Utilizing ChatGPT for Enhanced Content Segmentation in Broadcast Engineering Technology
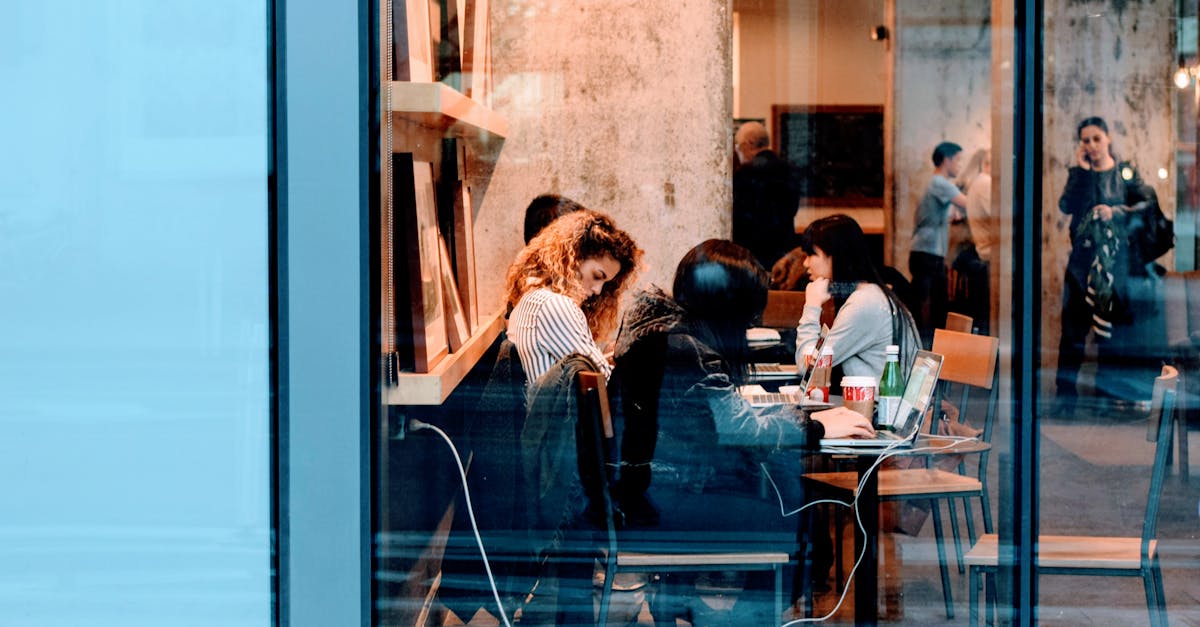
In the world of broadcast engineering, the management of content plays a crucial role in delivering high-quality programming to viewers. Traditionally, broadcast engineers manually categorize and manage different segments within a broadcast, such as news, sports, music, and more. However, with the advancement of technology, content segmentation can now be automated, saving time and effort for broadcasters.
One of the key technologies that enables this automation is machine learning. Machine learning algorithms can be trained to identify different segments within a broadcast by analyzing audio and visual data. This technology leverages artificial intelligence to recognize patterns and classify content based on predefined criteria.
The benefits of using content segmentation technology in the field of broadcast engineering are numerous. Firstly, it eliminates the need for manual intervention, reducing the chances of human error and ensuring consistent categorization. This automation streamlines the content management process, allowing broadcasters to focus on other aspects of production.
With content segmentation technology, broadcasters can also improve the viewer experience. By accurately categorizing segments, broadcasters can create personalized playlists or recommendations for viewers based on their preferences. This enhances user engagement and increases viewer satisfaction.
Moreover, automation through content segmentation technology enables broadcasters to optimize their content distribution strategies. By identifying popular segments, broadcasters can allocate resources more efficiently, creating targeted programming that appeals to their audience. This data-driven approach can lead to improved ratings and higher advertising revenues.
Content segmentation technology can also be integrated with other broadcast engineering solutions. For example, it can be combined with automated ad insertion systems, which can deliver targeted advertisements based on segment categories. This targeted advertising approach can enable broadcasters to generate additional revenue streams while providing relevant ads to their viewers.
While content segmentation technology offers numerous advantages, it is not without its challenges. Training the machine learning algorithm requires a significant amount of high-quality training data. Broadcasters must invest in data collection and annotation processes to ensure accurate categorization. Additionally, regular updates and maintenance of the algorithm are necessary to adapt to evolving trends and new content formats.
