Unlocking Efficiency and Accuracy in Data Labeling: Harnessing ChatGPT for Enhanced Data Transformation
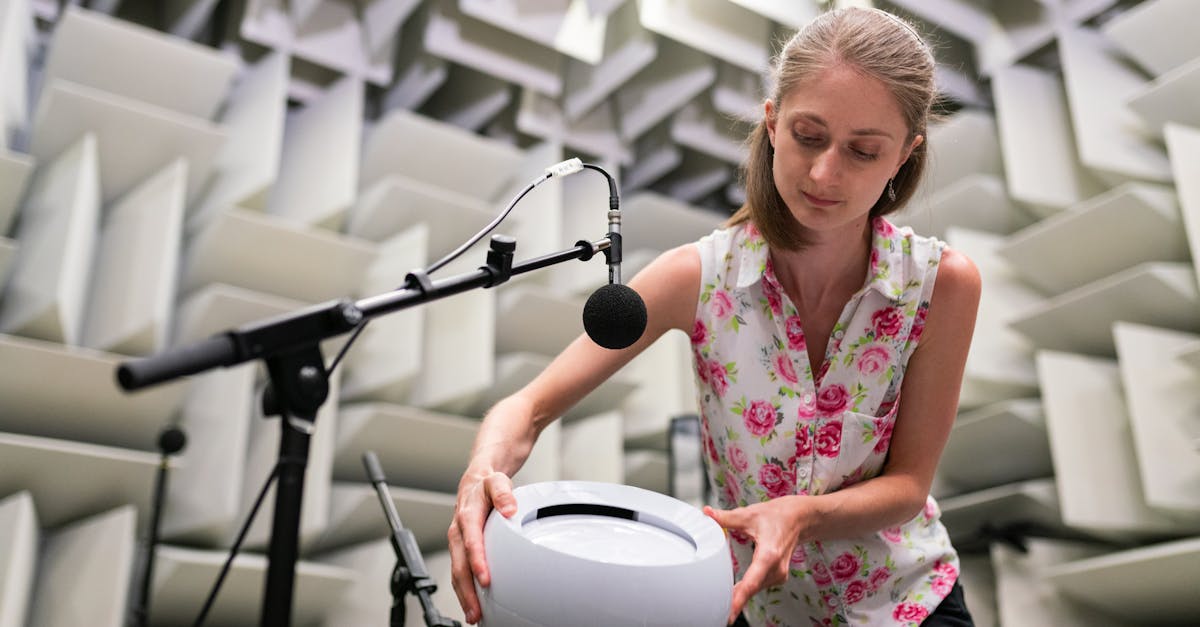
With the evolution of technology, data has become an integral part of modern businesses, making data transformation and data labeling paramount in manipulating and synthesizing raw data for the benefit of these enterprises. One of the most groundbreaking technologies in this domain is Chatgpt-4, an innovative tool that can automate the process of data labeling, which is a crucial component of most machine learning projects.
What is Data Transformation?
Data transformation refers to the set of processes that involve converting data from one form or structure into another. This process is pivotal in various tasks, such as data integration, data management, data warehousing, and data wrangling. The goal of data transformation is to improve data quality, enhance data value, and make data suitable for specific operational and business objectives.
Understanding Data Labeling
Data labeling, a subset of data pre-processing, plays a vital role in machine learning. It involves tagging or classifying the raw, unprocessed data to make it understandable for machine learning algorithms. Examples of data labeling techniques include image annotation, sentiment analysis, text categorization, etc.
The Significance of Data Labeling
In the realm of machine learning, AI, and deep learning models, clean, organized, and labeled data is the key to achieve accurate predictive models. Without proper data labeling, an ML model may struggle with identification and prediction tasks. Hence, investing time and resources into data labeling can prove to be fruitful for businesses aiming to harness the power of advanced AI and ML technologies.
How can Chatgpt-4 Automate Data Labeling?
Chatgpt-4, the latest groundbreaking technology built with OpenAI's powerful language model GPT-4, brings a revolution to the table regarding automated data labeling. This tool reads, understands, and processes data, providing labeled datasets needed for training machine learning models.
Efficiency and Accuracy
With Chatgpt-4, the data labeling process accelerates while ensuring high-quality results. It can sift through large amounts of data in real-time, accurately labeling them, improving the quality and consistency of the labeling compared to manual processes. This accuracy improves model performance and tuning, leading to improved AI and ML tools.
Cost and Time-effective
Automating data labeling reduces costs associated with manual labor and saves significant time in the process. Additionally, with the ongoing improvements in AI, the automation allowed by tools such as Chatgpt-4 becomes increasingly accurate and reliable, freeing up valuable human resources that can be redirected to higher-level tasks.
Scalable and Versatile
Chatgpt-4 can handle vast amounts of data, making it a scalable solution for businesses of all sizes. Furthermore, it's versatile enough to effectively label different data types and structures, from text and images to more complex datasets, thereby broadening its scope of application significantly.
