Unlocking Efficiency and Accuracy: Leveraging ChatGPT for Generating Metadata in Broadcast Engineering
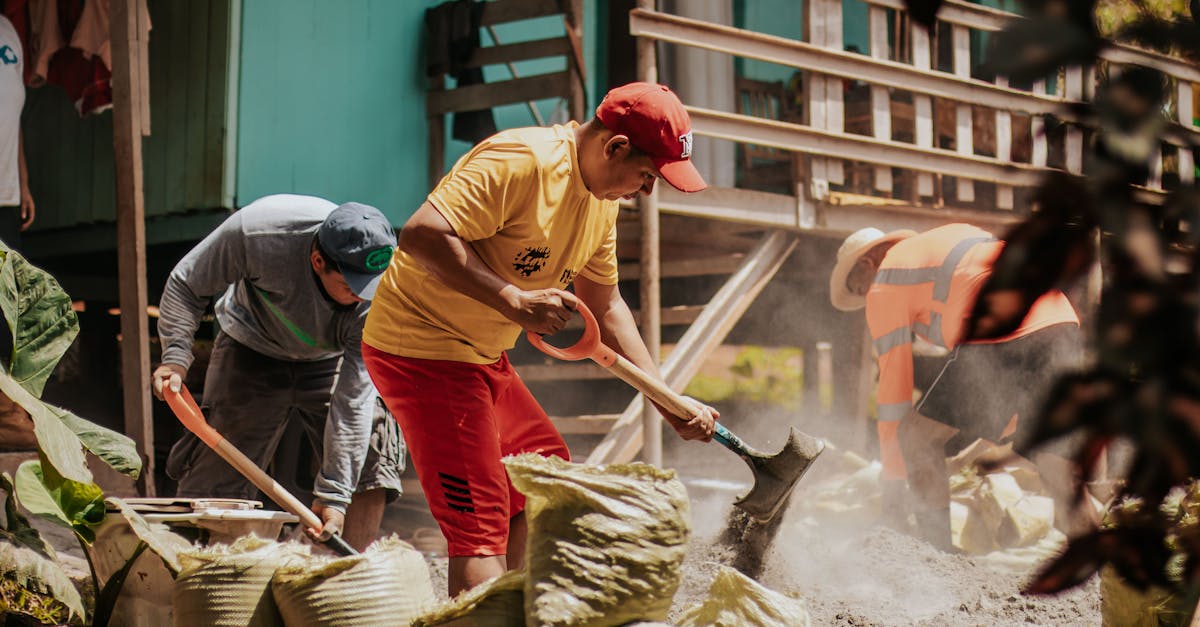
Metadata plays a crucial role in the broadcast industry, allowing broadcasters to organize and search for content efficiently. With the advancements in broadcast engineering, creating accurate and comprehensive metadata has become much easier. This article explores how broadcast engineering helps in generating metadata, ultimately enhancing searchability and organization.
What is Metadata?
Metadata refers to the descriptive information about a piece of content. In the context of broadcast engineering, metadata includes details such as title, duration, format, genre, keywords, and more. It provides vital information about the content, facilitating efficient management, distribution, and consumption.
The Role of Broadcast Engineering
Broadcast engineering involves the design, implementation, and maintenance of broadcasting equipment and systems. In the realm of metadata, broadcast engineering contributes significantly by automating the generation process through various technological advancements.
1. Automated Metadata Extraction
Modern broadcast engineering systems are equipped with automated tools that extract metadata from various sources. For example, speech-to-text technologies convert spoken words in video or audio content into textual data, which can be used as metadata. Automatic content recognition (ACR) systems help identify scenes, objects, and logos, adding descriptive metadata based on visual cues.
2. Integration with Broadcast Workflows
Efficient metadata generation requires tight integration with existing broadcast workflows. Broadcast engineers work closely with content management systems (CMS) and media asset management (MAM) systems to ensure seamless metadata extraction and association. By integrating metadata generation within the workflow, broadcasters can save time and effort, resulting in more accurate and consistent metadata.
3. AI and Machine Learning
Artificial Intelligence (AI) and machine learning algorithms have revolutionized metadata generation in the broadcast industry. With the ability to analyze vast amounts of data, AI-based systems can automatically tag content with relevant metadata, eliminating the need for manual intervention. These systems continuously learn and improve from user interactions and feedback, resulting in more accurate metadata over time.