In conclusion, content segmentation technology holds great potential for automating the content management process in broadcast engineering. By leveraging machine learning algorithms, broadcasters can identify different segments within a broadcast, such as news, sports, music, and more. This automation not only improves efficiency, but also enhances the viewer experience and enables targeted content distribution strategies. While there are challenges to overcome, the benefits of content segmentation technology are undeniable, making it an invaluable tool for broadcasters in the modern age.
Comments:
Thank you all for taking the time to read my article on Utilizing ChatGPT for Enhanced Content Segmentation in Broadcast Engineering Technology. I hope you found it informative and thought-provoking. I'm looking forward to hearing your thoughts and opinions!
Great article, Dan! I found the concept of using ChatGPT for content segmentation fascinating. It has the potential to greatly improve broadcast engineering technology.
I agree, Mark. This technology opens up exciting possibilities. It can help streamline processes and improve overall efficiency in content management for broadcast engineering.
I'm not entirely convinced about the practicality of using ChatGPT in this context. Can you provide more details on how it can be implemented effectively?
Good question, David. The implementation process involves training the ChatGPT model with domain-specific data related to broadcast engineering content. Once the model is fine-tuned, it can be used to automatically segment and categorize content, making it easier to manage and retrieve relevant information.
I'm curious to know if ChatGPT can handle different languages and dialects effectively. Content segmentation can vary depending on regional variations.
That's a valid concern, Sarah. While ChatGPT performs well in English, handling other languages and dialects effectively can be challenging. However, by providing training data specific to the target languages and dialects, the model's performance can be improved.
I'm interested in understanding the potential applications of content segmentation in broadcast engineering technology. Can you provide some examples, Dan?
Sure, Lisa! Content segmentation can be used to automatically categorize and tag different types of content such as news articles, video clips, audio recordings, and more. This enables broadcasters to efficiently organize their content libraries and improve the searching and retrieval process.
Thanks for clarifying, Dan! That seems extremely useful, especially for large archives of multimedia content.
I'm concerned about potential biases in content segmentation. How can we ensure fairness and accuracy, particularly when it comes to sensitive topics?
Valid point, Adam. Bias mitigation is crucial in any AI application. By carefully curating training data and actively monitoring the model's performance, we can work towards minimizing biases. It requires an ongoing effort and continuous refinements to ensure fairness and accuracy.
I'm impressed by the potential of ChatGPT for content segmentation. However, what are the limitations or challenges we might encounter when implementing it?
Great question, Karen! Some challenges include the need for high-quality training data, potential biases, language limitations, and the model's reliance on patterns from the text it was trained on. Addressing these challenges requires careful data curation, continuous improvement, and monitoring.
I wonder if ChatGPT can handle live content and real-time segmentation requirements in the broadcast engineering field.
That's a crucial consideration, Richard. Currently, ChatGPT is more suitable for offline content segmentation due to computational constraints. Implementing it for real-time segmentation would require significant infrastructure and technical adaptations.
I think ChatGPT's potential in the broadcast engineering field is immense. It can revolutionize content management and make it more efficient. I can't wait to see it in action!
Thank you for your enthusiasm, Catherine! Indeed, the possibilities are exciting, and as the technology continues to improve, we're likely to witness its transformative impact on the broadcast engineering field.
How does ChatGPT handle ambiguous or complex content that may require context beyond the immediate segment?
Good question, Samantha. ChatGPT can struggle with complex context or ambiguity, as it primarily relies on patterns from its training data. However, by incorporating additional context and employing human oversight where needed, we can mitigate these limitations and improve accuracy.
I'm concerned about potential privacy implications when using ChatGPT for content segmentation. How can we ensure sensitive information is appropriately handled?
Valid concern, Michael. Privacy is crucial, and appropriate measures must be taken. By carefully handling and managing the training data, ensuring secure data storage, and applying anonymization techniques when necessary, we can mitigate privacy risks while utilizing ChatGPT for enhanced content segmentation.
I'm curious about the computational requirements for implementing ChatGPT. How much computing power is needed to utilize the model effectively?
Great question, Emily. Utilizing ChatGPT effectively does require significant computational resources. Training the model often entails using powerful GPUs or specialized hardware accelerators. However, once trained, the inference process can be less computationally intensive, making it more practical for deployment.
It's fascinating to see how AI is increasingly being applied in different industries. Can you elaborate on the implications of ChatGPT for the future of broadcast engineering technology?