Conclusion
Automating data labeling using Chatgpt-4 can revolutionize the way businesses deal with data, providing effective, accurate, and efficient solutions. With this technology, the potentials for machine learning and AI development are only bounded by the imagination. In an era fueled by data, solutions like these are the stepping stones towards a more streamlined, precise, and advanced technological landscape.
Comments:
Thank you all for reading my article on 'Unlocking Efficiency and Accuracy in Data Labeling: Harnessing ChatGPT for Enhanced Data Transformation'. I'm glad you found it interesting! If you have any questions or comments, feel free to ask!
Great article, Jason! I never thought of using ChatGPT for data labeling. It seems like a powerful tool. Have you personally used it in your projects?
Thank you, Emily! Yes, I have used ChatGPT for data labeling in a few projects. It significantly improved the efficiency and accuracy of the process. Highly recommend giving it a try!
Interesting concept, Jason. Can you provide some examples where ChatGPT outperforms traditional methods of data labeling?
Certainly, David! ChatGPT performs exceptionally well in scenarios where the labeling task requires context understanding, ambiguous decision-making, or subjective judgment. It effectively reduces human biases and enhances the accuracy of labeling, especially in complex datasets.
I am worried about potential biases from AI models like ChatGPT. How can we ensure unbiased data labeling?
Valid concern, Sarah. To mitigate biases, it's essential to carefully design the guidelines for the ChatGPT assistants and provide them with proper training. Continuous monitoring, feedback loops, and iterative improvement processes can help optimize the models over time. Transparency in the labeling process is also crucial for identifying and addressing biases.
This is fascinating, Jason! How does the integration between ChatGPT and data labeling tools work?
Thank you, Emma! The integration usually involves building an interface that connects ChatGPT with the data labeling tool. The assistant communicates with the labeler through this interface, receiving instructions and providing responses. It streamlines the workflow and enables efficient collaboration between AI and human labelers.
I can see how ChatGPT can improve data labeling efficiency, but does it also reduce costs?
Absolutely, Ethan! By automating certain aspects of data labeling, ChatGPT can significantly reduce costs. However, it's important to strike a balance and find the right combination of AI and human involvement to ensure high-quality and accurate labeled data.
Do you think ChatGPT can completely replace human labelers in data labeling?
Not entirely, Liam. While ChatGPT greatly improves efficiency and accuracy, some labeling tasks still require human judgment, nuance, and creativity. Human labelers bring valuable context and decision-making abilities that AI models cannot fully replicate. The best approach is a collaborative one, leveraging the strengths of both AI and humans.
How do you avoid potential errors or mistakes when using ChatGPT for data labeling?
Good question, Sophia. It's crucial to have proper validation and quality control mechanisms in place. Regular and thorough testing of the ChatGPT models, along with human review and oversight, helps identify and rectify potential errors or mistakes. Iterative training and improvement cycles also contribute to reducing and preventing such issues.
I've read about ChatGPT's limitations in producing biased outputs. How do you address this problem in data labeling?
Addressing biases is a critical aspect, Oliver. It involves careful screening and training of ChatGPT models to minimize biased outputs. Additionally, diverse input examples, feedback loops, and close collaboration with human labelers contribute to identifying and mitigating potential biases. Transparency and continuous improvement are key to ensuring fair and unbiased data labeling.
What are the potential downsides or challenges of using ChatGPT for data labeling?
Great question, Ava. One challenge is that ChatGPT can sometimes generate responses that are contextually correct but conceptually incorrect. Another challenge is the need for ongoing model monitoring and maintenance to maintain quality standards. Striking the right balance between AI and human judgment can also be a challenge. Addressing these requires continuous improvement and adaptation of the labeling process.
What impact do you think ChatGPT will have on the future of data labeling in various industries?
ChatGPT has the potential to revolutionize data labeling in multiple industries, Mia. It enables faster and more accurate labeling, leading to improved AI models and decision-making systems. From healthcare to finance, autonomous driving to customer service, ChatGPT can enhance efficiency and enable new possibilities for data-driven industries.
Are there any ethical considerations that should be kept in mind while using ChatGPT for data labeling?
Absolutely, Leo. Ethical considerations are paramount. Ensuring privacy, avoiding bias, maintaining transparency, and obtaining proper consent when using ChatGPT assistants are critical ethical aspects. Striving for fairness, accountability, and responsible AI practices should guide the utilization of ChatGPT for data labeling.
What do you think the future holds for ChatGPT and data labeling methodologies?
The future looks promising, Olivia! ChatGPT is just the beginning of advanced AI-powered data labeling methodologies. We can expect more sophisticated models, improved integration with labeling tools, and better capabilities to handle complex labeling tasks. Collaboration between AI and human experts will continue to drive innovation and redefine the field of data labeling.
Jason, do you think ChatGPT can help overcome language barriers in data labeling?
