Exploring the Benefits of ChatGPT for Quality Control in the Pharmaceuticals Industry
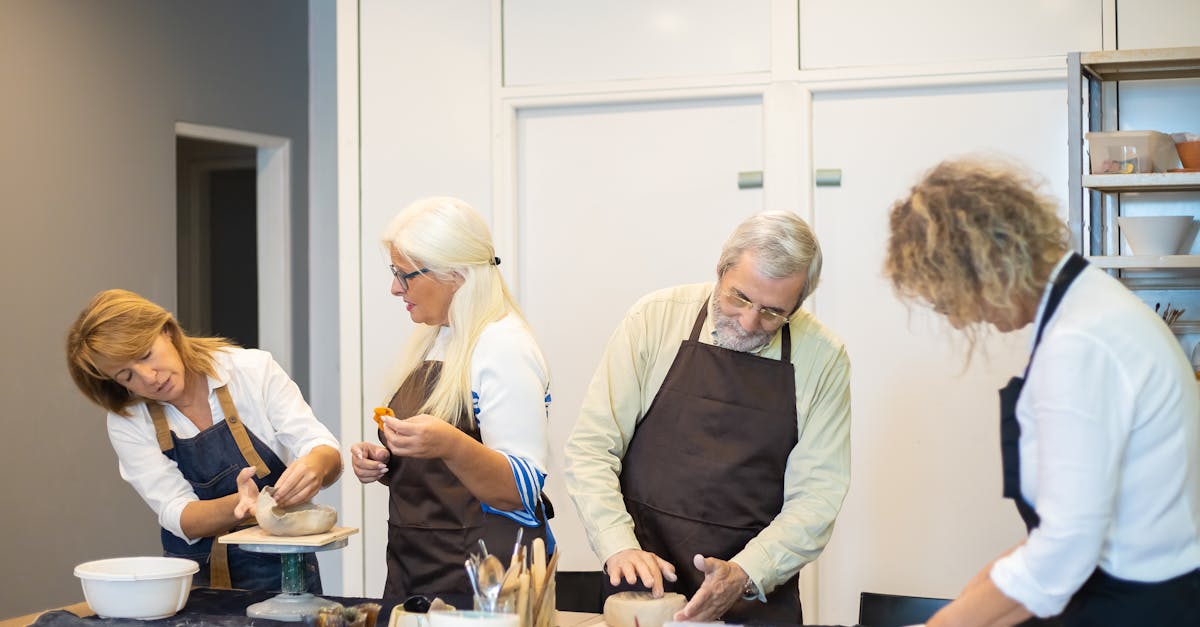
Introduction
In the field of pharmaceuticals, quality control plays a critical role in ensuring the safety and efficacy of drugs being developed. As manufacturing processes become more complex and stringent regulations are imposed, the need for advanced technologies to analyze and predict potential issues has become increasingly important.
The Role of Technology
To address this need, a new model leveraging advanced technology has been developed specifically for pharmaceutical quality control. This model utilizes machine learning algorithms to analyze large amounts of manufacturing data and predict potential issues that may arise during the production process.
How the Model Works
The model is trained using historical data from various pharmaceutical manufacturing processes. It takes into account various parameters such as temperature, pressure, humidity, and ingredient proportions, among others, to determine patterns and correlations that may indicate potential issues. By continuously learning from new data, the model becomes more accurate and efficient over time.
Benefits of the Model
Implementing this model in pharmaceutical quality control processes brings numerous benefits:
- Enhanced Efficiency: By predicting potential issues in advance, manufacturing processes can be optimized and preventive measures can be taken to minimize the occurrence of errors or deviations.
- Cost Reduction: By minimizing errors and deviations, the model helps reduce the need for rework or the disposal of faulty batches, resulting in significant cost savings.
- Improved Product Quality: Identifying potential issues allows for proactive measures to be taken, ensuring the production of high-quality drugs that meet regulatory standards.
- Enhanced Safety: By detecting potential issues that may compromise drug safety, the model helps prevent the release of harmful or defective products into the market, safeguarding public health.
Limitations and Future Developments
While the model shows promise in analyzing and predicting potential issues in pharmaceutical manufacturing processes, it is important to note its limitations. The accuracy of predictions can vary based on the availability and quality of data. Additionally, the model may not account for unforeseen circumstances or novel issues that have not been previously encountered.