Absolutely, Gregory! ChatGPT has the potential to revolutionize content management and enhance efficiency in the broadcast engineering field. With improved segmentation and categorization capabilities, broadcasters can streamline workflows, improve content retrieval, and even personalize content delivery, ultimately providing a better viewing experience to audiences.
I wonder if ChatGPT can be used to automate the process of generating video transcripts. It could save a lot of time and effort.
Indeed, Oliver. ChatGPT can be utilized to automate certain aspects of generating video transcripts. By transcribing spoken content and segmenting it into meaningful sentences or paragraphs, it can significantly reduce the manual effort required for transcription tasks.
That would be incredibly useful, especially for broadcasters dealing with a large volume of video content. It could speed up the process and make transcription more accessible.
However, it's important to note that while ChatGPT can automate certain aspects, proofreading and ensuring accuracy may still require human involvement to guarantee high-quality transcripts.
Can ChatGPT be applied to live captioning for broadcasts?
Live captioning is indeed a potential application, Jennifer. However, it brings real-time challenges that require fast processing and immediate response. While ChatGPT might not be the perfect fit for live captioning, advancements in AI may enable future solutions to meet those requirements.
I'm curious to know how ChatGPT handles technical jargon and specialized terminology commonly used in broadcast engineering.
Technical jargon and specialized terminology can pose challenges for ChatGPT. However, by fine-tuning the model with domain-specific training data that includes relevant jargon, we can improve its understanding and handling of such terminology in the context of broadcast engineering.
I found the article quite interesting, but are there any drawbacks or risks associated with implementing a content segmentation system based on AI?
There are indeed potential drawbacks, Sophie. Some risks include bias in the model's output, challenges in handling complex or ambiguous content, privacy concerns, and the need for ongoing human oversight. It's crucial to address these risks through rigorous data curation, transparent processes, and continuous improvement.
It's interesting to see how AI technologies like ChatGPT can enhance existing workflows and bring about new possibilities. However, it's also important to strike a balance between automation and human involvement. We don't want to completely undermine human expertise, especially in the field of broadcast engineering.
Absolutely, Peter. AI technologies should be seen as tools to augment human capabilities rather than replace them. In the broadcast engineering field, human expertise remains invaluable, and the role of AI is to assist and streamline processes, allowing professionals to focus on higher-level tasks that require human judgment and creativity.
As AI technologies become more prevalent, how can we ensure transparency and trust in the decision-making processes of AI models like ChatGPT?
Transparency and trustworthiness are crucial, Laura. To achieve this, models like ChatGPT should provide explanations for their decisions, be subject to independent audits, and involve diverse stakeholders in their development. Open dialogue and collaboration among experts can help ensure transparency and build trust in AI technologies.
I find it fascinating how AI can be applied to diverse fields. ChatGPT's potential for enhanced content segmentation in broadcast engineering is a great example. It showcases the possibilities that arise from combining AI capabilities with industry-specific needs.
Thank you, Robert. Indeed, the potential in combining AI capabilities with industry-specific needs is vast. It allows us to address unique challenges and improve efficiency in ways that were previously difficult to imagine. The application of ChatGPT in content segmentation for broadcast engineering is a testament to these possibilities.
I'm wondering if there are any current implementations or success stories of utilizing ChatGPT for content segmentation in the broadcast engineering field.
Great question, Michelle! While ChatGPT is still relatively new, there have been successful implementations and promising results in various domains. As for the broadcast engineering field, early adopters are exploring its potential, and while specific success stories may be limited, the initial feedback is promising.
What are the potential cost implications of implementing ChatGPT for content segmentation in the broadcast engineering industry?
Cost implications are an important consideration, Brandon. Implementing ChatGPT for content segmentation requires investment in computational resources, data curation, training, and ongoing maintenance. However, as AI technologies advance and become more accessible, costs are expected to decrease, making it more viable for widespread adoption.
I'm curious if ChatGPT can be used to automatically generate metadata for content in the broadcast engineering field. This could further enhance content organization and retrieval.
Absolutely, Lucy! ChatGPT can assist in automatically generating metadata for content in broadcast engineering, such as keywords, tags, or even summaries. This enriched metadata can greatly improve content organization and make it easier to find relevant information. It's an exciting prospect for the industry.
I'm curious if there are any specific use cases where ChatGPT's content segmentation capabilities have proven particularly effective?
Great question, Julia! While ChatGPT's content segmentation capabilities are still being explored in various domains, it has shown promise in applications like news article categorization, content recommendation systems, and intelligent content filtering. As the technology evolves, we can expect more targeted use cases to emerge in broadcast engineering and beyond.