Indeed, Joshua! ChatGPT's multilingual capabilities can help bridge language barriers in data labeling. It can assist in understanding and processing labels in multiple languages, expanding the accessibility and reach of data labeling efforts. This is particularly valuable in globally distributed projects or those involving diverse linguistic requirements.
I appreciate the insights, Jason! Are there any specific industries or domains where ChatGPT shines in data labeling?
Certainly, James! ChatGPT shines in industries like e-commerce, content moderation, virtual assistants, and customer support where context understanding, conversation, and subjective labeling are important. It's also useful in domains like healthcare, autonomous vehicles, and finance, dealing with intricate decision-making processes that require nuanced understanding.
I'm curious, Jason. How do you ensure alignment between the ChatGPT assistant and human labelers in data labeling projects?
Excellent question, Chloe. Ensuring alignment requires clear guidelines, detailed documentation, and proper training programs for ChatGPT assistants. Establishing effective communication channels, feedback loops, and regular meetings also facilitate alignment between the AI and human labelers. Collaboration and a shared understanding of the labeling objectives are crucial for success.
How do you measure the accuracy and quality of ChatGPT's labeled data?
Measuring accuracy and quality involves various approaches, Aiden. It can include comparison with ground truth data, inter-rater agreements, consistency checks, or spot checks with human experts. Continuous feedback and improvement loops are essential for assessing and enhancing ChatGPT's performance, ensuring high-quality and reliable labeled data.
Do you have any tips for implementing ChatGPT for data labeling projects?
Absolutely, Hannah! Ensure clear task definitions, guidelines, and instructions for ChatGPT assistants. Iteratively refine the models based on feedback loops and constantly monitor their performance. Collaborate closely with human labelers, maintaining transparency, and addressing any concerns or questions they might have. Lastly, continuously iterate and improve the labeling process based on the insights gained.
Jason, with ChatGPT, do you think we can achieve real-time or near real-time data labeling?
Real-time or near real-time data labeling is indeed a possibility with ChatGPT, Isabella. The automation and efficient collaboration it enables can significantly reduce the time required for labeling tasks. With continuous improvements and enhancements in AI models and integration, we can expect faster turnaround times for data labeling, empowering real-time decision-making.
Jason, what are your thoughts on using ChatGPT for labeling large-scale datasets?
ChatGPT can certainly handle large-scale datasets, Sophie. Its scalability makes it suitable for labeling vast amounts of data. However, it's important to ensure proper infrastructure, monitoring, and iterative training to maintain accuracy and quality. Carefully designing labeling strategies and leveraging human expertise, alongside AI capabilities, can help tackle large-scale data labeling effectively.
Thanks for sharing your insights, Jason! I'm excited to explore the possibilities of using ChatGPT for data labeling in my projects.
You're welcome, Noah! I'm glad you found the insights useful. Feel free to reach out if you have any further questions or need assistance while working with ChatGPT for data labeling. Good luck with your projects!
This article opened my eyes to the potential of using ChatGPT for data labeling. I can see how it can greatly improve efficiency and quality. Thanks for sharing, Jason!
Thank you, Lily! I'm delighted that the article helped you recognize the benefits of ChatGPT for data labeling. If you have any specific questions or need further information, feel free to ask. Happy labeling!
I've been skeptical about AI-driven data labeling, but this article has given me a new perspective. Exciting stuff, Jason!
I'm glad to hear that, William! AI-driven data labeling can indeed be transformative when applied effectively. If you have any doubts or need assistance with implementing ChatGPT in your projects, don't hesitate to ask. Good luck and enjoy exploring the possibilities!
Interesting read, Jason! I can see how ChatGPT can bring valuable improvements to data labeling processes. Do you have any specific success stories where ChatGPT made a significant impact?
Thank you, Addison! Yes, there are several success stories where ChatGPT made a significant impact. One notable example is a content moderation task where ChatGPT effectively reduced errors and improved efficiency by generating contextually accurate labels. Another success story involves healthcare data labeling, where ChatGPT assisted in subjective judgment tasks, improving accuracy and reducing bias. These are just a couple of examples where ChatGPT has proven its value.
Jason, what are the criteria to consider when choosing ChatGPT for data labeling projects?
Great question, Henry! When choosing ChatGPT for data labeling projects, key criteria to consider include the complexity of labeling tasks, available resources, integration capabilities with existing labeling tools, multilingual requirements, and the need for human expertise. Moreover, assessing the readiness of the models, monitoring mechanisms, and ongoing support from the AI provider are also important factors to evaluate.
Thanks for sharing your expertise, Jason! This article provided valuable insights into leveraging ChatGPT for enhanced data labeling. I appreciate it!
You're welcome, Grace! I'm thrilled that the article provided valuable insights for you. If you have any further questions or need assistance in implementing ChatGPT for data labeling, feel free to ask. Best of luck in your labeling endeavors!