Benefits of Metadata
Generating metadata using broadcast engineering techniques offers several benefits for broadcasters and content creators:
1. Improved Searchability
Accurate and comprehensive metadata enables broadcasters to search for content quickly and efficiently. With precise metadata tags, content can be indexed, making it easier to locate specific assets based on search queries. This makes the overall content discovery process seamless and enhances productivity.
2. Enhanced Organization
Metadata helps in organizing content effectively by sorting it into relevant categories, such as genre, format, or topic. This categorization improves the overall content management process and ensures that assets are properly organized and easily accessible when needed.
3. Personalized Content Recommendations
Metadata plays a crucial role in understanding the preferences and behavior of viewers. By analyzing metadata tags, broadcasters can provide personalized content recommendations to their audience. This helps in enhancing the viewer experience and increasing engagement.
Conclusion
As broadcast engineering continues to evolve, the generation of metadata has become an essential aspect of content management in the broadcast industry. Automation, integration, and AI-based technologies have revolutionized the metadata generation process, improving searchability and organization of broadcast content. With accurate and comprehensive metadata, broadcasters can efficiently manage their assets and provide a better viewing experience for their audience.
Comments:
Thank you all for reading my article! I'm excited to discuss the potential of leveraging ChatGPT for generating metadata in broadcast engineering. Feel free to share your thoughts and opinions.
Great article, Dan! ChatGPT seems like a powerful tool for automating metadata generation. Can you provide some examples of how it could be used in broadcast engineering?
Certainly, Alice! ChatGPT could be utilized to automatically generate metadata such as program descriptions, episode tags, closed captions, and even timecoded summaries of video content. It has the potential to significantly improve efficiency and accuracy in the broadcast industry.
I'm intrigued by the idea, but how reliable is ChatGPT in generating accurate metadata? Are there any potential limitations or challenges to consider?
That's a valid concern, Bob. While ChatGPT can be a powerful tool, it has its limitations. It may sometimes generate inaccurate or irrelevant metadata, especially with complex or specialized content. Fine-tuning and continuous improvements are necessary to address these challenges. However, the potential gains in efficiency outweigh the challenges.
I can see how ChatGPT can save time and effort for broadcasters. Does it require a large amount of training data to achieve accurate results?
Good question, Carol. Initially, ChatGPT requires a substantial amount of training data to achieve decent results. However, fine-tuning on specific domains like broadcast engineering and continuous feedback can gradually improve the accuracy. Flexibility and adaptability are inherent advantages of ChatGPT.
I'm concerned about potential biases in generated metadata. How can we ensure fairness and prevent any biases from influencing the content?
Fairness is indeed an important aspect to consider, Eva. To mitigate biases, it's crucial to carefully curate and review training data to ensure it's diverse and representative. Additionally, gathering user feedback and continuously iterating on the model's outputs can help in addressing and reducing any biases that may arise.
I'm curious, can ChatGPT assist in real-time metadata generation during live broadcasts or is it more suited for post-production tasks?
Good question, Frank. While ChatGPT can potentially assist in real-time metadata generation, it currently performs better in post-production tasks where it has more context and available data. However, with advancements in technology, real-time application scenarios can be explored and improved upon.
Are there any privacy concerns when using ChatGPT? Should we be worried about the data being processed?
Privacy is important, Grace. When using ChatGPT or any AI model, it's crucial to handle data responsibly. By implementing strong data protection measures and ensuring compliance with applicable regulations, privacy concerns can be minimized. Transparency and user consent are essential throughout the data processing pipeline.
I'm curious about the implementation process. How easy or complex is it to integrate ChatGPT into existing broadcast engineering workflows?
Integration complexities can vary, Henry. It depends on factors like the existing infrastructure, required data sources, and the level of customization needed. While it may require some initial effort, leveraging APIs and compatible tools can simplify the process. Collaborative efforts with AI experts and engineers are recommended for a smooth integration experience.
How does ChatGPT handle multilingual metadata generation? Can it support multiple languages?
Yes, Ivy! ChatGPT has the ability to handle multilingual metadata generation. By incorporating training data and fine-tuning on specific languages, it can generate accurate metadata in various languages. This opens up opportunities for global broadcasters and wider audience reach.
What are some key factors to consider when deciding to adopt ChatGPT for metadata generation? Any advice for broadcasters looking to leverage this technology?