Future developments in this area aim to improve the model's accuracy by incorporating real-time data monitoring and expanding the scope of parameters analyzed. Collaborations between pharmaceutical companies and technology providers will be crucial in refining the model and adapting it to different manufacturing environments.
Conclusion
The use of advanced technologies, such as machine learning, in pharmaceutical quality control has the potential to revolutionize the industry. By analyzing and predicting potential issues in manufacturing processes, this model aids in enhancing efficiency, reducing costs, improving product quality, and ensuring public safety. As technology continues to advance, the pharmaceutical industry can leverage these innovations to improve drug development and manufacturing processes.
Disclaimer: This article is for informational purposes only and should not be considered as medical or legal advice.
Comments:
This article provides a fascinating insight into the benefits of ChatGPT in the pharmaceuticals industry. It's amazing to see how AI technology is revolutionizing various sectors.
I totally agree, Alice! The potential of ChatGPT for quality control in the pharmaceuticals industry seems immense. It could greatly enhance efficiency and accuracy in drug production.
As someone working in the pharmaceuticals industry, I'm excited about the prospects of implementing ChatGPT for quality control. It could help us identify potential issues earlier and ensure better safety standards.
I have some concerns though. AI technology has its limitations, and in a critical field like pharmaceuticals, we need to be cautious. How can we ensure the reliability and integrity of ChatGPT's outputs?
Valid point, David. Quality control in pharmaceuticals requires rigorous processes. It would be interesting to know how ChatGPT's performance can be validated and if there are any fail-safes in place.
Eleanor, you raised an important question about validation. Continuous evaluation and auditing of ChatGPT's performance are key to ensure its reliability in pharmaceutical quality control.
Thank you all for your comments! David and Eleanor, you raise important concerns. When implementing ChatGPT for quality control, validation processes and fail-safes are crucial. Rigorous testing and human oversight are essential to ensure trustworthy outputs.
I have experience with using AI in quality control, and it can be challenging to strike the right balance. While AI systems can improve efficiency, they require consistent monitoring and human intervention.
That's a great point, Frank. AI systems should augment human expertise, not replace it. Continuous monitoring and manual intervention are key to maintaining the highest quality standards.
Frank, you mentioned the need for consistent monitoring. I think regulatory bodies should play a crucial role in overseeing the implementation of AI systems like ChatGPT in the pharmaceuticals industry.
Absolutely, Kevin. Regulatory bodies need to establish guidelines and standards to ensure the responsible and ethical use of AI for quality control, thereby ensuring the safety and efficacy of pharmaceutical products.
I have a question for Mark Schmitz. Are there any specific pharmaceutical companies that have already adopted ChatGPT for quality control? It would be interesting to hear about their experiences.
Good question, Hannah. While I can't disclose specific company names, I can tell you that several pharmaceutical companies are exploring the use of ChatGPT for quality control. The initial feedback has been promising, but research and development are still ongoing.
Mark Schmitz, could you provide some examples of the specific tasks that ChatGPT can assist with in pharmaceutical quality control?
Certainly, Xavier. ChatGPT can help with tasks such as verifying test results, analyzing data for anomalies, identifying potential quality issues in manufacturing processes, and providing real-time assistance in compliance with regulations.
That sounds really promising, Mark! Having an AI system like ChatGPT to assist with quality control tasks would undoubtedly be valuable. It could potentially save time and resources.
I agree, Yara. With the right implementation and training, ChatGPT could become an indispensable tool in ensuring top-notch quality control in the pharmaceuticals industry.
Indeed, Zach. The purpose is to augment human expertise and improve the overall quality control process. ChatGPT can assist but should be seen as a support system.
I'm curious about the potential risks associated with relying heavily on AI for quality control. Would it make us more vulnerable to cyberattacks or system failures?
That's a valid concern, Ian. Cybersecurity and risk mitigation will certainly be critical elements when implementing AI systems like ChatGPT. Robust security measures and backup systems should be in place.
Agreed, Jake. The pharmaceutical industry handles sensitive data, and any AI-powered system must prioritize data security and resilience. Adequate measures should be taken to prevent cyber threats.
While I understand the concerns raised, I believe that with proper oversight and risk management practices, ChatGPT can significantly contribute to quality control in pharmaceuticals. AI has the potential to outperform human capabilities.
I'd like to know more about the inputs and training data for ChatGPT. How do we ensure that it is updated with the latest pharmaceutical knowledge and regulations?
