scalability. Can it handle large datasets efficiently, or would the inference time be too long?
Scalability is indeed an important consideration, Peter. While Gemini has made significant progress, there are still challenges in handling very large datasets effectively. Research in that area is ongoing to make it more efficient.
Gemini could have broad applications in areas like customer support and virtual assistants. The ability to generate detailed, context-aware responses with accurate probabilities could greatly enhance user experiences.
I can see the potential of Gemini in generating more reliable risk assessments for insurance companies. It can process vast amounts of data efficiently and provide insights that traditional methods may miss.
However, we should be cautious about relying solely on Gemini for critical decisions without human oversight. It's important to maintain a balance between automation and human judgment.
Absolutely, Martha. Gemini should be seen as a tool to assist human decision-making, not as a replacement. The combination of AI capabilities and human expertise can lead to the best outcomes.
I'm curious about the underlying methodology of Gemini. How does it handle uncertainty when generating probabilities? Are there any built-in mechanisms for measuring uncertainty?
George, Gemini doesn't have explicit mechanisms for measuring uncertainty. However, techniques like Monte Carlo dropout can be used to estimate uncertainty in predictions and provide confidence intervals.
Gemini's impact on decision-making also raises ethical concerns. Who would be responsible for decisions made based on its probabilities? Are there any guidelines or regulations being developed?
Ethical considerations are paramount, Laura. Guidelines and regulations are evolving to address responsible use of AI technologies like Gemini. Accountability should be shared among developers, organizations, and domain experts.
I'm eager to see the continuous advancements in Gemini. As technology and data improve, its capabilities in probability assessment will only get better. Exciting times ahead!
Thank you, Sophia! I couldn't agree more. The future holds immense potential for Gemini to further revolutionize probability theory and its applications. Let's keep exploring!
Great article! I was amazed by the potential of Gemini in probability theory.
Absolutely! The way Gemini can revolutionize probability theory in technology is mind-blowing.
The insights provided by Gemini in probability theory can definitely lead to breakthroughs in various fields.
I agree! The advancements in technology continue to astound me.
Thank you all for your positive feedback! I'm glad you find the potential of Gemini in probability theory exciting.
I'm curious about how Gemini can be specifically applied to probability theory. Can anyone provide more insights?
Certainly, Frank! One application could be in the analysis of large datasets, allowing for more accurate predictions and decision-making.
In addition to that, Gemini can help in modeling complex systems, aiding in understanding and improving probability estimations.
Another application could be Gemini assisting in risk assessment and management by offering probabilistic analysis for different scenarios.
That sounds fascinating! It's incredible how Gemini can enhance our capabilities in probability theory.
Excellent points, everyone! Gemini is indeed versatile when it comes to probability theory applications.
I'm a data scientist, and I'm impressed by the potential of Gemini in probability theory. It's a game-changer.
Absolutely! As a data scientist myself, I can see many practical applications of Gemini in probability analysis.
That's interesting! Are there any challenges or limitations to consider when utilizing Gemini in probability theory?
Good question, Frank! One challenge is the potential bias that can arise from training data. It's essential to ensure diverse and representative datasets.
I agree with Ethan. Another limitation is the difficulty in understanding the exact reasoning behind Gemini's probabilities, making it important to validate results.
That makes sense. Transparency and interpretability are crucial in any probabilistic analysis.
You bring up valid points, Ethan, Sophie, and Oliver. Addressing bias and ensuring interpretability are important considerations when using Gemini in probability theory.
I wonder whether Gemini has any potential applications in financial risk management.
Definitely, Lily! Gemini can be employed in financial risk management to analyze market trends, assess risk levels, and support decision-making.
That's fascinating! The possibilities seem endless with Gemini's capabilities in probability theory.
I agree! The advancements in AI, like Gemini, open up new horizons in many fields.
Indeed, the potential applications of Gemini in various domains, including financial risk management, are vast.
I'm thrilled to see how Gemini will shape the future of probability theory and technology.
Absolutely! The possibilities are exciting, and I can't wait to see what lies ahead.
Considering the rapid advancements, how do you see Gemini evolving in probability theory in the next few years?
It's hard to predict precisely, but I imagine Gemini will become more sophisticated, capable of handling complex probabilistic models and offering more refined insights.
I think Gemini will also continue to improve on minimizing bias and providing better explanations for its probability estimations.
Indeed, as more research and development go into Gemini, we can expect remarkable advancements in its probabilistic capabilities.
I share your sentiments, Amanda, Frank, and Ethan. Gemini's evolution in probability theory holds tremendous potential for transformative advancements.
Do you think Gemini will ever replace human expertise in probability analysis?
You raise an important point, Lily. Human expertise and AI, like Gemini, can coexist to create more accurate and well-rounded probability analysis.
While Gemini enhances probability analysis, I believe human expertise will remain invaluable. It can complement and collaborate with AI systems, leading to better outcomes.
I agree with Sophie. Human expertise brings contextual understanding and ethical considerations that AI may lack.
Human judgment and decision-making skills will always be valuable alongside AI's probabilistic capabilities.
The combination of human insight and AI capabilities holds the potential for groundbreaking achievements in probability theory.
Absolutely! It's a synergy between human intelligence and AI that will drive the best outcomes.
Gemini and similar systems augment human expertise rather than replace it, leading to a powerful collaboration.
Well said, Amanda, Frank, and Ethan! The collaboration between human intelligence and AI is key to unlocking new frontiers in probability theory.
I'm grateful for this discussion. It's compelling to see how Gemini can revolutionize probability theory.
Indeed, Sophie! This conversation has been enlightening. Exciting times lie ahead with Gemini.
Thank you all for your contributions to this engaging discussion. It's invigorating to see the enthusiasm for Gemini's potential.
Gemini can also be beneficial in natural language understanding within probability theory.
Absolutely, Liam! Gemini's language comprehension capabilities can improve the analysis and interpretation of probabilistic data.
I wonder if there are any potential ethical concerns when using Gemini's probabilities in decision-making.
Ethics is a crucial aspect, Ben. Transparency, accountability, and addressing biases are essential when deploying AI systems like Gemini for probability analysis.
Addressing biases and ensuring fairness in probabilistic analysis is vital to avoid unintended consequences.
Continued research and development should focus on enhancing the interpretability of Gemini's probabilistic results.
I agree, Emily. Improved interpretability enables better understanding and trust in Gemini's probability estimations.
Human experts play a crucial role in addressing ethical concerns and ensuring AI is used responsibly in probability analysis.