Great question, Jack! When considering ChatGPT adoption, it's important to evaluate factors such as available training data, potential integration challenges, ongoing maintenance, and the expected return on investment. Collaborating with AI experts, conducting pilot tests, and gathering feedback from end users can help make informed decisions.
Do you foresee any potential legal or copyright issues when using ChatGPT in metadata generation?
Legal and copyright compliance is essential, Karen. Care should be taken to ensure that the generated metadata does not infringe upon any copyrights or violate content licensing agreements. Collaborating with legal experts and understanding industry guidelines can help broadcasters navigate these issues effectively.
Is ChatGPT only limited to broadcast engineering, or are there other domains where it can be applied?
While ChatGPT's applications in broadcast engineering are promising, its potential extends beyond that domain. It can be adapted for content generation, customer support, writing assistance, and more. The versatility of ChatGPT opens up numerous possibilities in various industries.
I'm concerned about potential job losses for metadata professionals if ChatGPT becomes widely adopted. How can this technology be used without causing significant job displacement?
Valid concern, Megan. While the adoption of ChatGPT may change some job roles, it can also create new opportunities. Instead of displacing professionals, this technology can assist them by streamlining repetitive tasks, enabling them to focus on higher-value activities such as quality assurance, customization, and domain-specific expertise. Embracing AI as a tool rather than treating it as a replacement is key.
What are the potential cost implications of implementing ChatGPT for metadata generation? Is it an affordable solution for broadcasters?
Cost considerations are important, Nancy. Implementing ChatGPT can have upfront and ongoing expenses involving infrastructure, fine-tuning, and maintenance. However, increased efficiency, improved accuracy, and time savings can provide a positive return on investment in the long run. Evaluating specific cost factors and conducting a cost-benefit analysis is recommended.
How does ChatGPT handle domain-specific jargon and technical terms in metadata generation? Can it grasp the intricacies of broadcast engineering language?
ChatGPT's performance with technical language has improved, Oliver, but it may still encounter challenges with specialized terms. In such cases, fine-tuning the model on domain-specific data and incorporating expert knowledge can help improve accuracy. Continuous updates and user feedback are crucial to refine the language capabilities of ChatGPT.
Are there any risks associated with using ChatGPT for metadata generation? Potential errors or issues that broadcasters need to be aware of?
While ChatGPT can be a valuable tool, Pamela, there are risks to consider. Generated metadata may sometimes contain errors, inaccuracies, or biases. It's important to validate and review the output before publishing or relying solely on it. Regular monitoring, human oversight, and continuous improvement processes are necessary to mitigate risks effectively.
Can ChatGPT learn from user corrections or feedback to improve the quality of generated metadata?
Absolutely, Quentin! User corrections and feedback play a vital role in improving the quality of metadata generated by ChatGPT. By utilizing human-in-the-loop approaches and establishing feedback loops, the model can learn from its mistakes and iteratively enhance its performance. Continuous user engagement helps drive accuracy improvements.
Can ChatGPT generate metadata across different media formats, or is it primarily focused on video content?
Great question, Rachel! While ChatGPT's capabilities can be primarily focused on video content, it is adaptable to generate metadata for various media formats. With the right training data and customizations, it can handle tasks associated with audio, images, or even text-based content. Its versatility makes it a promising tool across multiple media domains.
What are some alternatives to ChatGPT in the realm of metadata generation?
Good question, Steve! Depending on specific requirements, alternatives to ChatGPT can include rule-based approaches, keyword extraction, or even hybrid models combining AI techniques. Each approach has its own advantages and limitations. Determining the most suitable solution requires assessing factors like accuracy, efficiency, scalability, and available resources.
What are your thoughts on the future advancements of ChatGPT for metadata generation in the broadcast industry?
Exciting times lie ahead, Tina! The future advancements for ChatGPT in metadata generation include better language understanding, reduced biases, improved handling of technical terms, and enhanced domain-specific accuracy. As the model evolves and researchers continue to push its boundaries, broadcasters can expect even more efficient and accurate metadata generation.
Can ChatGPT be combined with other AI technologies to further enhance its capabilities in metadata generation?
Absolutely, Victor! Combining ChatGPT with complementary AI technologies such as object recognition, speech-to-text, or sentiment analysis can further enhance metadata generation capabilities. By leveraging multiple AI tools and techniques, broadcasters can create more comprehensive and accurate metadata for their content.