That's an important aspect, Mary. Continuous training and updating of ChatGPT with the latest information are vital to ensure accuracy and compliance. Feedback loops and regular data updates should be implemented.
In addition, Mary, Nathan mentioned feedback loops. Continuous user feedback and improvement cycles are vital for fine-tuning ChatGPT's performance in line with evolving pharmaceutical knowledge and regulations.
It's good to hear that many pharmaceutical companies are exploring ChatGPT. Collaboration and sharing of best practices among these companies could advance the field further.
I agree, Olivia. Collaboration is key to drive innovation and establish industry standards when it comes to AI in quality control. Sharing insights and experiences can benefit everyone.
Peter, you're right. Collaboration among pharmaceutical companies can also help mitigate risks, share best practices, and continuously improve AI-driven quality control techniques.
Indeed, Oscar! The exchange of knowledge and experiences can accelerate the adoption of AI technology in quality control and lead to better industry-wide standards.
What are the potential cost implications of implementing ChatGPT for quality control? Would it require significant investment and ongoing maintenance expenses?
That's a valid concern, Quentin. Implementing AI systems does require initial investment and ongoing maintenance. However, if the benefits outweigh the costs in terms of improved quality and efficiency, it can be deemed a worthwhile investment.
Additionally, as AI technology advances and becomes more widely adopted, costs typically decrease. The long-term cost-effectiveness of ChatGPT in quality control should be assessed.
I'd like to know how ChatGPT compares to traditional quality control methods in terms of accuracy and reliability. Are there any studies or research papers exploring this?
That's an interesting point, Tom. Comparative studies between ChatGPT and traditional methods will be crucial to evaluate its performance. It would be great to see more research in this area.
Indeed, Ursula. Objective evaluations and benchmarking studies can provide valuable insights into the strengths and limitations of ChatGPT in quality control.
While AI can offer great benefits, it's important we don't overlook the ethical considerations. Fairness, transparency, and accountability need to be prioritized while implementing ChatGPT for quality control.
I wonder if ChatGPT can help in pharmacovigilance and adverse event reporting. Identifying safety issues promptly is crucial in the pharmaceutical industry.
Great suggestion, Adam. Early detection of adverse events is indeed vital. ChatGPT's ability to analyze data and patterns could potentially aid in pharmacovigilance.
Absolutely, Beatrice. The analysis of data and identification of patterns is one of the strengths of ChatGPT. It can contribute to pharmacovigilance efforts in spotting potential adverse events.
While AI can bring great benefits, it's essential to strike the right balance between human judgment and automated systems. It's important to avoid over-reliance on AI technology.
It's crucial to have clear guidelines and protocols in place when implementing ChatGPT for quality control. Transparency and clear communication among teams are imperative.
Eric, you're absolutely right. The implementation of ChatGPT for quality control should be accompanied by extensive training and clear guidelines to maximize its usefulness.
I agree, Isabel. Training the workforce on how to effectively utilize ChatGPT and interpret its outputs will be key to optimizing its impact on quality control in the pharmaceutical industry.
Well said, Jack. Proper training and collaboration between humans and AI systems are essential to harness the full potential of ChatGPT for quality control purposes.
Mark Schmitz, can you elaborate on the scalability of ChatGPT? How can it handle the increasing volume of quality control tasks in a fast-paced industry like pharmaceuticals?
That's a great question, Quincy. Scalability is a significant consideration. ChatGPT should be designed to handle higher workloads, and efficient resource allocation strategies need to be in place to meet the demands.
You're right, Robert. Scalability is paramount. ChatGPT's deployment must ensure adequate computational resources, parallel processing capabilities, and response time optimization to handle the workload effectively.
To address David's concerns, I believe it's crucial to have a strong governance framework in place when utilizing AI systems such as ChatGPT. Regular audits and human oversight can help mitigate potential risks.
Continuously reviewing and updating the AI models integrated with ChatGPT is crucial. This will ensure that the system remains aligned with the latest scientific advancements and regulatory requirements.
Pharmaceutical quality control plays a critical role in ensuring patient safety. If ChatGPT proves to be reliable and effective, it could significantly enhance these efforts.
I agree, Michael. Embracing AI technology like ChatGPT can lead to more accurate and efficient identification of potential quality issues, ultimately benefiting patients and the industry as a whole.